name: oiq-mar25 class: title, middle ## Intelligence artificielle et la crise climatique ### Défis et opportunités pour l’ingénierie Alex Hernández-García (he/il/él) .turquoise[Ordre des ingénieurs du Québec · Virtuel · 20 mars 2025] .center[
    
] .center[
    
] .smaller[.footer[ Slides: [alexhernandezgarcia.github.io/slides/{{ name }}](https://alexhernandezgarcia.github.io/slides/{{ name }}) ]] .qrcode[] --- .left-column[
.center[.smaller[
Le Devoir
, 2 juillet 2024]]
.center[.smaller[
Radio Canada
, 27 janvier 2024]]
.center[.smaller[
La Presse
, 3 juin 2023]]
] .right-column[
.center[.smaller[
Science Presse
, 26 février 2024]]
.center[.smaller[
Digital HEC
, 27 mars 2024]]
] --- count: false name: title class: title, middle ## L’empreinte carbone de l’IA .center[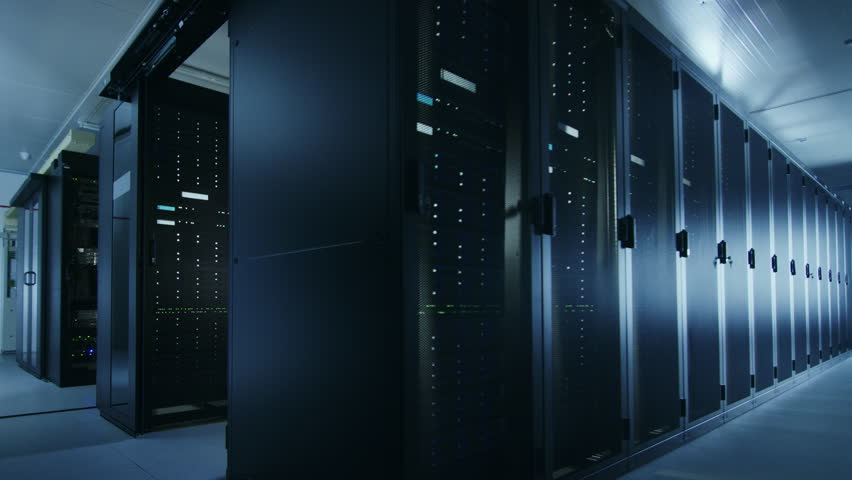] --- ## Pourquoi l’IA est-elle énergivore ? -- .highlight1[Réponse courte et simple]: Les modèles d'intelligence artificielle sont exécutés par des ordinateurs et les ordinateurs sont énergivores. -- > _Mais un ordinateur n'est pas si énergivore par rapport à d'autres choses, non ?_ -- > _Pas de tout !_ -- > _Et donc ?_ -- La question et la réponse sont plus complexes. Pour y réfléchir : - Pourquoi le transport est-il énergivore ? - Pourquoi la production alimentaire est-elle énergivore ? ??? Walking and biking is not energy-demanding, but transportation within car culture and mindless flying is. Traditional agriculture is not energy-demanding, but food production based on animal products and fertilizers is. -- .conclusion[L'intelligence artificielle n'est pas _nécessairement_ énergivore. Il existe une IA efficace et à petite échelle. Le principal **problème est l'échelle** que nous avons atteinte.] --- ## Pourquoi l’IA est-elle énergivore ? ### Une réponse plus nuancée -- .left-column[ Qu'est-ce qu'un modèle d'intelligence artificielle ? On peut considérer le pilier des modèles actuels d'intelligence artificielle comme des programmes informatiques qui transforment des données d'entrée par des opérations mathématiques pour produire des données de sortie. ] .right-column[ .center[
.smaller[
Schéma d'un réseau neuronal très simple.
]
] ] -- .right-column[ .conclusion[Chaque opération mathématique consomme en peu d'énergie. Les grands modèles d'IA effectuent une myriade de ces opérations.] ] --- ## Pourquoi l’IA est-elle énergivore ? ### Une réponse plus nuancée -- Il est important de distinguer deux phases principales dans la vie d'un modèle d'IA: .highlight1[entraînement] et .highlight1[déploiement] -- - .highlight1[Entraînement]: Il s'agit du processus d'ajustement des poids qui modulent les opérations mathématiques dans le réseau neuronal afin que le modèle exécute avec succès la tâche souhaitée. Certains modèles s'entraînent sur un ordinateur portable en quelques minutes ou quelques heures. Les modèles comme ChatGPT nécessitent plusieurs semaines et de nombreux ordinateurs puissants. -- - .highlight1[Déploiement]: Il s'agit de l'utilisation du modèle une fois qu'il a été entraîné. Certains modèles ne sont utilisés qu'avec modération. Les modèles comme ChatGPT sont utilisés par des millions d'utilisateurs chaque minute. -- .conclusion[C'est le déploiement à grande échelle de très grands modèles qui pose problème dans un contexte de crise climatique.] ??? Talk about scaling is all you need and this philosophy promoted from the industry as part of a capitalist mindset. --- ## Estimation des émissions de carbone de l'IA De quoi dépendent la consommation d'énergie et les émissions de GES d'un modèle IA ? 1. .highlight1[Temps des entraînements], $T$: somme totale du temps d'utilisation des machines de calcul (heures). 2. .highlight1[Puissance électrique], $P$, des machines de calcul (watts). 3. .highlight1[Facteur d'émission], $I$: ratio entre la quantité de gaz à effet de serre émis par quantité d'électricité produite par la source d'énergie (grammes de dioxyde de carbone par kilowatt-heure). .references[ Luccioni and Hernandez-Garcia. [Counting Carbon: A Survey of Factors Influencing the Emissions of Machine Learning](https://arxiv.org/abs/2302.08476). arXiv 2302.08476, 2023. ] -- La quantité de CO2 équivalent [CO2eq] émise lors de l'entraînement d'un modèle, C: $$C = T \times P \times I = E \times I$$ -- .conclusion[Il est assez simple d'obtenir une estimation approximative, mais **il est vraiment difficile de calculer exactement** l'énergie due à des processus spécifiques.] --- ## Estimation des émissions ## de carbone de l'IA .context[Les facteurs principaux sont les temps d'entraînement, la puissance électrique et le facteur d'émission.] En 2022, avec Sasha Luccioni, nous avons réalisé une analyse des émissions de 95 modèles d'apprentissage automatique, en interrogeant les auteurs sur les détails de leur entraînement. .center[] .references[ Luccioni and Hernandez-Garcia. [Counting Carbon: A Survey of Factors Influencing the Emissions of Machine Learning](https://arxiv.org/abs/2302.08476). arXiv 2302.08476, 2023. ] ?? Talk about lack of transparency and difficulty to obtain data. --- count: false ## Estimation des émissions ## de carbone de l'IA .context[Les facteurs principaux sont les temps d'entraînement, la puissance électrique et le facteur d'émission.] En 2022, avec Sasha Luccioni, nous avons réalisé une analyse des émissions de 95 modèles d'apprentissage automatique, en interrogeant les auteurs sur les détails de leur entraînement. .center[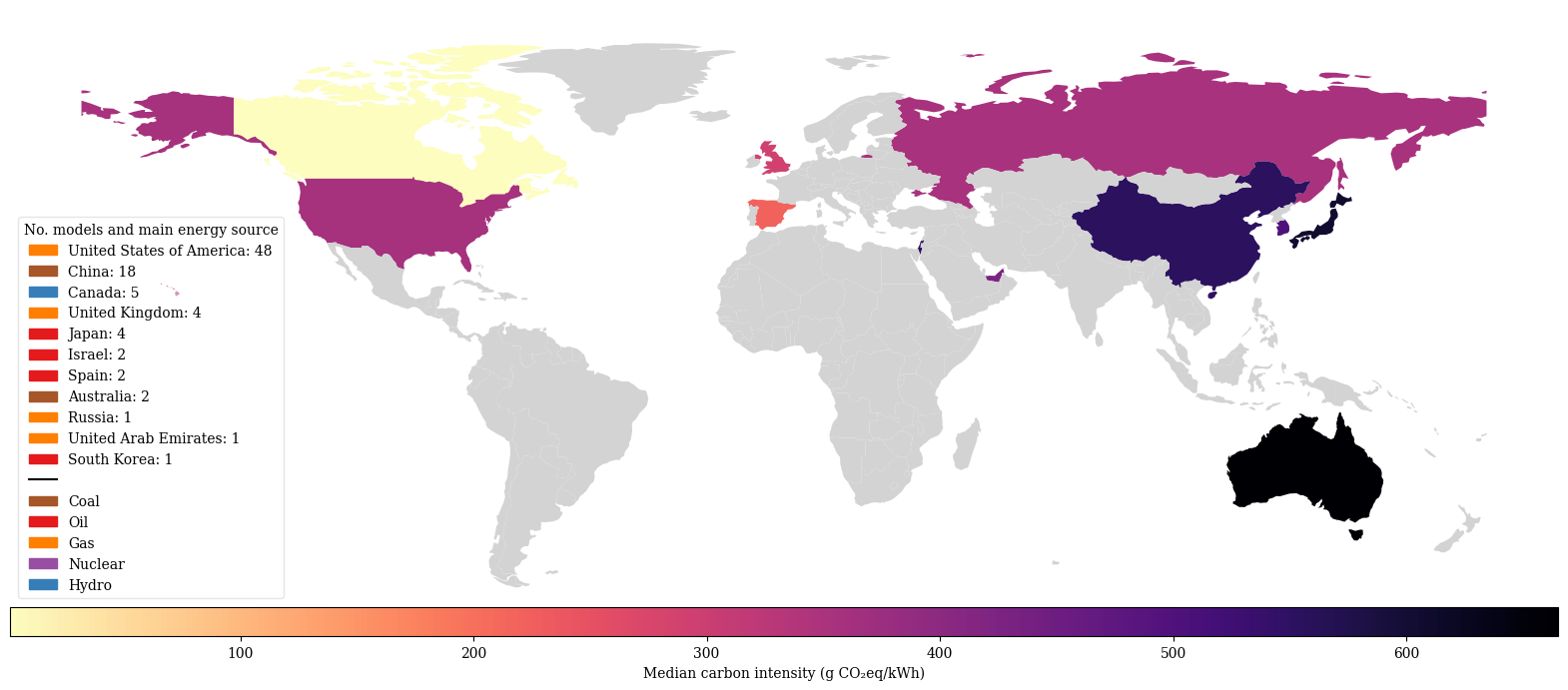] .references[ Luccioni and Hernandez-Garcia. [Counting Carbon: A Survey of Factors Influencing the Emissions of Machine Learning](https://arxiv.org/abs/2302.08476). arXiv 2302.08476, 2023. ] --- count: false ## Estimation des émissions ## de carbone de l'IA .context[Les facteurs principaux sont les temps d'entraînement, la puissance électrique et le facteur d'émission.] En 2022, avec Sasha Luccioni, nous avons réalisé une analyse des émissions de 95 modèles d'apprentissage automatique, en interrogeant les auteurs sur les détails de leur entraînement. .center[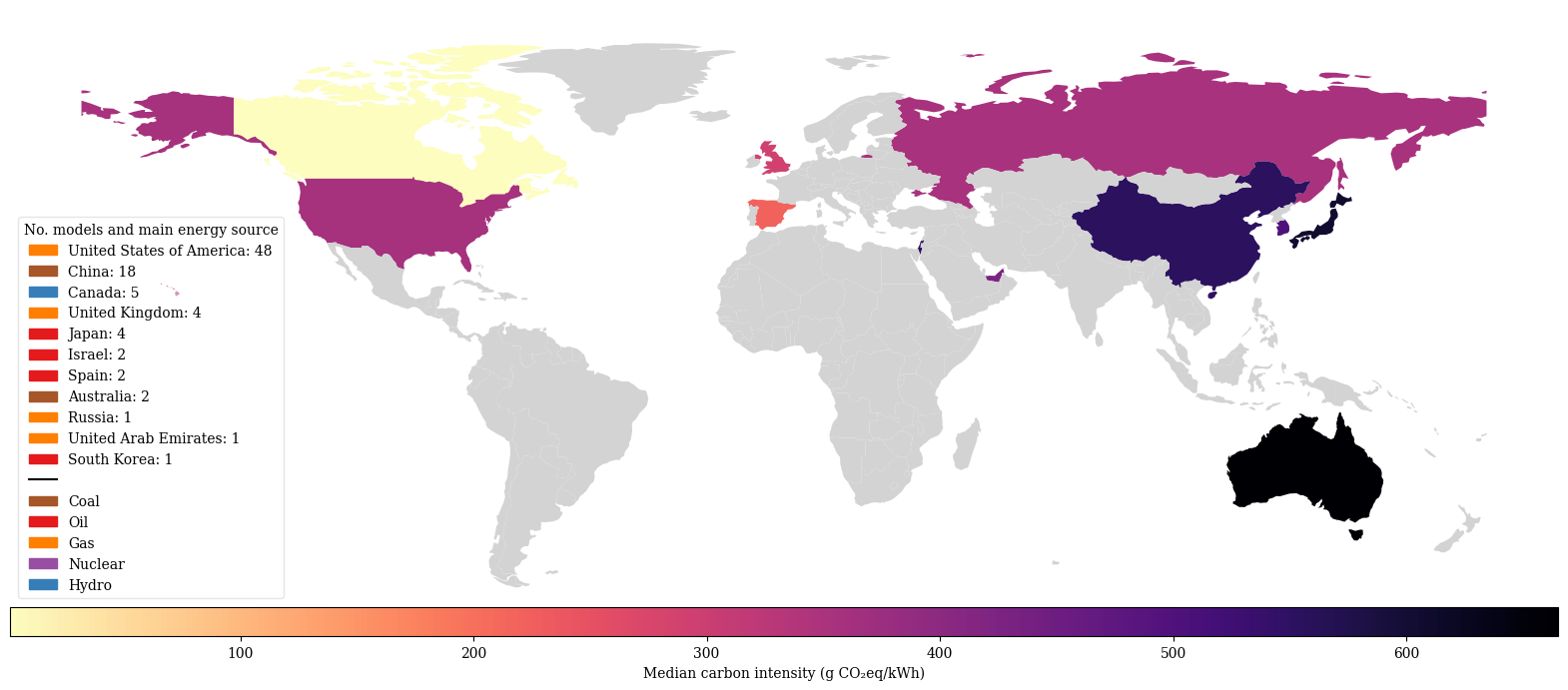] .conclusion[Il existe de grandes différences dans le [facteur d'émission du réseau énergétique](https://ourworldindata.org/grapher/carbon-intensity-electricity). De ~10 gCO2eq/kWh dans le cas de l'énergie hydroélectrique à près de 700 gCO2eq/kWh pour le charbon.] --- ## Estimation des émissions ## de carbone de l'IA ### Puissance électrique .context[Les facteurs principaux sont les temps d'entraînement, la puissance électrique et le facteur d'émission.] .center[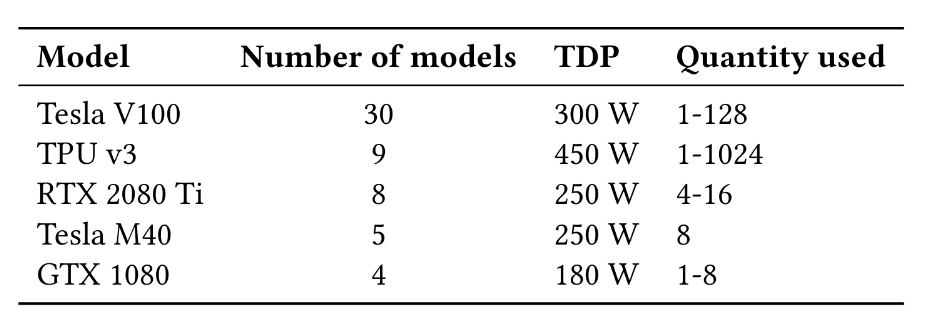] .conclusion[Par contre, il n'existe de grandes différences de puissance électrique dans le hardware utilisé.] --- ## Estimation des émissions ## de carbone de l'IA .context[Les facteurs principaux sont les temps d'entraînement, la puissance électrique et le facteur d'émission.] Le facteur d'émission depends du réseau énergétique et la puissance électrique est similaire pour les différentes options de hardware. .highlight1[Le facteur définitif restant est le temps d'entraînement]. .center[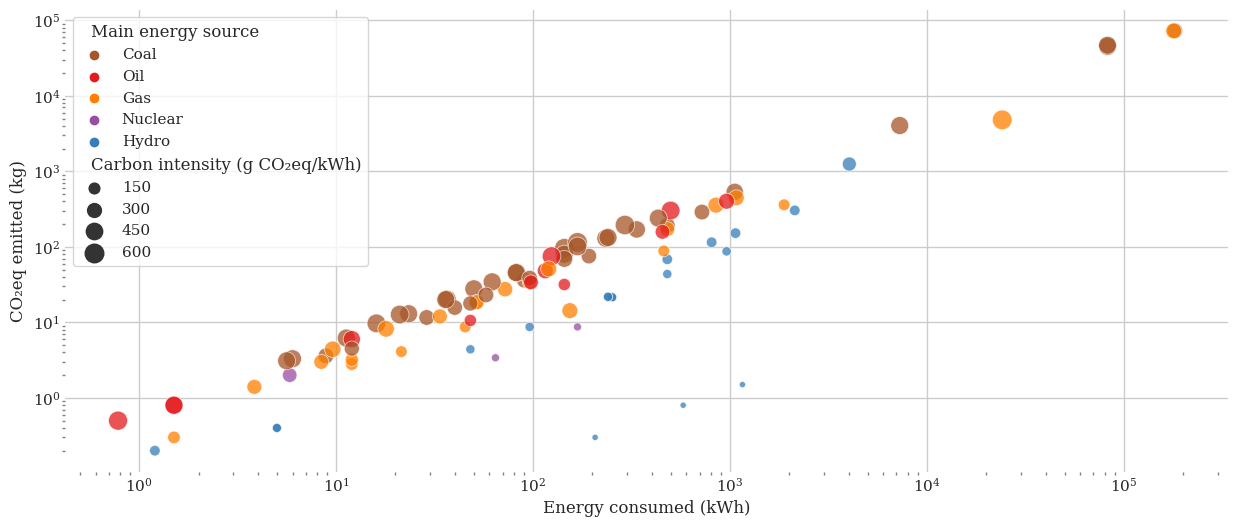] -- .conclusion[Il existe de grandes différences de temps d'entraînement et donc des émissions de CO2eq.] ??? Charging an average smartphone uses about 22 Wh. In terms of training time, the models in our sample range from just about 15 minutes (total GPU/TPU time) up to more than 400,000 hours, with a median of 72 hours, pointing again to large variance in our sample. While the maximum of of 400,000 GPU hours (equivalent to about 170 days with 100 GPUs) in our sample seems very large, note that the total training time of GPT-3 was estimated to be over 3.5 million hours (14.8 days with 10,000 GPUs). The total carbon emissions of the models analyzed in our study is about 253 tons of CO2eq, which is equivalent to about 100 flights from London to San Francisco or from Nairobi to Beijing. --- ## Estimation de la consommation d'énergie de l'IA ### Comparison des modèles IA Le modèle .highlight1[le plus léger] a été entraîné en .highlight1[15 minutes], tandis que l'un des modèles a nécessité 400 000 heures. -- Le .highlight1[total des émissions] de carbone des modèles analysés dans notre étude (95) est d'environ .highlight1[253 tonnes de CO2eq], ce qui correspond à .highlight1[environ 100 vols] de Londres à San Francisco. -- .highlight1[GPT-3], le prédécesseur de ChatGPT, a nécessité .highlight1[3,5 millions d'heures] d'entraînement (14,8 jours avec 10 000 GPU) et .highlight1[500 toones de CO2eq], ce qui équivaut à .highlight1[450 vols] transatlantiques. --- ## Comparison des modèles IA .context[Est-ce que plus d'énergie et de CO2 conduisent à une meilleure performance du modèle ?]
.center[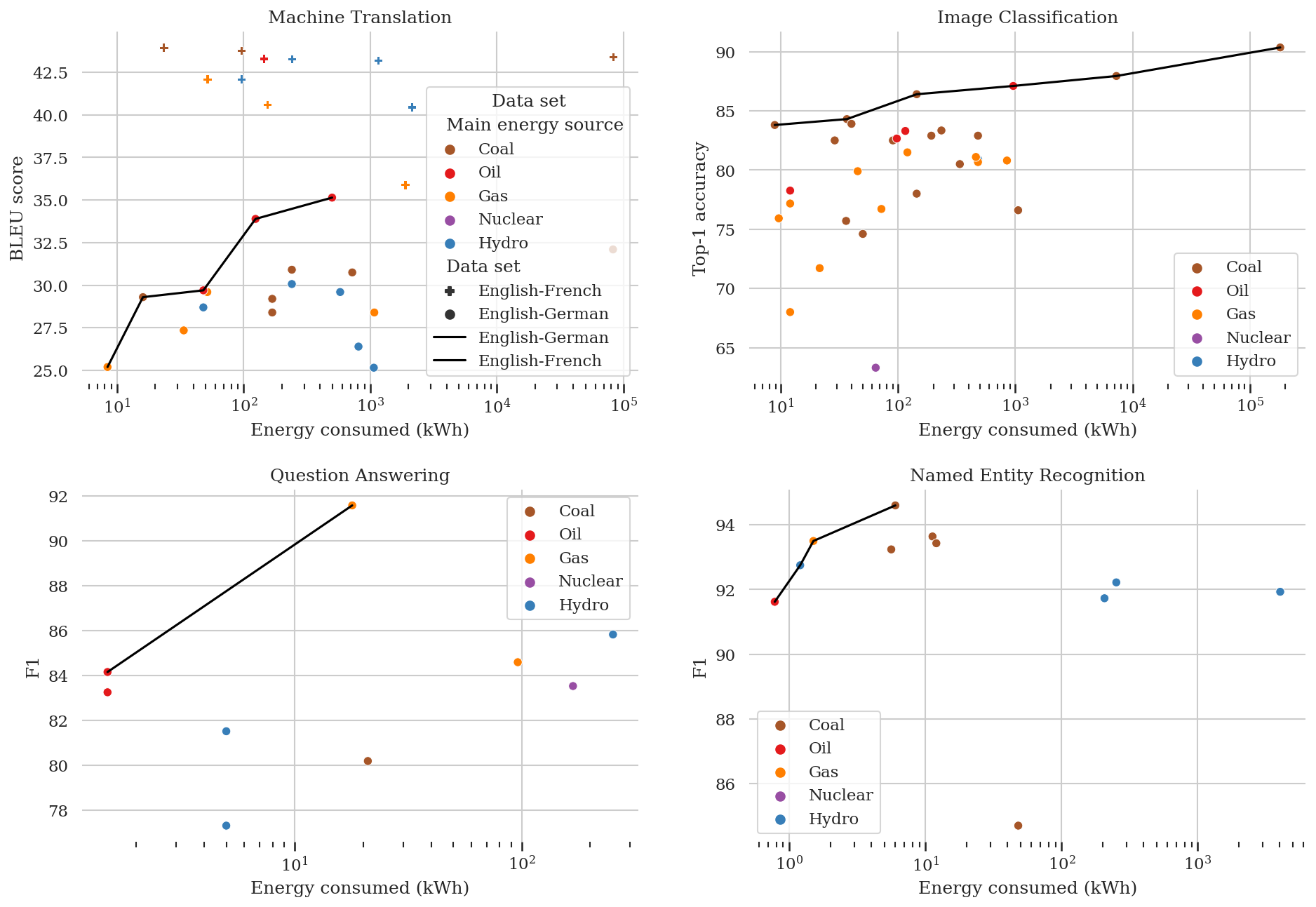] --- count: false ## Comparison des modèles IA .context[Est-ce que plus d'énergie et de CO2 conduisent à une meilleure performance du modèle ?]
.center[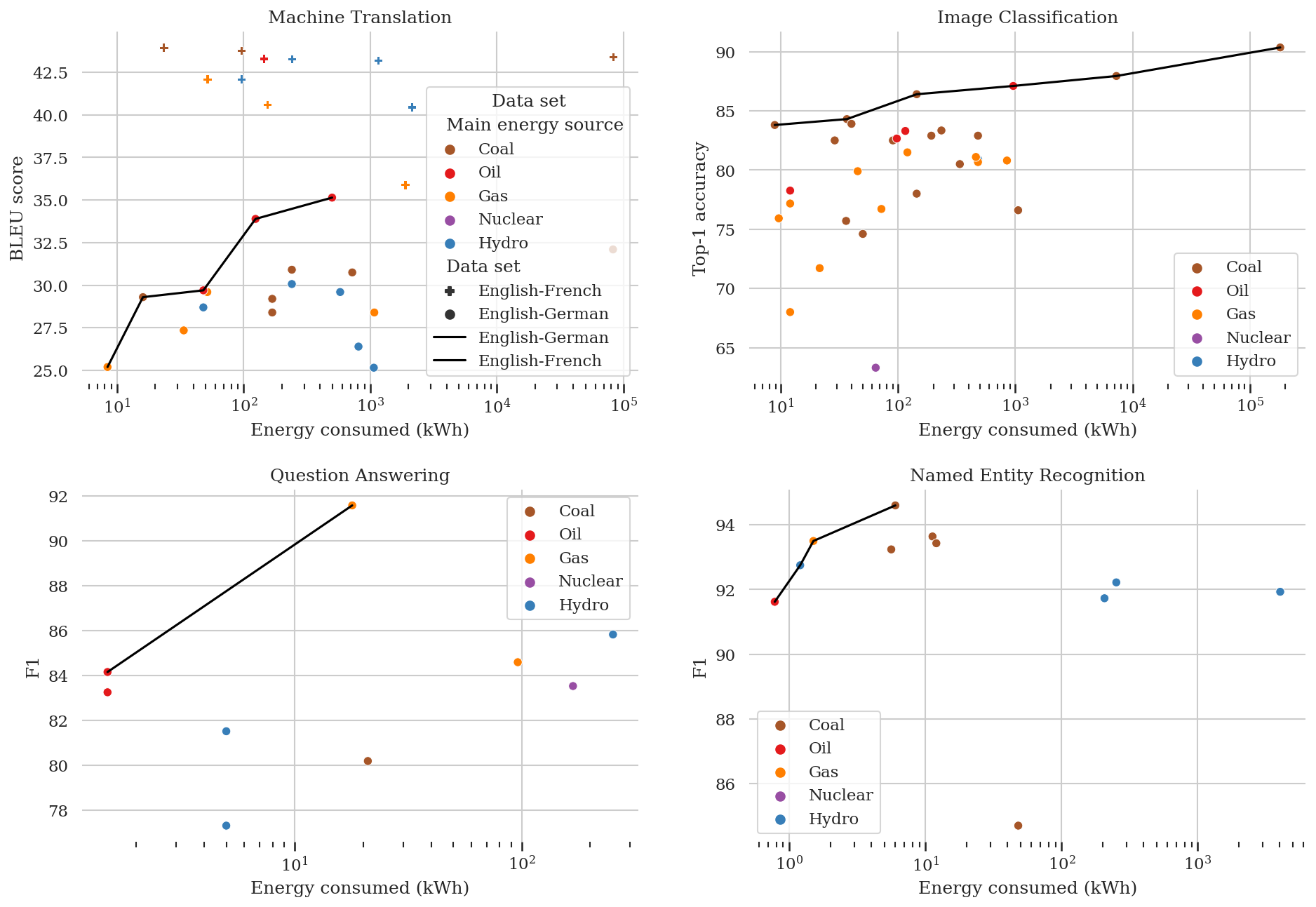] .conclusion[L'une des conclusions de notre étude est qu'il n'existe qu'une faible corrélation entre la consommation d'énergie et la performance. _Plus gros n'est pas mieux_.] --- ## Comparison des modèles IA ### Que dire des grands modèles de langage modernes ? Tout d'abord, les grandes entreprises ne fournissent pratiquement aucune information sur les besoins énergétiques de leurs modèles. Grâce au travail des chercheuses et chercheurs, nous en savons de plus en plus. -- - Les émissions de carbone de l'entraînement de BLOOM ont été estimées à 25 tonnes de CO2eq. .cite[(Luccioni et al., 2022)] - Au plus fort de la popularité de ChatGPT en 2023, l'application consommait environ 564 mégawattheures d'électricité par jour, équivalent à la consommation quotidienne d'énergie d'environ 19 000 familles des États Unis. .cite[(de Vries, 2023)] .references[ - Luccioni, Viguier, Ligozat. [Estimating the Carbon Footprint of BLOOM, a 176B Parameter Language Model](https://arxiv.org/abs/2211.02001). arXiv 2211.02001, 2022. - de Vries. [The growing energy footprint of artificial intelligence](https://www.cell.com/action/showPdf?pii=S2542435123003653). CellPress, 2023. - Luccioni, Jernite, Strubell. [Power Hungry Processing: Watts Driving the Cost of AI Deployment?](https://arxiv.org/abs/2311.16863). arXiv 2311.16863, 2023. - [AI Energy Score](https://huggingface.co/spaces/AIEnergyScore/Leaderboard) ] ??? 25 tons of CO2eq are equivalent to 180,000 km en voiture. 25 tons of CO2eq are equivalent to 40 short-haul flights. https://www.openco2.net/en/co2-converter --- ## Comparison des modèles IA ### Que dire des grands modèles de langage modernes ? .center[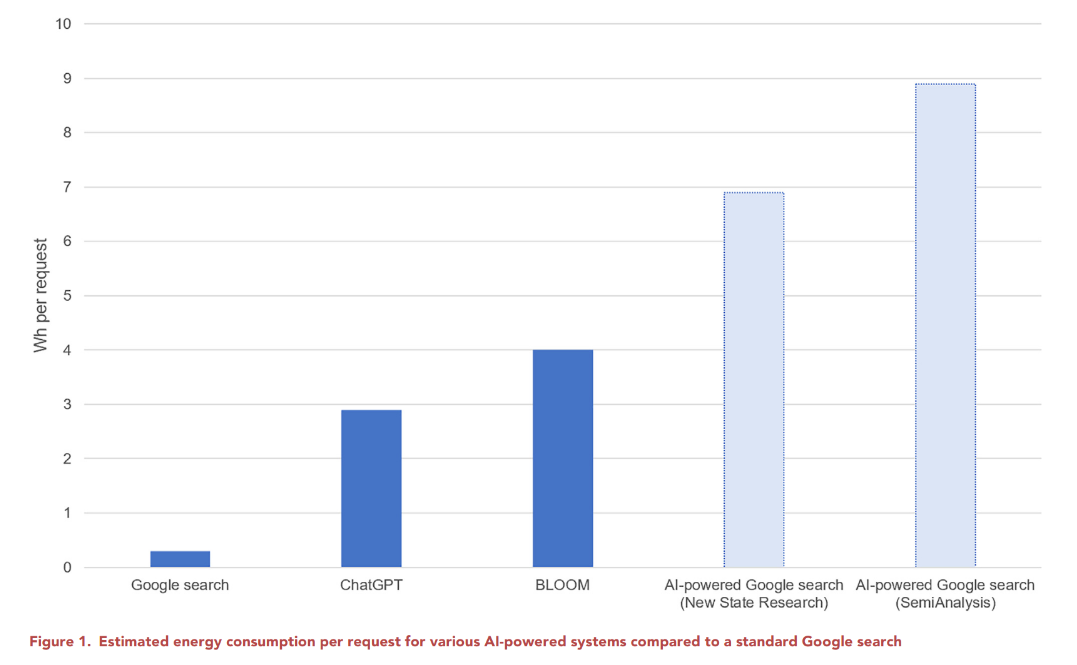] .references[ de Vries. [The growing energy footprint of artificial intelligence](https://www.cell.com/action/showPdf?pii=S2542435123003653). CellPress, 2023. ] --- count: false ## Comparison des modèles IA ### Que dire des grands modèles de langage modernes ? .center[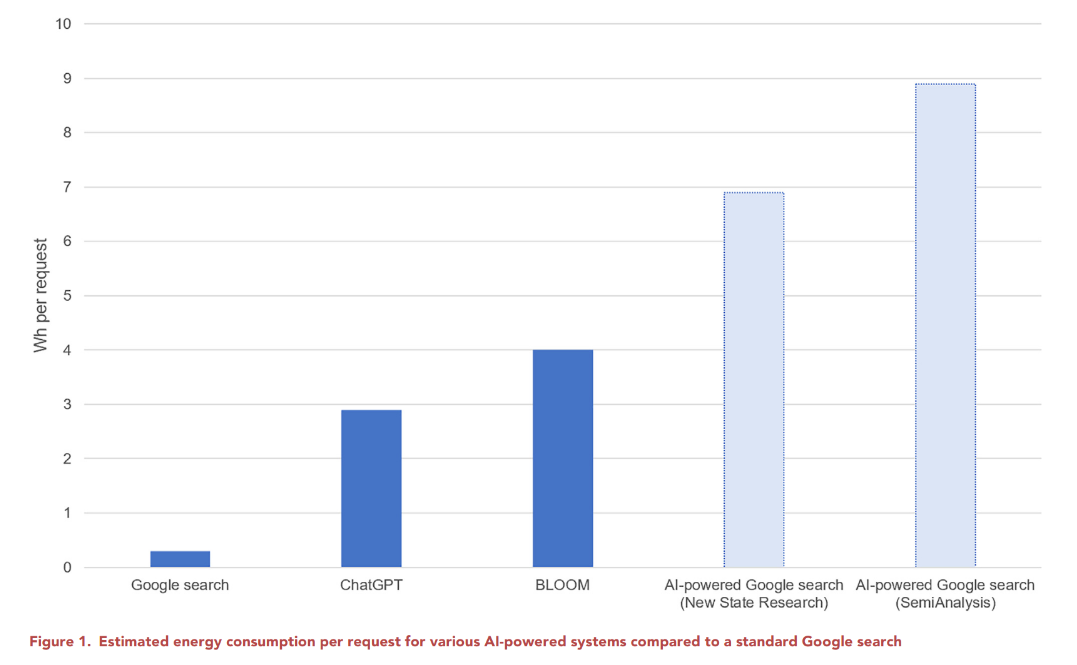] .conclusion[Une interaction avec ChatGPT pourrait consommer 10 fois plus d'énergie qu'une recherche Google.] ??? Charging an average smartphone uses about 22 W. --- ## Comparison des modèles IA ### Que dire des grands modèles de langage modernes ? .center[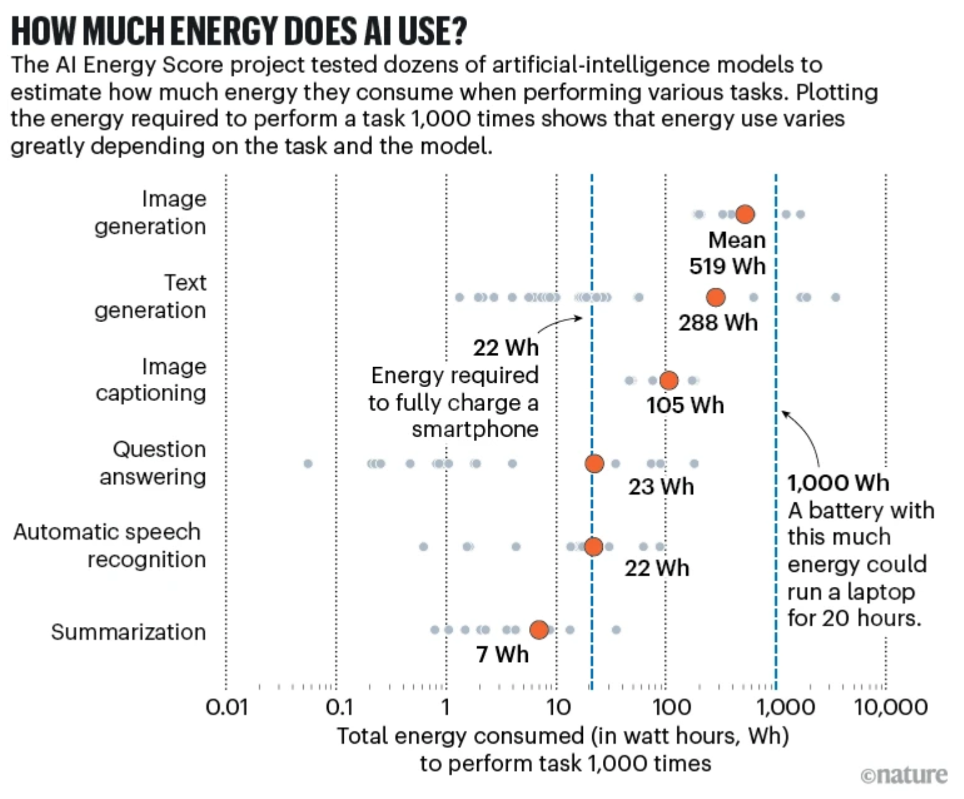] .references[ - [AI Energy Score](https://huggingface.co/spaces/AIEnergyScore/Leaderboard) - Chen. [How much energy will AI really consume? The good, the bad and the unknown](https://www.nature.com/articles/d41586-025-00616-z). Nature, News Feature, 2025. ] --- ## Demande d'énergie des centres de données ### Estimations actuelles et projections future .center[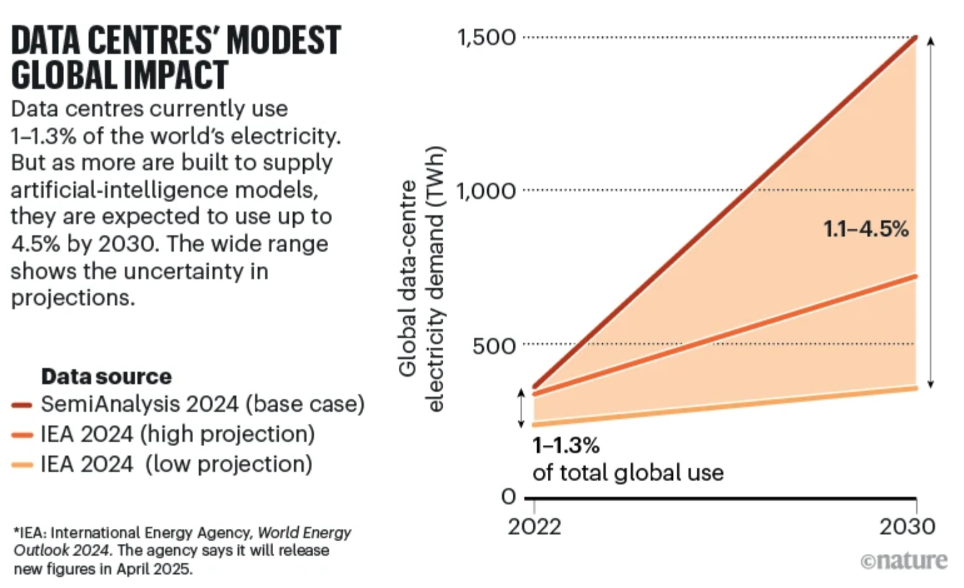] .references[ - Chen. [How much energy will AI really consume? The good, the bad and the unknown](https://www.nature.com/articles/d41586-025-00616-z). Nature, News Feature, 2025. ] --- ## Autres impacts de l'IA .context[Les grands modèles d'IA déployés à grande échelle demandent beaucoup d'énergie et émettent donc des GES.]
Outre l'énergie, les centres de données et donc l'IA consomment de .highlight1[grandes quantités d'eau potable] et exigent des .highlight1[matériaux rares]. De plus, il ne faut pas oublier l'impact social, comme les .highlight1[mauvaises conditions de travail] et l'.highlight1[accroissement des inégalités]. .center[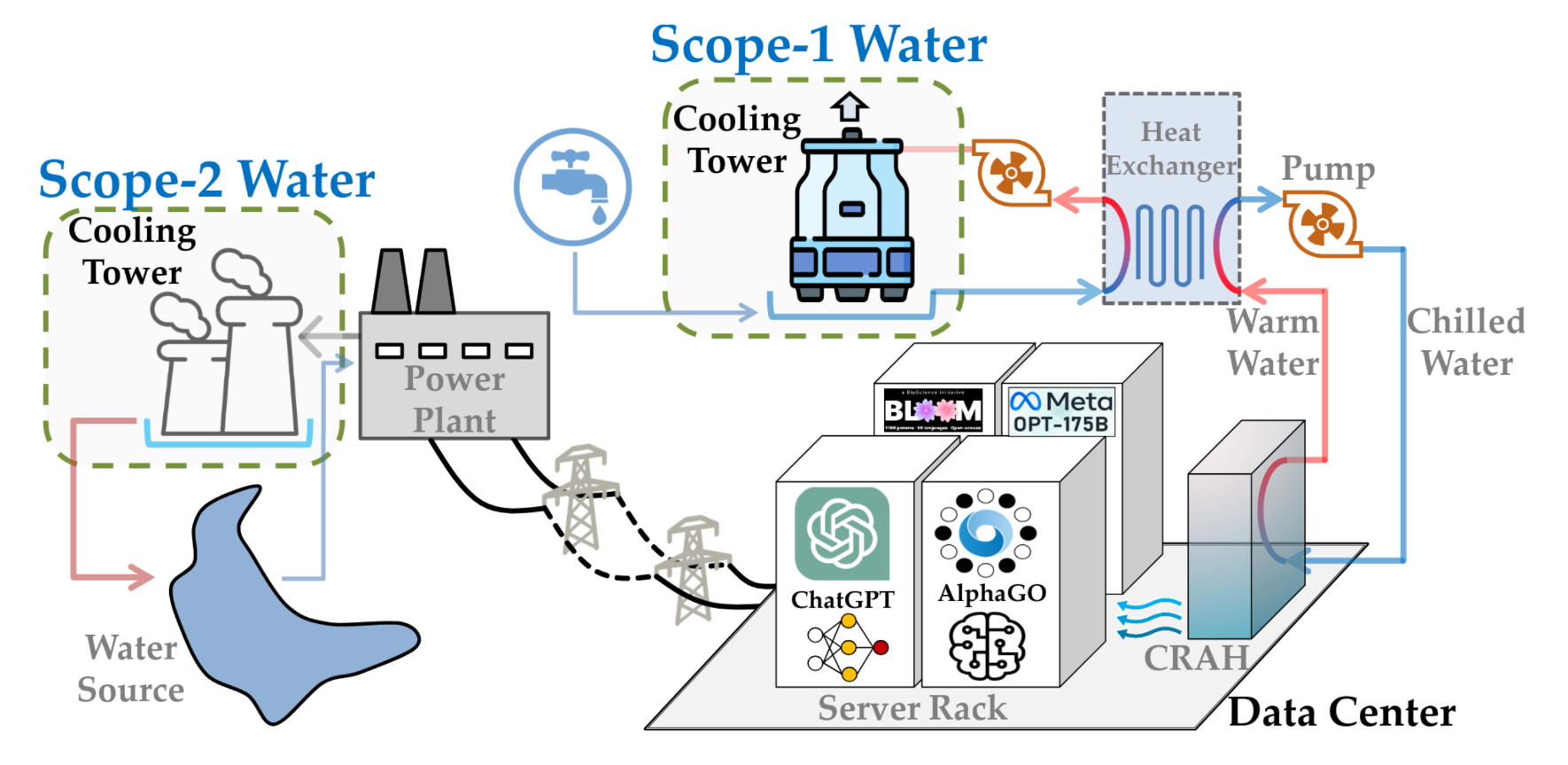] .references[ - Li et al. [Making AI Less "Thirsty": Uncovering and Addressing the Secret Water Footprint of AI Models](https://arxiv.org/abs/2304.03271). arXiv 2304.03271, 2023. - Crawford. [Atlas of AI](https://en.wikipedia.org/wiki/Atlas_of_AI), 2021 ] --- ## Initiatives pour réduire l'impact - Sensibilisation aux impacts environnementaux - Transparence améliorée des impacts sur l'environnement - Développement de modèles plus efficaces - Privilégier les modèles plus efficaces - Utilisation modérée, proportionnée et consciente des outils d'intelligence artificielle --- ## Sur l'utilisation consciente de l'IA .context35[Récemment, l'IA a été déployée dans un grand nombre de nos outils quotidiens.]
Copilot est un exemple d'intégration de l'IA dans les outils professionnels. Des chercheurs en France ont étudié son impact énergétique. .center[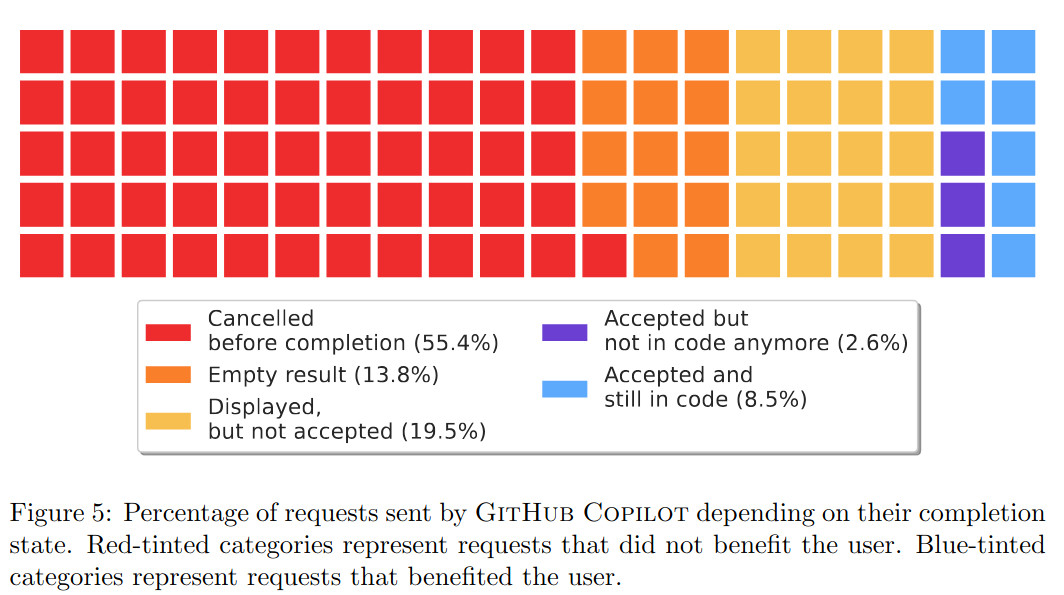] .references[ Coignion, Quinton, Rouvoy. [Green My LLM: Studying the key factors affecting the energy consumption of code assistants](https://arxiv.org/abs/2411.11892), arXiv 2411.11892, 2024. ] --- count: false ## Sur l'utilisation consciente de l'IA .context35[Récemment, l'IA a été déployée dans un grand nombre de nos outils quotidiens.]
Copilot est un exemple d'intégration de l'IA dans les outils professionnels. Des chercheurs en France ont étudié son impact énergétique. .center[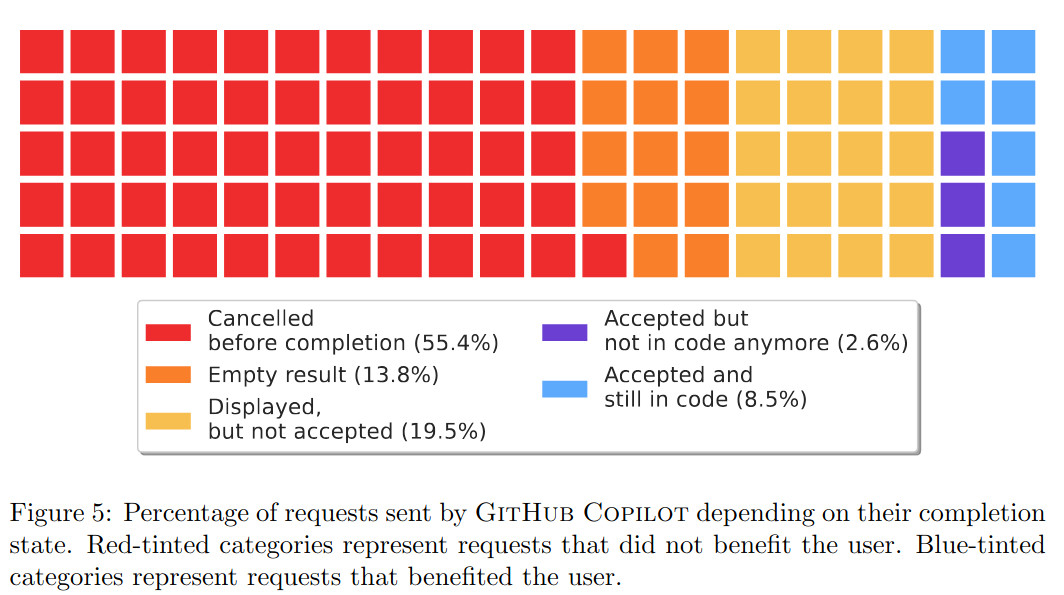] .conclusion[La plupart des résultats de Copilot sont simplement gaspillés.] --- count: false name: title class: title, middle ## Le rôle des ingénieur·e·s dans la conception responsable des IA .center[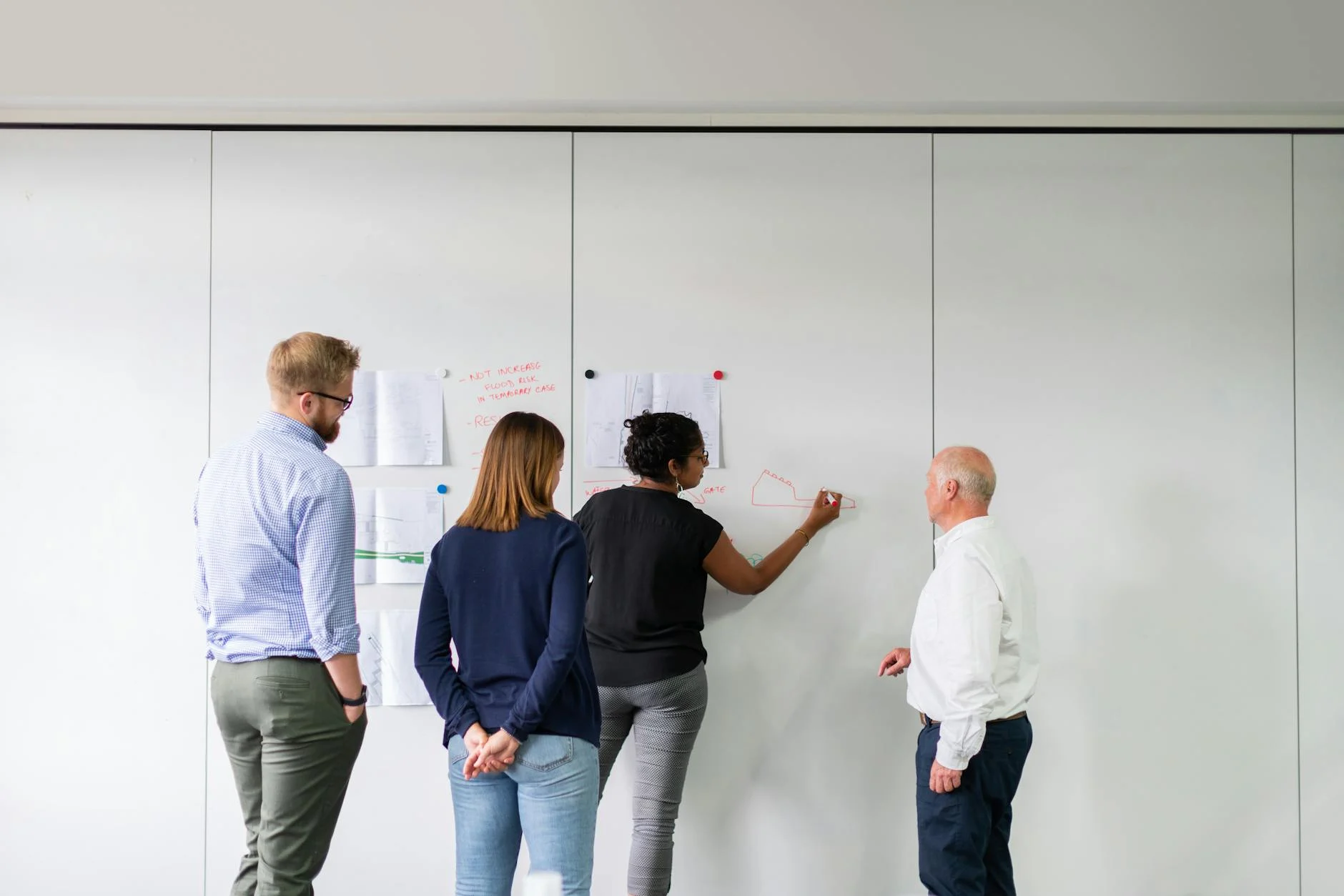] --- ## Le rôle des ingénieur·e·s dans la conception responsable des IA En tant qu'ingénieurs, nous sommes en mesure de concevoir : - des algorithmes d'intelligence artificielle - des systèmes ou des infrastructures qui utilisent l'intelligence artificielle - des systèmes ou des infrastructures pour soutenir le développement de l'intelligence artificielle -- .center[.bigger[.highlight1[Sommes-nous responsables des dommages humains et environnementaux causés par l'intelligence artificielle ?]]] -- Cela pose d'autres questions : - Qu'entendons-nous par responsable ? - Comment mesurer et attribuer la responsabilité ? - Quels sont les positionnements qui s'offrent aux ingénieurs lorsque nous prenons conscience de nos rôles ? --- ## _The implicated subject_ .left-column-66[Le livre _The Implicated Subject_ (Le sujet impliqué), de Michael Rothberg développe un cadre et un vocabulaire pour analyser la complexité de la responsabilité.] .right-column-33[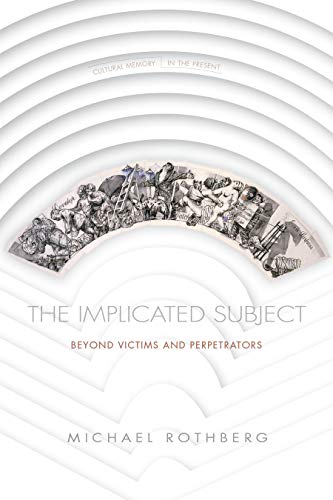] -- .left-column-66[ > .highlight1[_An implicated subject is neither a victim nor a perpetrator], but rather a participant in histories and social formations that generate the positions of victim and perpetrator, and yet in which most people do not occupy such clear-cut roles. Less “actively” involved than perpetrators, implicated subjects do not fit the mold of the “passive” bystander, either._ ] -- .left-column-66[ > _Although indirect or belated, [implicated subjects'] .highlight1[actions and inactions help produce and reproduce the positions of victims and perpetrators]._ ] --- ## Les ingénieurs en tant que sujets impliqués ### Que peut-on faire ? À mon avis, se considérer comme un _sujet impliqué_ est utile pour .highlight1[développer des plans d'action] et se permettre de .highlight1[moduler son implication]. -- Mon attitude personnelle : 1. Mieux comprendre les impacts non seulement directs mais aussi indirects de notre travail. -- 2. Développer des stratégies pour atténuer mon propre impact. -- 3. Considérer toujours le déclin comme une attitude valable. -- 4. Développer des stratégies pour atténuer l'impact systémique. -- .conclusion[L'implication implique la responsabilité mais aussi la possibilité de changer la situation.] --- ## Pour en savoir plus .columns-4[.center[
.smaller[[The Implicated Subject, Michael Rothberg (2019)](https://www.sup.org/books/literary-studies-and-literature/implicated-subject)]
]] .columns-4[.center[
.smaller[[Science as Social Knowledge, Helen Longino (1990)](https://www.jstor.org/stable/j.ctvx5wbfz)]
]] .columns-4[.center[
.smaller[[Atlas of AI, Kate Crawford (2021)](https://en.wikipedia.org/wiki/Atlas_of_AI)]
]] .columns-4[.center[
.smaller[[Race After Technology, Ruha Benjamin (2019)](https://en.wikipedia.org/wiki/Race_After_Technology)]
]] .references[ - Fernandes, Sano-Franchini and McIntyre. [What Is GenAI Refusal?](https://refusinggenai.wordpress.com/what-is-refusal/). 2024. - Abramovich and Ma. [Evaluating Refusal](https://evaleval.github.io/accepted_papers/EvalEval_24_Abramovich.pdf). 2024. ] --- count: false name: title class: title, middle ## Le rôle de l'IA dans le développement durable .center[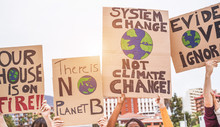] ??? More positive note --- ## Panorama des applications de l'IA pour l'ingénierie durable .highlight1[L'intelligence artificielle] ne doit pas être considérée comme la principale solution à la crise climatique, mais elle .highlight1[peut être utilisée dans de nombreux domaines] liés à l'.highlight1[atténuation] du changement climatique et à l'.highglight1[adaptation] à celui-ci. - Optimisation énergétique des bâtiments et infrastructures. - Optimisation de la production et de la distribution d'énergie - Prédiction et gestion des ressources naturelles. - Optimisation des réseaux et de la gestion des transports - Amélioration des modèles climatiques - Sensibilisation au changement climatique - Applications dans la conception de matériaux innovants .references[ Rolnick et al. [Tackling Climate Change with Machine Learning](https://dl.acm.org/doi/10.1145/3485128), ACM Computing Surveys, 2022. ] --- ## Panorama des applications de l'IA pour l'ingénierie durable .highlight1[L'intelligence artificielle] ne doit pas être considérée comme la principale solution à la crise climatique, mais elle .highlight1[peut être utilisée dans de nombreux domaines] liés à l'.highlight1[atténuation] du changement climatique et à l'.highglight1[adaptation] à celui-ci. - Optimisation énergétique des bâtiments et infrastructures. - Optimisation de la production et de la distribution d'énergie - Prédiction et gestion des ressources naturelles. - Optimisation des réseaux et de la gestion des transports - Amélioration des modèles climatiques - .highlight2[Sensibilisation au changement climatique] - .highlight2[Applications dans la conception de matériaux innovants] .references[ Rolnick et al. [Tackling Climate Change with Machine Learning](https://dl.acm.org/doi/10.1145/3485128), ACM Computing Surveys, 2022. ] --- name: title class: title, middle ## Cas concret ### Sensibilisation au changement climatique .center[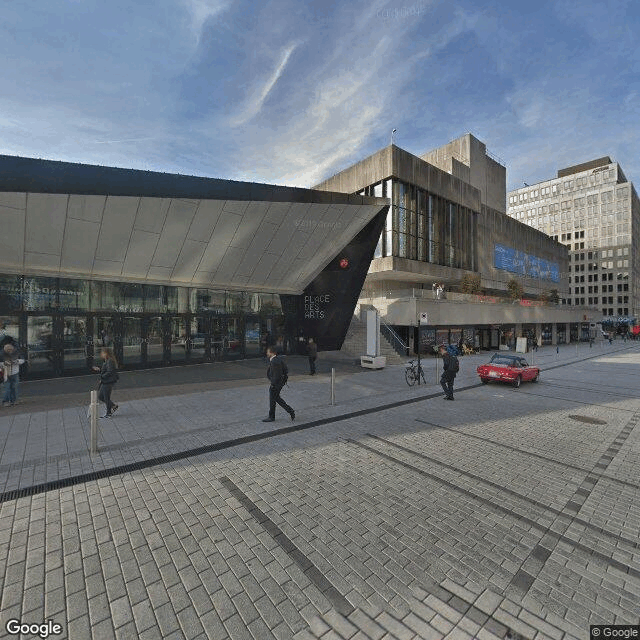] --- ## Sensibilisation au changement climatique Il y a un décalage entre la gravité de la crise climatique et les préoccupations du public à ce sujet. .center[
.smaller[
Stokes et al.,
Global concern about climate change, broad support for limiting emissions
. Pew Research, 2015
]
] --- count: false ## Cas concret ### Sensibilisation au changement climatique Il y a un décalage entre la gravité de la crise climatique et les préoccupations du public à ce sujet. .highlight1[_Pourquoi ?_] -- * .highlight1[Distance psychologique]: > "_People struggle to engage with climate change because they perceive it as distant: temporally, geographically and/or socially. _" .cite[Stoknes, 2016] .references[ * Stoknes, P. E. [Why the human brain ignores climate change—and what to do about it](https://documentcloud.adobe.com/link/track?uri=urn%3Aaaid%3Ascds%3AUS%3A1ef80b88-177c-4e5d-b879-d6d3a059c694). Environmental Reality: Rethinking the Options, 2016. ] ??? The dot next to US is Australia, then Canada, then Russia. --- count: false ## Cas concret ### Sensibilisation au changement climatique Il y a un décalage entre la gravité de la crise climatique et les préoccupations du public à ce sujet. .highlight1[_Pourquoi ?_] * .highlight1[Distance psychologique]: > "_People struggle to engage with climate change because they perceive it as distant: temporally, geographically and/or socially. _" .cite[Stoknes, 2016] * .highlight1[Doom-framings et fatigue des messages clichés]: > "_[C]lichéd images of climate change [...]—such as ‘smokestacks’, deforestation, and polar bears on melting ice—were positively received [but] also produced a muted emotional response and often prompted cynicism._" .cite[Chapman et al., 2016] .references[ * Stoknes, P. E. [Why the human brain ignores climate change—and what to do about it](https://documentcloud.adobe.com/link/track?uri=urn%3Aaaid%3Ascds%3AUS%3A1ef80b88-177c-4e5d-b879-d6d3a059c694). Environmental Reality: Rethinking the Options, 2016. * Chapman, D. A. et al. [Climate visuals: A mixed methods investigation of public perceptions of climate images in three countries](https://sci-hub.st/https://www.sciencedirect.com/science/article/abs/pii/S095937801630351X). GCE, 2016. ] --- count: false ## Cas concret ### Sensibilisation au changement climatique Il y a un décalage entre la gravité de la crise climatique et les préoccupations du public à ce sujet. .highlight1[_Pourquoi ?_] * .highlight1[Distance psychologique]: > "_People struggle to engage with climate change because they perceive it as distant: temporally, geographically and/or socially. _" .cite[Stoknes, 2016] * .highlight1[Doom-framings et fatigue des messages clichés]: > "_[C]lichéd images of climate change [...]—such as ‘smokestacks’, deforestation, and polar bears on melting ice—were positively received [but] also produced a muted emotional response and often prompted cynicism._" .cite[Chapman et al., 2016] .conclusion[Une plus grande sensibilisation du public pourrait accélérer les changements politiques. L'IA peut-elle aider ?] --- ## Notre objectif ### .alpha0[Placeholder] .context[On perçoit la menace du changement climatique comme étant éloignée dans le temps, géographiquement et socialement.] --
.center[.bigger[.highlight1[Est-il possible d'aider les gens à visualiser les effets du changement climatique chez eux ?]]] -- .left-column[
Montréal, Québec, Canada
] .right-column[
Berlin, Germany
] .left[ ] .right[ ] --- count: false ## Notre objectif ### Inondations .context[On perçoit la menace du changement climatique comme étant éloignée dans le temps, géographiquement et socialement.]
.center[.bigger[.highlight1[Est-il possible d'aider les gens à visualiser les effets du changement climatique chez eux ?]]] .left-column[
Montréal, Québec, Canada
] .right-column[
Berlin, Germany
] .left[ ] .right[ ] --- count: false ## Notre objectif ### Feux de fôret .context[On perçoit la menace du changement climatique comme étant éloignée dans le temps, géographiquement et socialement.]
.center[.bigger[.highlight1[Est-il possible d'aider les gens à visualiser les effets du changement climatique chez eux ?]]] .left-column[
Montréal, Québec, Canada
] .right-column[
Berlin, Germany
] .left[ ] .right[ ] --- count: false ## Notre objectif ### Smog .context[On perçoit la menace du changement climatique comme étant éloignée dans le temps, géographiquement et socialement.]
.center[.bigger[.highlight1[Est-il possible d'aider les gens à visualiser les effets du changement climatique chez eux ?]]] .left-column[
Montréal, Québec, Canada
] .right-column[
Berlin, Germany
] .left[ ] .right[ ] --- ## Notre objectif Un site web pour encourager la sensibilisation et l'action en matière de changement climatique. .context[On peut rechercher l'adresse de notre choix.] .center[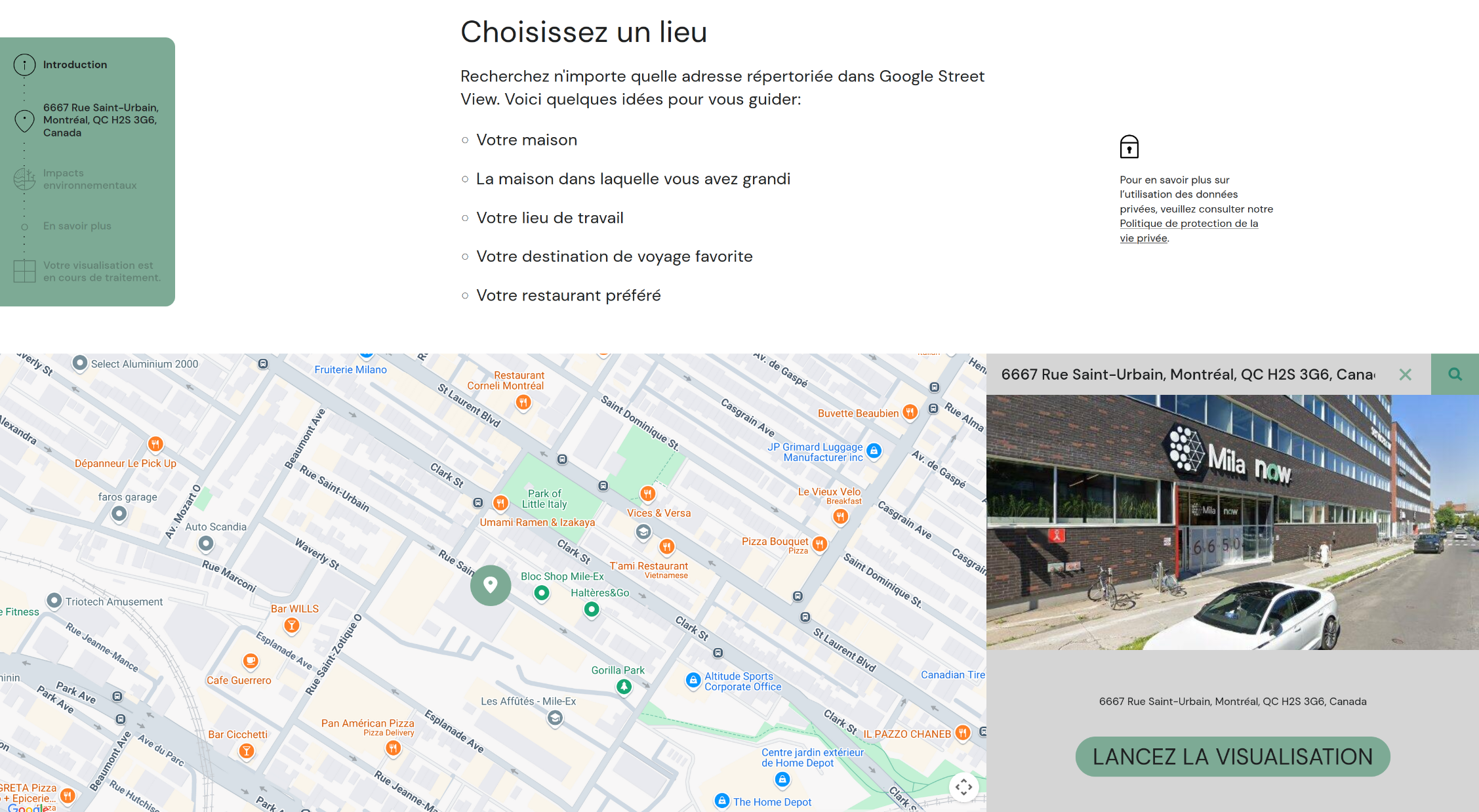] --- count: false ## Notre objectif Un site web pour encourager la sensibilisation et l'action en matière de changement climatique. .context65[Obtenir une visualisation générée par l'IA sur une photo de rue.] .center[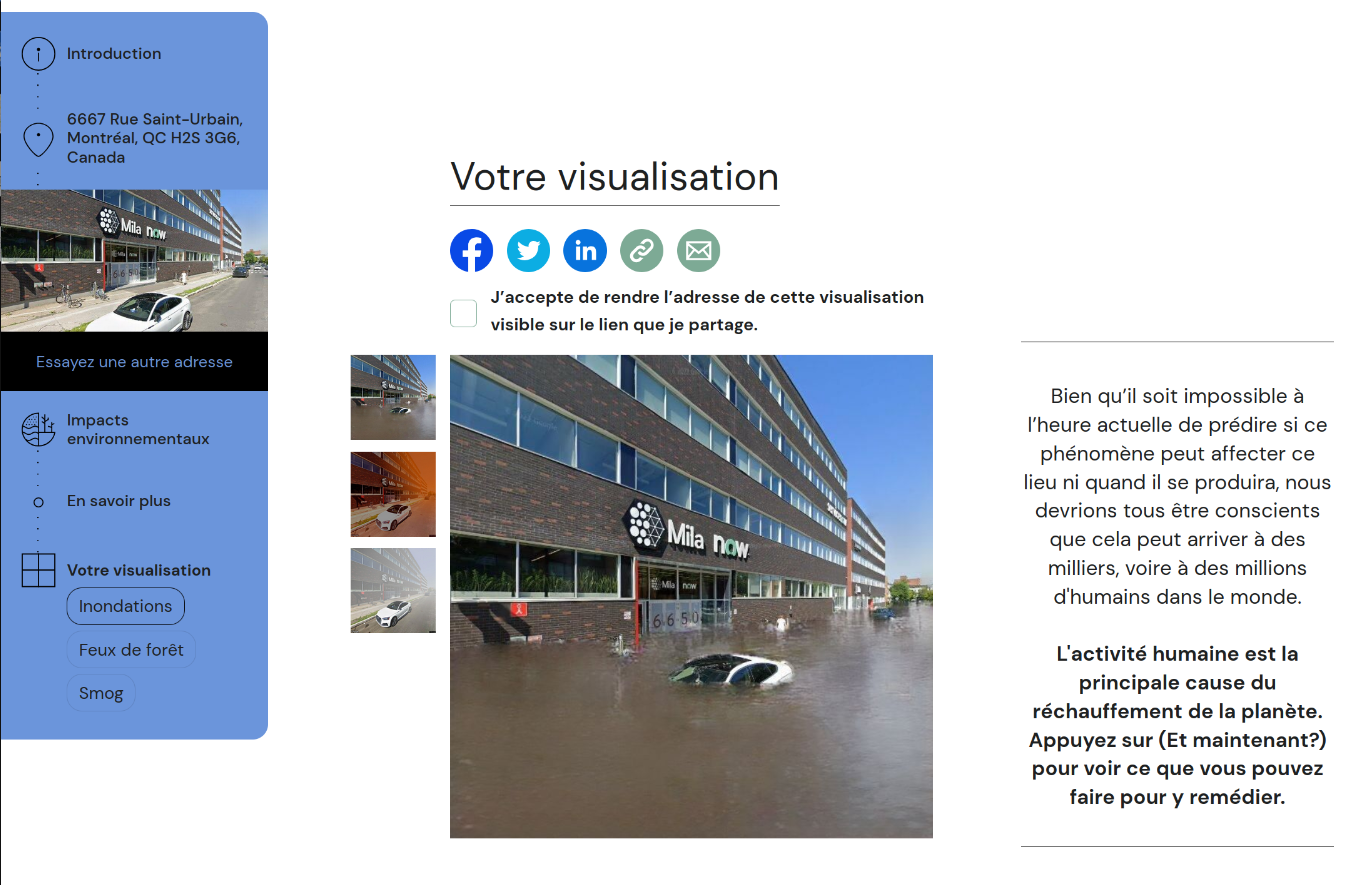] --- count: false ## Notre objectif Un site web pour encourager la sensibilisation et l'action en matière de changement climatique. .context65[En savoir plus sur le changement climatique et les moyens d'agir maintenant.] .center[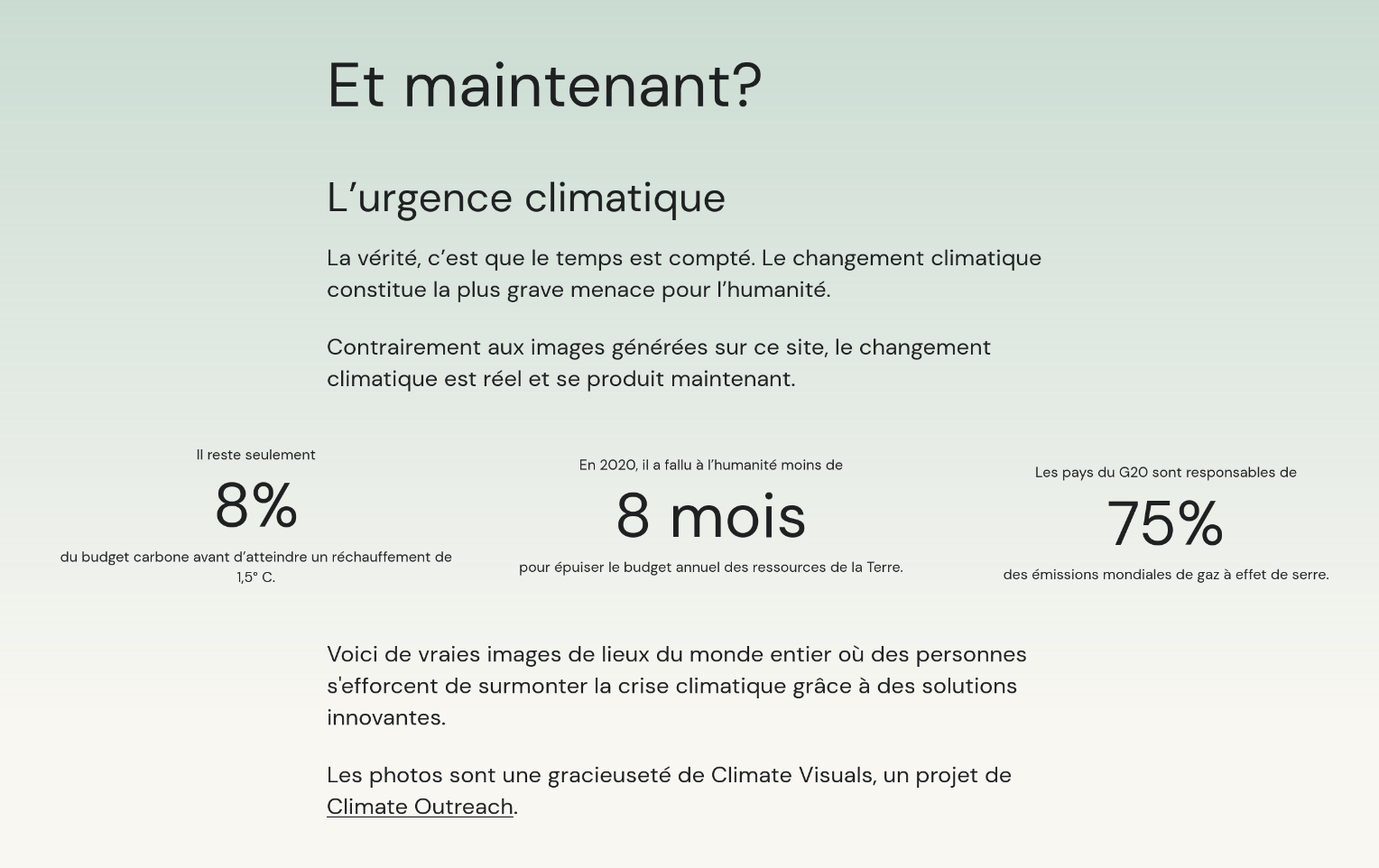] --- count: false ## Essayons-la ! .center[ .bigger[.bigger[[CeClimatNExistePas.com](https://ceclimatnexistepas.com)]] ] .center[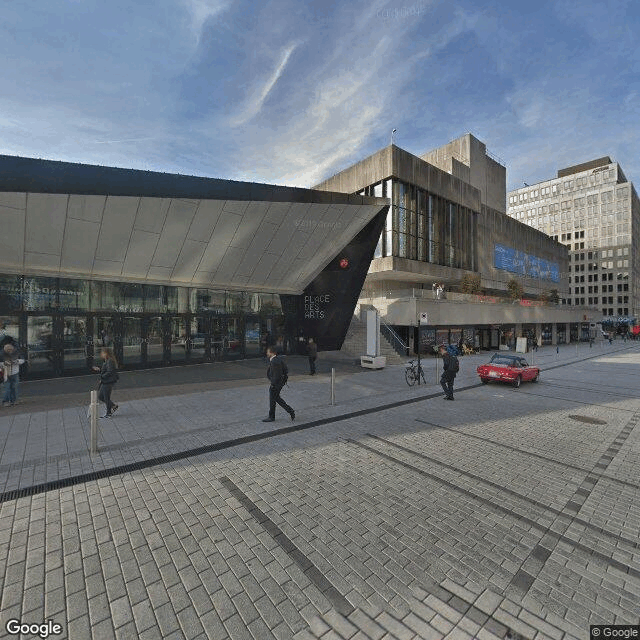] ??? https://thisclimatedoesnotexist.com/en/share/56d8058c-23d5-4083-b1b4-4afe6a5b2fe9 --- ## Méthodes ### Défis principaux .context[L'algorithme devait être capable de générer des inondations réalistes sur n'importe quelle photo de Google Street View.] -- .left-column-66[ * La perception visuelle est sensible aux scènes irréalistes : * Texture de l'eau (reflets, luminosité, etc.) * Géométrie de la scène (bords, obstacles, etc.) * Physique (pente, point de vue, etc.) * L'algorithme a été conçu pour être déployé _in the wild_ et devrait fonctionner avec une grande variété de photos. * Nous avons dû surmonter le manque de données d'entraînement : il n'existe pas de bases de donnés de photos avant et après une inondation. ] .right-column-33[ .center[ 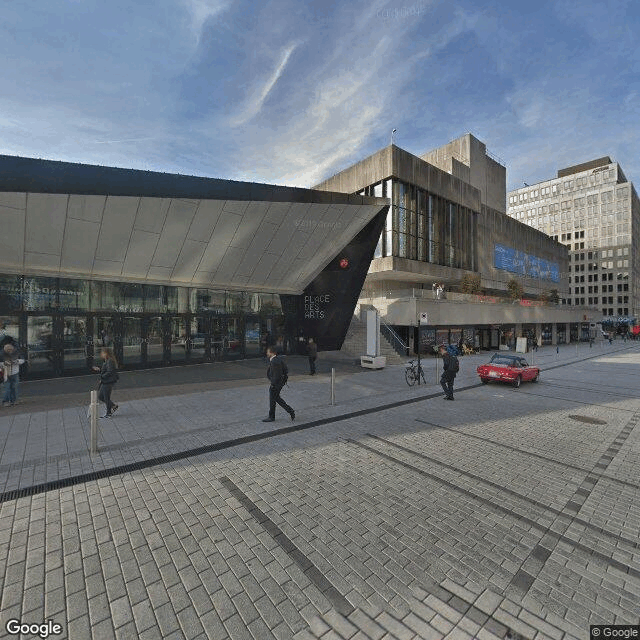 ] ] --- ## Methods ### Caractéristiques principales .context[La simulation d'inondations photo-réalistes est un défi car la perception visuelle est très sensible aux scènes irréalistes et au manque de données.] -- .left-column[ * Des données provenant d'un .highlight1[monde virtuel simulé] pour pallier le manque de données de formation * .highlight1[Domain adaptation] pour réduire l'écart entre les photos simulées et les photos réelles * Génération d'inondations en deux temps : .highlight1[Masker] + .highlight1[Painter] * Combinaison de la .highlight1[segmentation de profondeur] et de la .highlight1[segmentation sémantique] pour améliorer les prédictions de masque d'eau * .highlight1[Génération d'images conditionnelles] pour peindre de l'eau réaliste sur le masque prédit ] .right-column[ 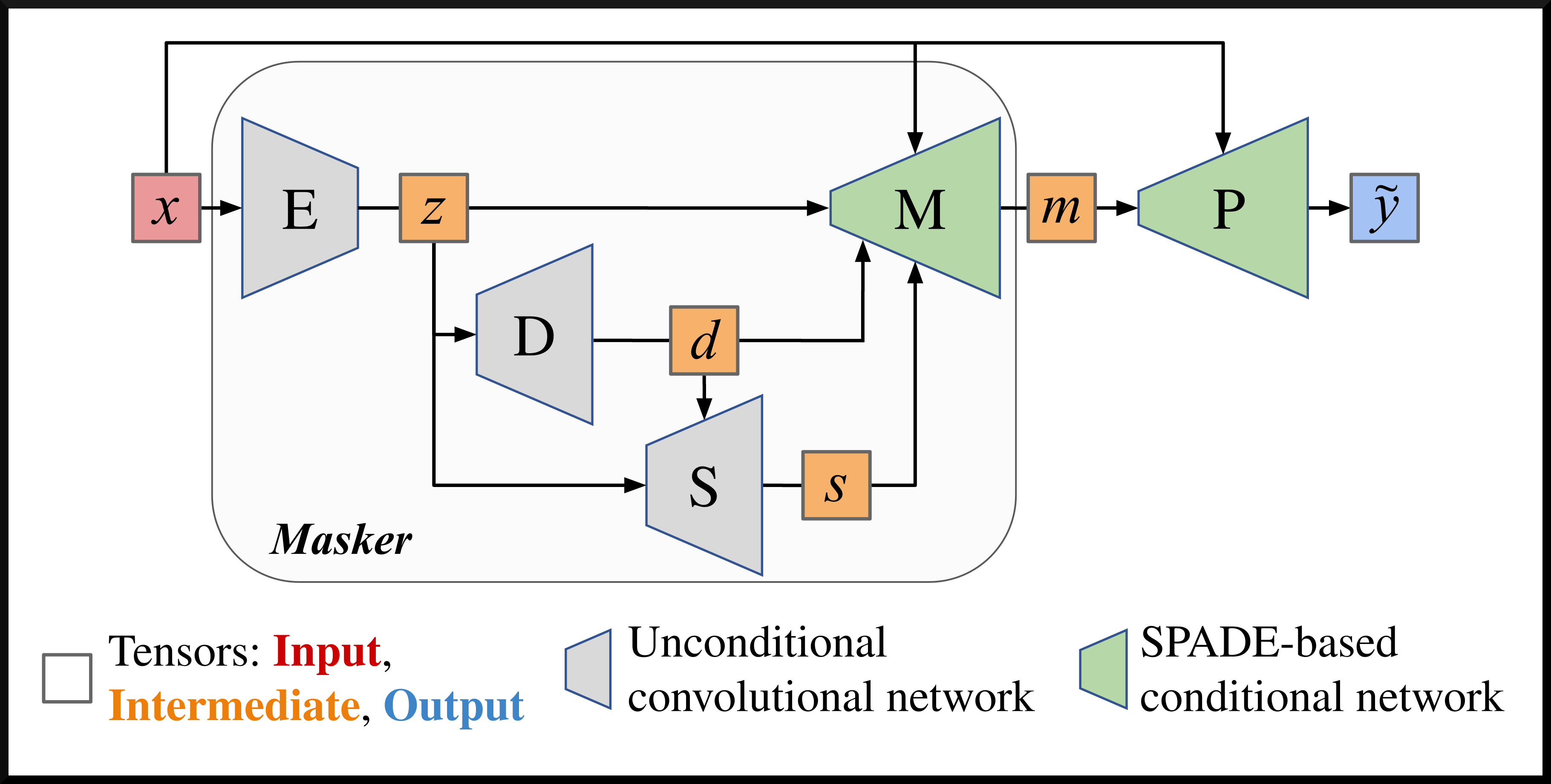 ] --- ## Pour en savoir plus Visitez le site web : [CeClimatNEXistePas.com](https://ceclimatnexistepas.com) .center[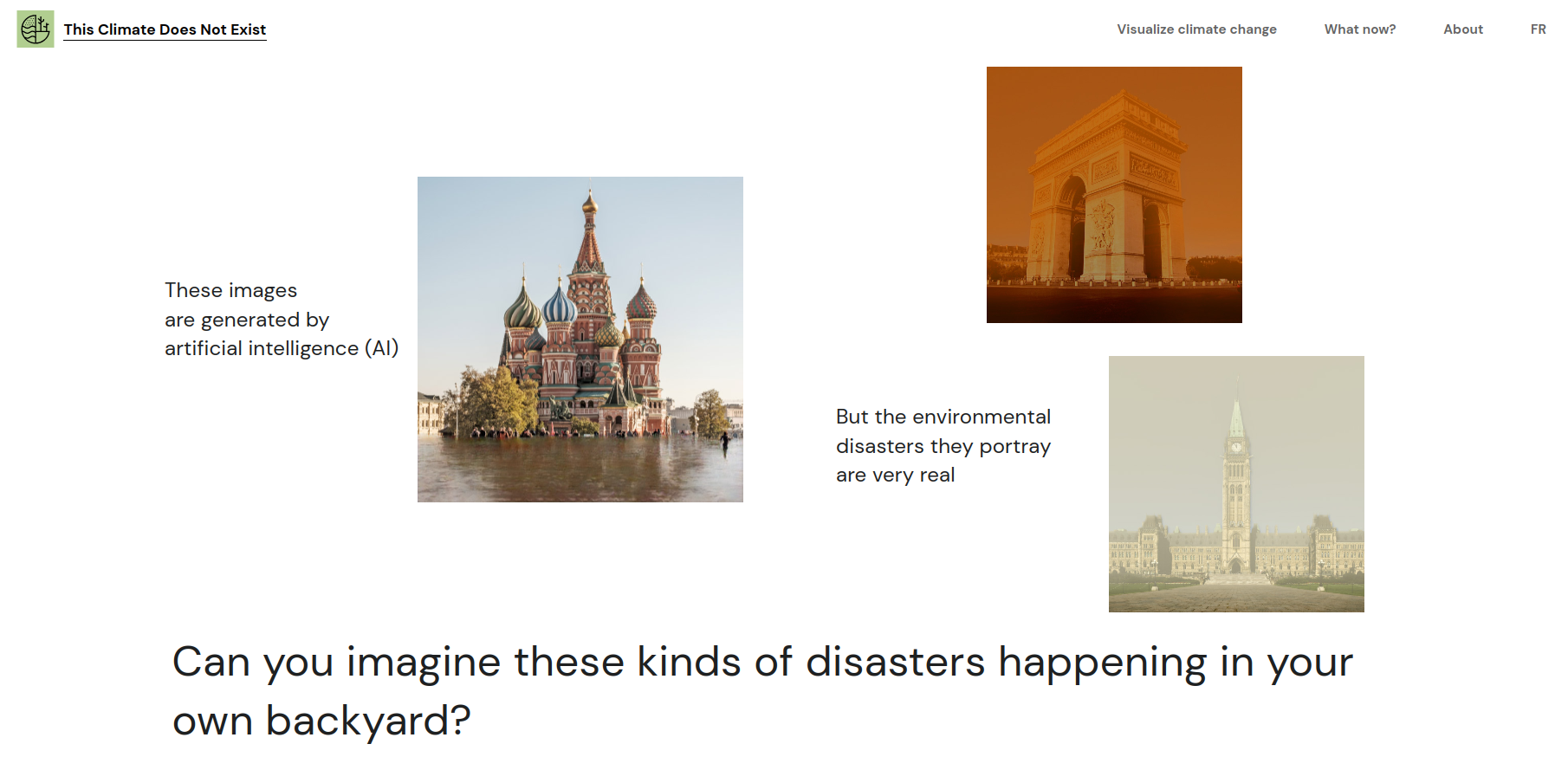] Lisez l'article (ICLR 2022): [ClimateGAN: Raising Climate Change Awareness by Generating Images of Floods](https://arxiv.org/abs/2110.02871v1) .center[] --- name: title class: title, middle ## Cas concret ### Découverte de matériaux .center[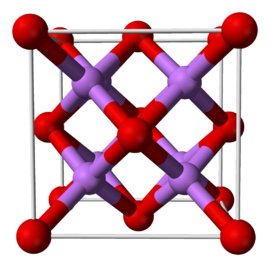] --- ## Le potentiel des découvertes scientifiques * L'amélioration de l'efficacité des matériaux peut réduire 0.93 ($\pm$ 0.23) GtCO₂-eq par an. * Le changement de combustible peut réduire 2.1 ($\pm$ 0.52) GtCO₂-eq par an, uniquement dans le secteur industriel. * Le captage et le stockage du carbone peuvent réduire 0.54 ($\pm$ 0.27) GtCO₂-eq par an, dans le secteur énergetique. .right[.cite[IPCC Sixth Assessment Report (2022)]] .smaller[.footnote[† Global anthropogenic emissions in 2019 were estimated in 59 ($\pm$ 6.6) GtCO₂-eq. The budget from 2020 to limit warming to 1.5°C is estimated in 510 ($\pm$ 180) GtCO₂-eq.]] --- count: false ## Le potentiel des découvertes scientifiques * L'amélioration de l'efficacité des matériaux peut réduire 0.93 ($\pm$ 0.23) GtCO₂-eq par an. * Le changement de combustible peut réduire 2.1 ($\pm$ 0.52) GtCO₂-eq par an, uniquement dans le secteur industriel. * Le captage et le stockage du carbone peuvent réduire 0.54 ($\pm$ 0.27) GtCO₂-eq par an, dans le secteur énergetique. .right[.cite[IPCC Sixth Assessment Report (2022)]] Quels sont les besoins en matériaux innovants ? * Électrocatalyseurs pour les piles à combustible, le stockage de l'hydrogène, les réactions chimiques industrielles, la capture du carbone, etc. * Électrolytes solides pour batteries. * _Thin film materials_ pour l'énergie photovoltaïque. * ... .smaller[.footnote[† Global anthropogenic emissions in 2019 were estimated in 59 ($\pm$ 6.6) GtCO₂-eq. The budget from 2020 to limit warming to 1.5°C is estimated in 510 ($\pm$ 180) GtCO₂-eq.]] --- ## Découvertes scientifiques dans l'histoire De nombreuses découvertes scientifiques notables ont été faites par .highlight1[sérendipité] ou par .highlight1[accident] : -- * **Dynamite** (Alfred Nobel, 1867) * **X-rays** (Wilhelm C. Röntgen, 1895) * **Radioactivity** (Henri Becquerel and Marie Skłodowska–Curie, 1896) * **Penicillin** (Alexander Fleming, 1929) * **Cyanoacrylate (superglue)** (Harry Coover, 1942) * **Lysergic acid diethylamide (LSD)** (Albert Hofmann, 1943) --
.conclusion[Il est clair que nous ne devons pas compter sur la sérendipité pour lutter contre le changement climatique.] --- count: false ## Découvertes scientifiques dans l'histoire .center[
Quatre paradigmes dans la découverte scientifique. Source:
Li et al., 2022
. (
CC BY 4.0
)
] .references[ * Li et al. [Machine learning in concrete science: applications, challenges, and best practices](https://www.nature.com/articles/s41524-022-00810-x). Nature npj Computational Materials, 2022 ] ??? Example of concrete: most prevalent human-made material on Earth, and the most consumed commodity after water. The annual consumption of concrete in the world has reached 35 billion tons, which is twice as much as that of all other building materials combined. --- count: false ## Découvertes scientifiques dans l'histoire .center[
Quatre paradigmes dans la découverte scientifique. Source:
Li et al., 2022
. (
CC BY 4.0
)
] .references[ * Li et al. [Machine learning in concrete science: applications, challenges, and best practices](https://www.nature.com/articles/s41524-022-00810-x). Nature npj Computational Materials, 2022 ] ??? Concrete: The properties and performance of concrete can be tailored to meet design requirements by varying the type and quantity of the mixture constituents (e.g., cement, water, aggregate, and admixtures). Traditional approaches for designing concrete mixtures often rely on trial-and-error, iterative proportioning, processing, and characterization until the target properties are achieved. --- count: false ## Découvertes scientifiques dans l'histoire .center[
Quatre paradigmes dans la découverte scientifique. Source:
Li et al., 2022
. (
CC BY 4.0
)
] .references[ * Li et al. [Machine learning in concrete science: applications, challenges, and best practices](https://www.nature.com/articles/s41524-022-00810-x). Nature npj Computational Materials, 2022 ] ??? Concrete: it is possible to optimize the compressive strength of concrete mixtures by adjusting the water/cement ratio, total aggregate/cement ratio, and coarse aggregate/total aggregate ratio6. Yet the practical application of this iterative refinement approach is limited by the exponential increase in the number of specimens and experiments when complex concrete mixtures are studied and several compositional parameters are simultaneously considered as combinatorial variables. As a result, materials development in concrete science involves time-consuming validation/development cycles from laboratory trials to field applications. Efforts to accelerate knowledge acquisition and materials design in concrete science are thus of paramount importance. Beginning in the 1980s, the development of microstructural models of cement hydration has enabled a fundamental understanding of microstructure–property relationships in concrete7, which has marked the second paradigm. By applying basic laws of kinetics, thermodynamics, and mechanics, and providing analytical solutions to cement hydration. Successful demonstrations include the three-dimensional cement hydration and microstructure development model (CEMHYD3D)8,9; the hydration, morphology, and structural development model (HYMOSTRUC)10; the integrated particle kinetics model11; and the microstructural modeling platform (μic) --- count: false ## Découvertes scientifiques dans l'histoire .center[
Quatre paradigmes dans la découverte scientifique. Source:
Li et al., 2022
. (
CC BY 4.0
)
] .references[ * Li et al. [Machine learning in concrete science: applications, challenges, and best practices](https://www.nature.com/articles/s41524-022-00810-x). Nature npj Computational Materials, 2022 ] ??? Concrete: However, the complex nature of cement hydration makes it challenging to develop accurate and generalizable models, and these modeling approaches, to varying degrees, rely on thermochemical, physical, and structural data that must be obtained either from accurate experimental observations or from calculations at the atomistic and molecular scales. In this context, the use of density-functional theory (DFT) and classical molecular dynamics (MD) simulations has been explored in concrete science since the 2000s owing to the ever-growing computing power16. This has given rise to the third paradigm (computational science; Fig. 1), where the first-principle models have been integrated and employed to further describe cementitious materials properties and improve understanding of cement hydration. Related simulation efforts have focused primarily on cementitious phases such as the calcium silicate hydrate (C-S-H) gel, the essential reaction product of cement hydration. --- count: false ## Découvertes scientifiques dans l'histoire .center[
Quatre paradigmes dans la découverte scientifique. Source:
Li et al., 2022
. (
CC BY 4.0
)
] .references[ * Li et al. [Machine learning in concrete science: applications, challenges, and best practices](https://www.nature.com/articles/s41524-022-00810-x). Nature npj Computational Materials, 2022 ] ??? Concrete: However, these computational techniques require considerable computational resources and thus come with significant challenges, such as their limited time scales and the relatively small number of atoms in a simulated system. In addition, it may be difficult to validate these simulations with experiments, given the small time and length scales and high-fidelity measurements required. By leveraging existing datasets with data-driven models, ML can automatically learn implicit patterns and extract valuable information while accounting for the inherent complexity of concrete mixtures and their properties. --- ## Cycle de découverte traditionnel .context35[La crise climatique nécessite une accélération des découvertes scientifiques.] -- .right-column-66[
.center[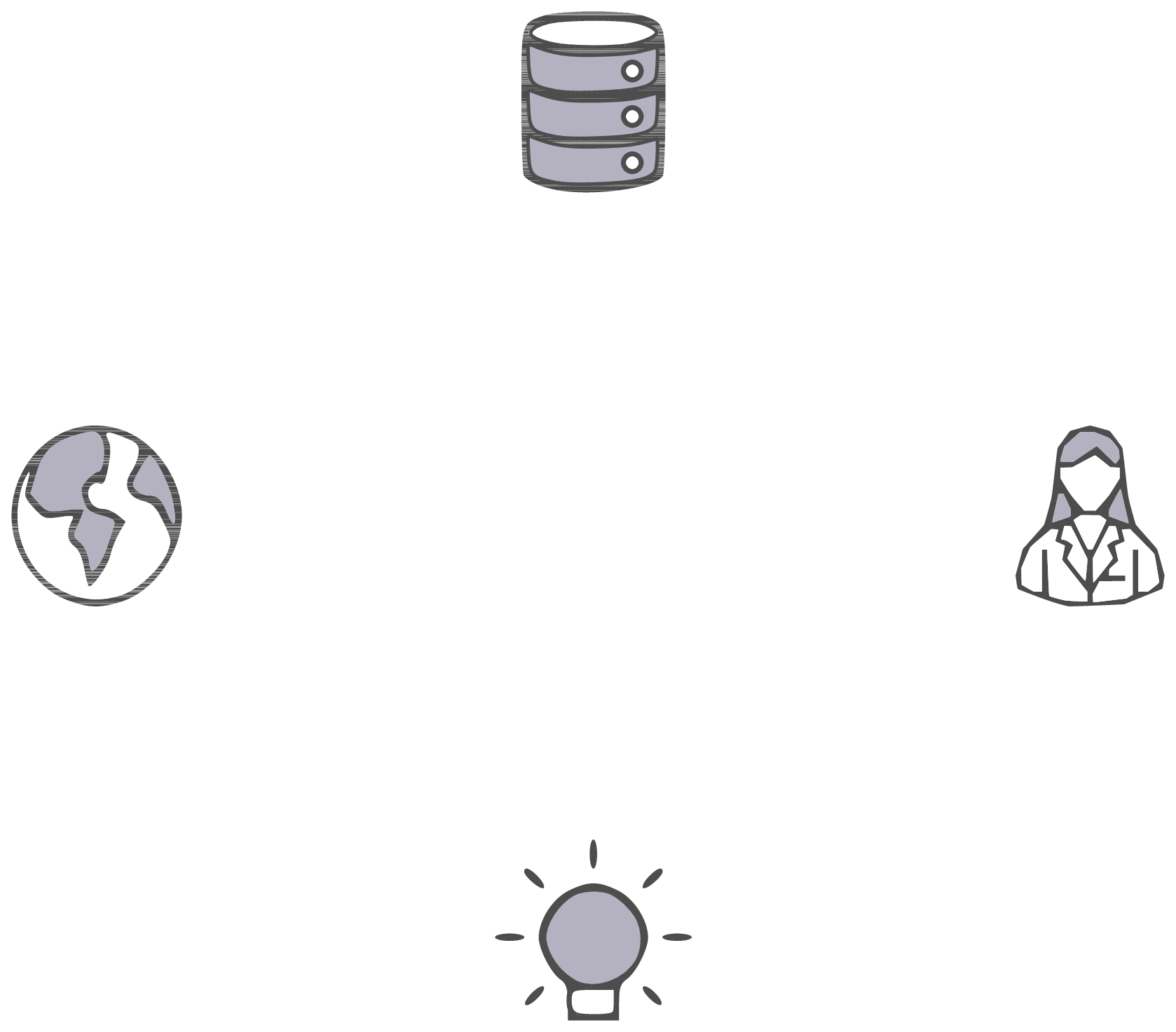]] .left-column-33[
Le .highlight1[cycle traditionnnel] pour la découverte scientifique : * il repose .highlight1[sur l'expertise humaine], * il .highlight1[prend du temps] et * il est .highlight1[coûteux financièrement et en termes de calcul]. ] --- count: false ## Apprentissage (machine) _actif_ .context35[Le cycle traditionnel est trop lent pour certaines applications.] .right-column-66[
.center[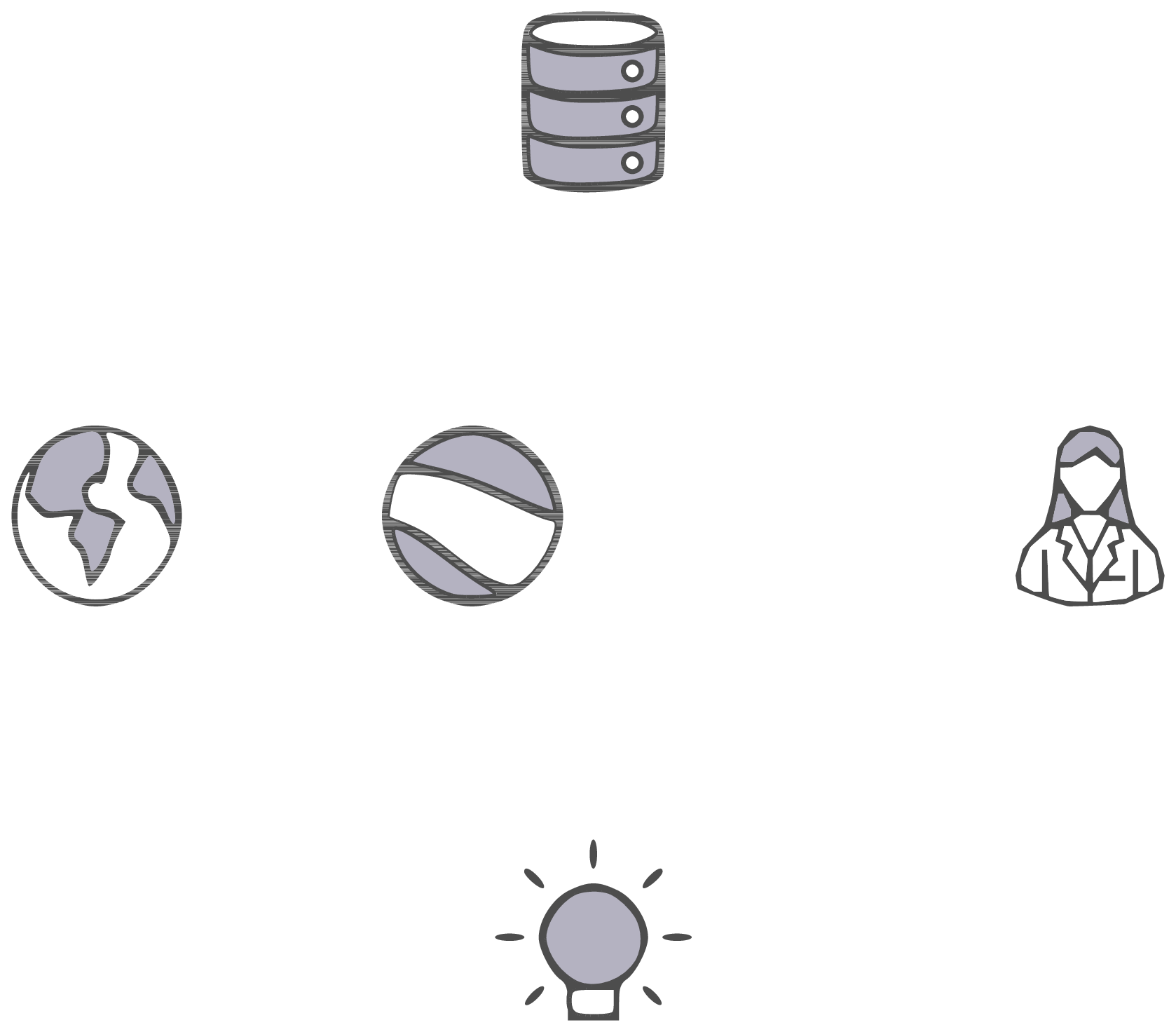]] .left-column-33[
Un .highlight1[modèle d'apprentissage automatique] peut être: * à partir de données provenant d'expériences réeles et ] --- count: false ## Apprentissage (machine) _actif_ .context35[Le cycle traditionnel est trop lent pour certaines applications.] .right-column-66[
.center[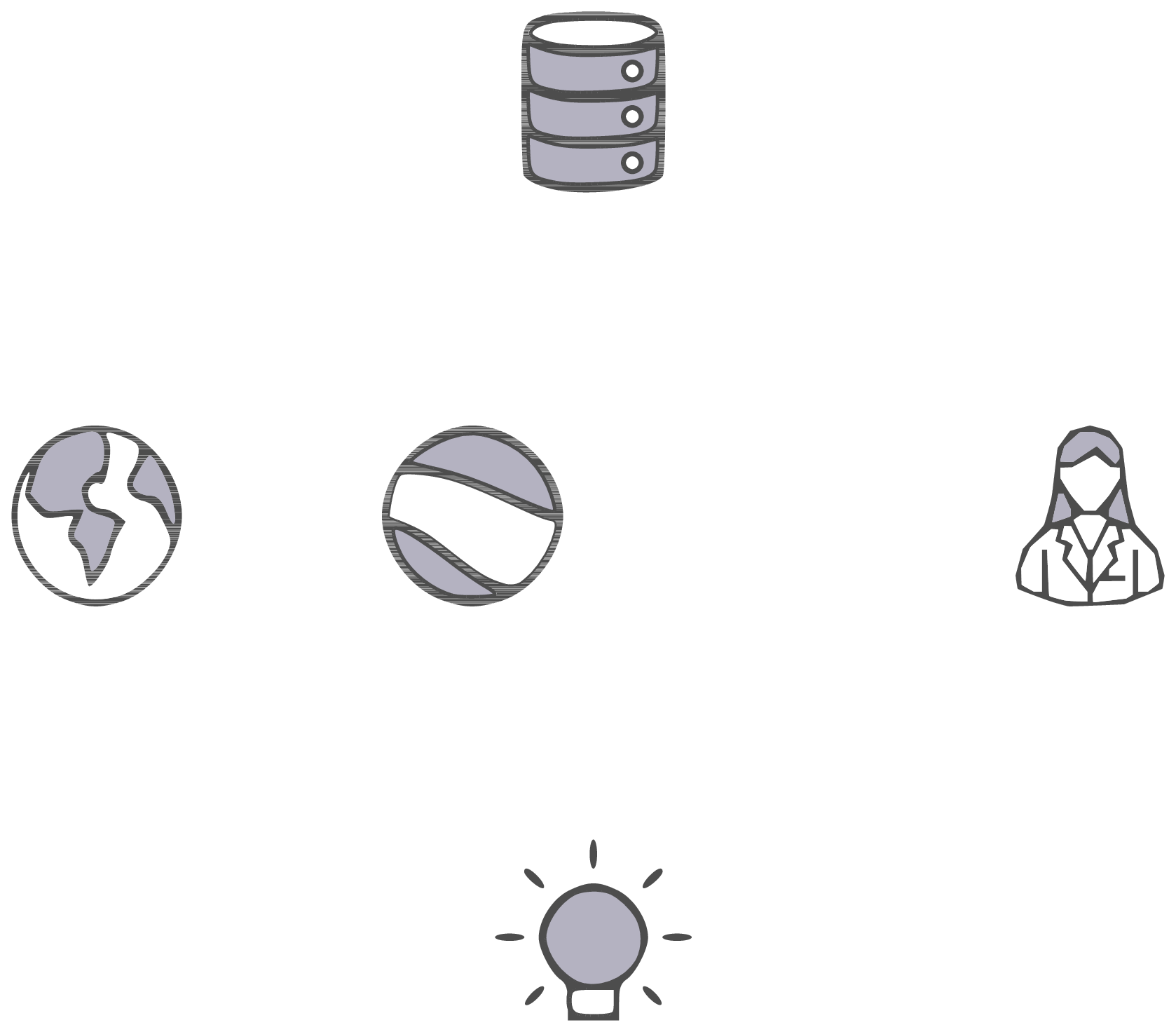]] .left-column-33[
Un .highlight1[modèle d'apprentissage automatique] peut être: * à partir de données provenant d'expériences réeles et * utilisé pour évaluer des candidats plus rapidement ] --- count: false ## Apprentissage (machine) _actif_ .context35[Le cycle traditionnel est trop lent pour certaines applications.] .right-column-66[
.center[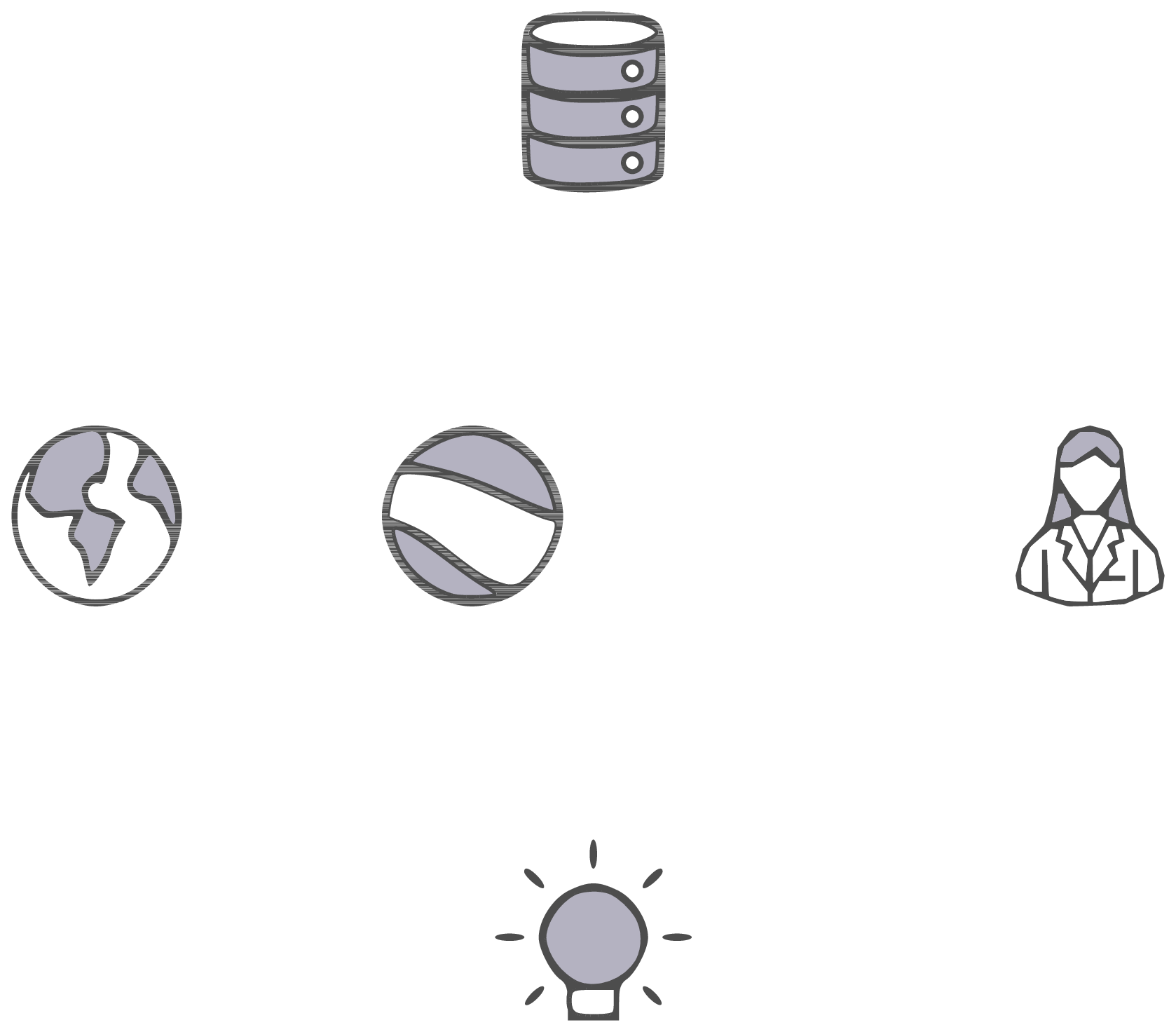]] .left-column-33[
Un .highlight1[modèle d'apprentissage automatique] peut être: * à partir de données provenant d'expériences réeles et * utilisé pour évaluer des candidats plus rapidement ] .conclusion[Il existe une infinité de matériaux concevables, $10^{180}$ potentiellement stable et $10^{60}$ molecules médicamenteuses. Les modèles prédictifs sont-ils suffisants ?] ] --- count: false ## Apprentissage (machine) _actif_ et _génératif_ .right-column-66[
.center[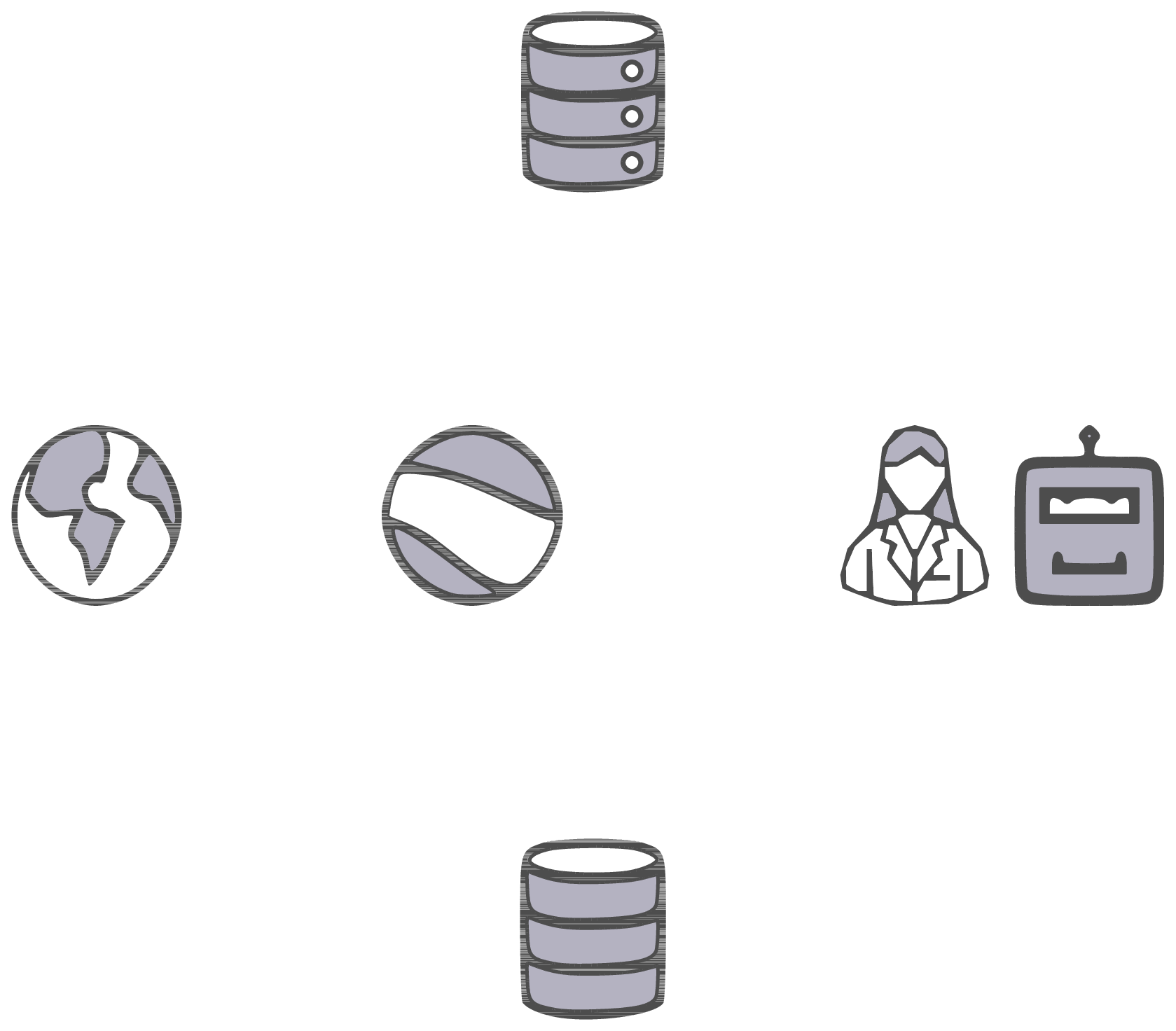]] .left-column-33[ .highlight1[L'IA générative] peut: * .highlight1[apprendre la structure] à partir des donnés disponibles, * .highlight1[généraliser] aux régions inexplorées de l'espace de recherche et * .highlight1[proposer des meilleures candidats] ] --- count: false ## Apprentissage (machine) _actif_ et _génératif_ .right-column-66[
.center[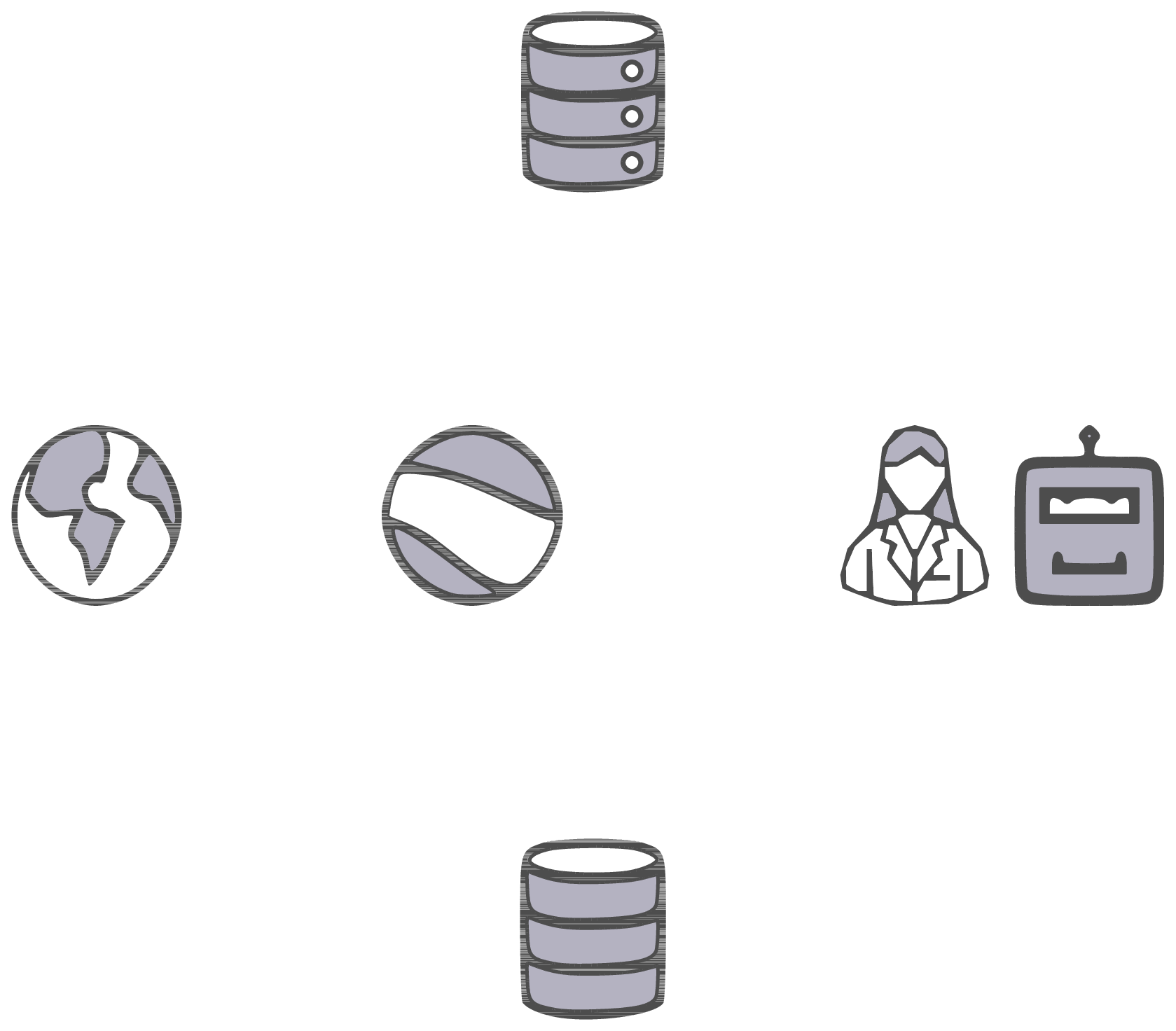]] .left-column-33[ .highlight1[L'IA générative] peut: * .highlight1[apprendre la structure] à partir des donnés disponibles, * .highlight1[généraliser] aux régions inexplorées de l'espace de recherche et * .highlight1[proposer des meilleures candidats] ] .conclusion[L'apprentissage actif avec l'apprentissage machine génératif peut en théorie explorer plus efficacement l'espace des candidats.] ] --- ## Résumé - Certains modèles actuels d'intelligence artificielle ont un impact environnemental important en raison de leur taille et de leur déploiement à grande échelle. - Mais tous les modèles ne sont pas énergivores. -- - En tant qu'ingénieurs, nous jouons un rôle important dans la durabilité du développement, de l'utilisation et de l'adoption de l'IA. -- - L'intelligence artificielle peut être appliquée dans de nombreux domaines pour contribuer à la lutte contre la crise climatique. Par exemple : - Sensibilisation au changement climatique - Découverte scientifique --- name: oiq-mar25 class: title, middle Alex Hernández-García (he/il/él) .center[
    
    
    
] .footer[[alexhernandezgarcia.github.io](https://alexhernandezgarcia.github.io/) | [alex.hernandez-garcia@mila.quebec](mailto:alex.hernandez-garcia@mila.quebec)] | [alexhergar.bsky.social](https://bsky.app/profile/alexhergar.bsky.social) [](https://bsky.app/profile/alexhergar.bsky.social)
.smaller[.footer[ Slides: [alexhernandezgarcia.github.io/slides/{{ name }}](https://alexhernandezgarcia.github.io/slides/{{ name }}) ]] --- count: false name: title class: title, middle ### The challenges of scientific discoveries .center[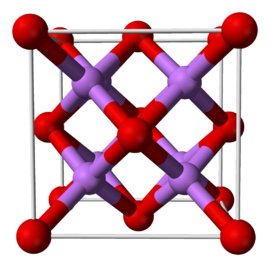] .center[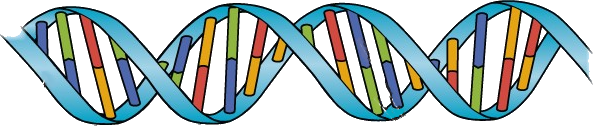] --- count: false ## An intuitive trivial problem .highlight1[Problem]: find one arrangement of Tetris pieces on the board that minimise the empty space. .left-column-33[ .center[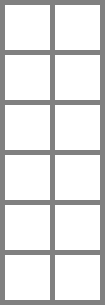] ] .right-column-66[ .center[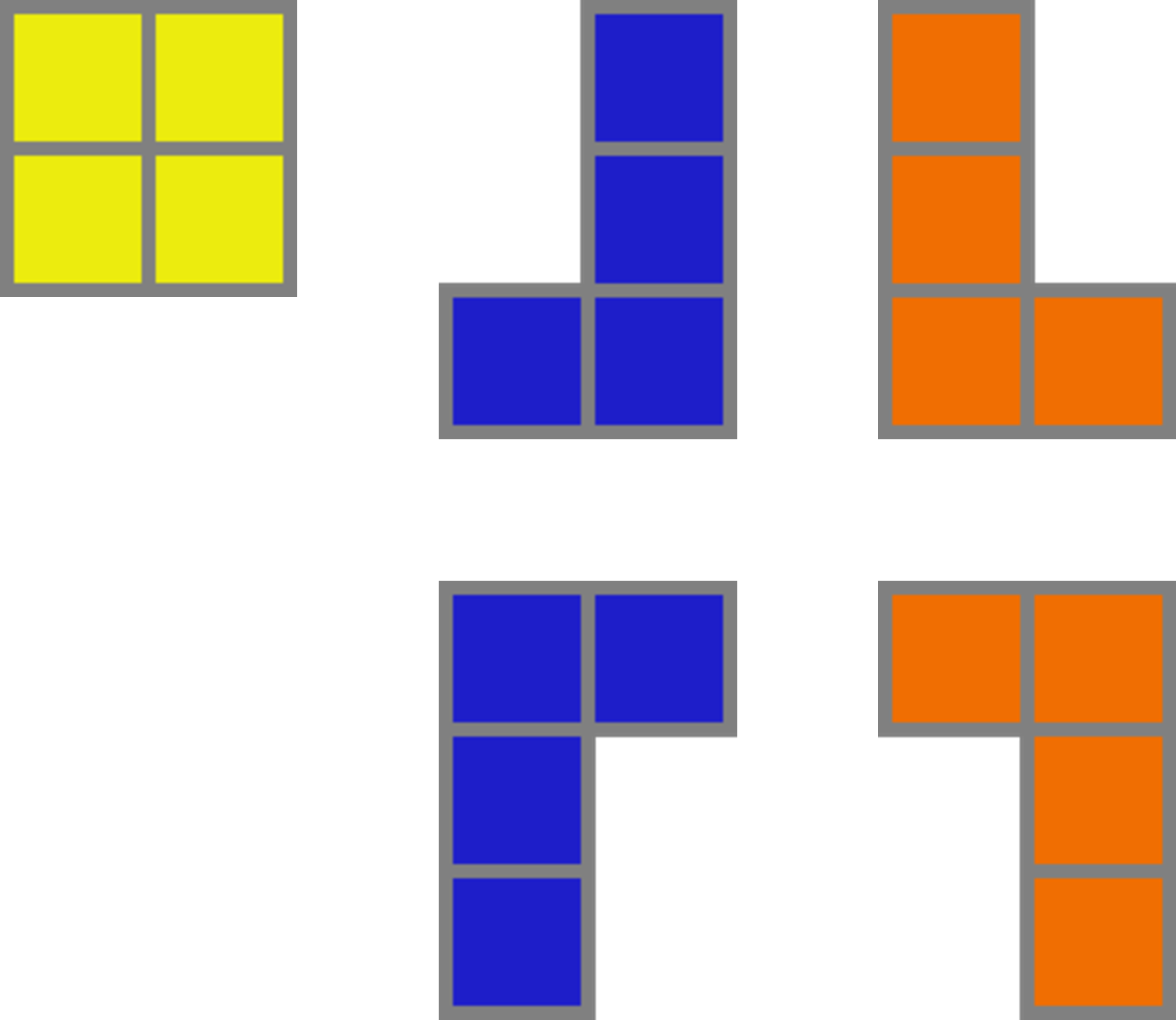] ] -- .full-width[.center[
Score: 12
]] --- count: false ## An intuitive ~~trivial~~ easy problem .highlight1[Problem]: find .highlight2[all] the arrangements of Tetris pieces on the board that minimise the empty space. .left-column-33[ .center[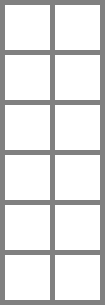] ] .right-column-66[ .center[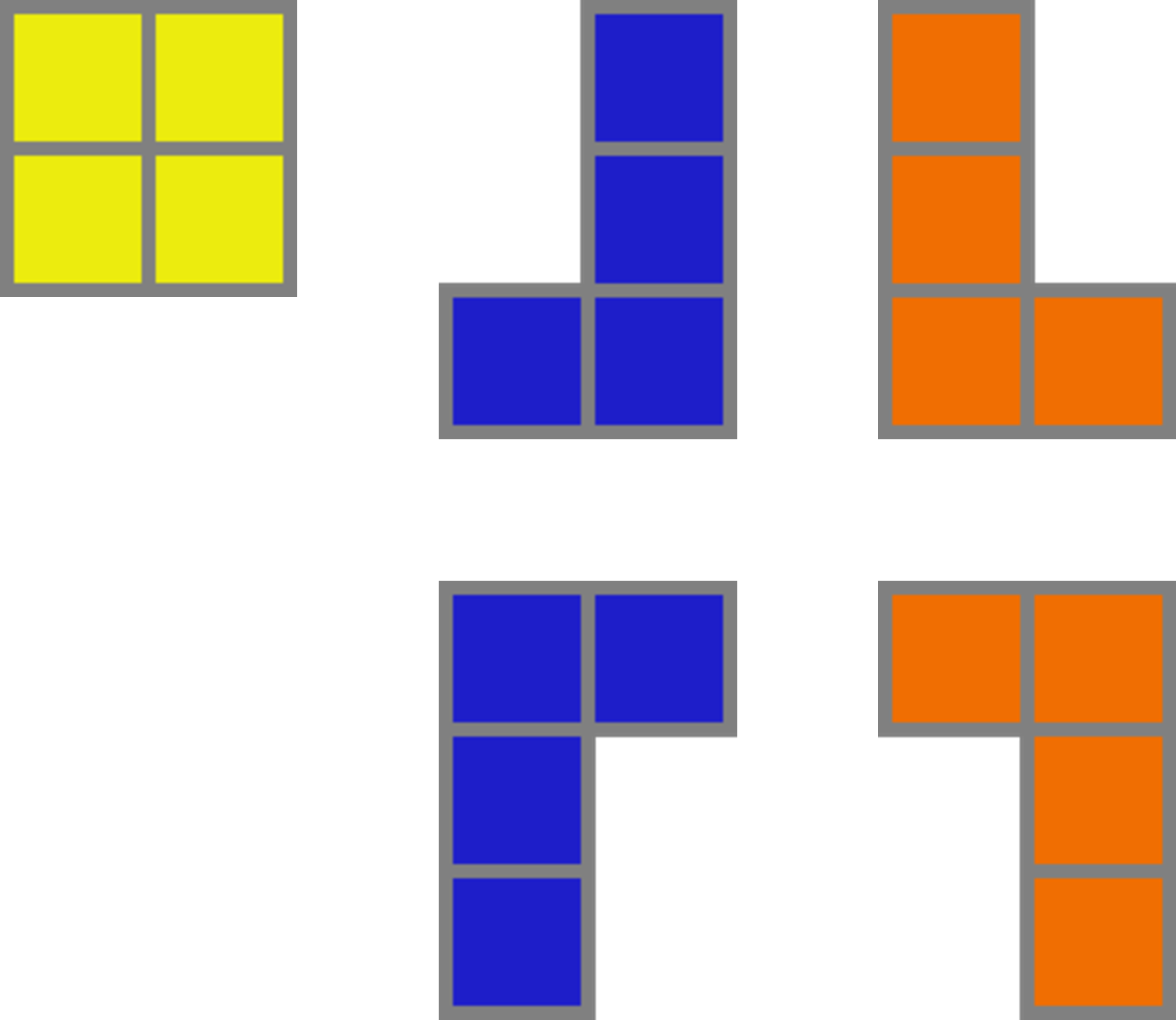] ] -- .full-width[.center[
12
12
12
12
12
]] --- count: false ## An intuitive ~~easy~~ hard problem .highlight1[Problem]: find .highlight2[all] the arrangements of Tetris pieces on the board that minimise the empty space. .left-column-33[ .center[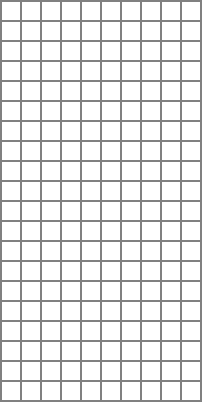] ] .right-column-66[ .center[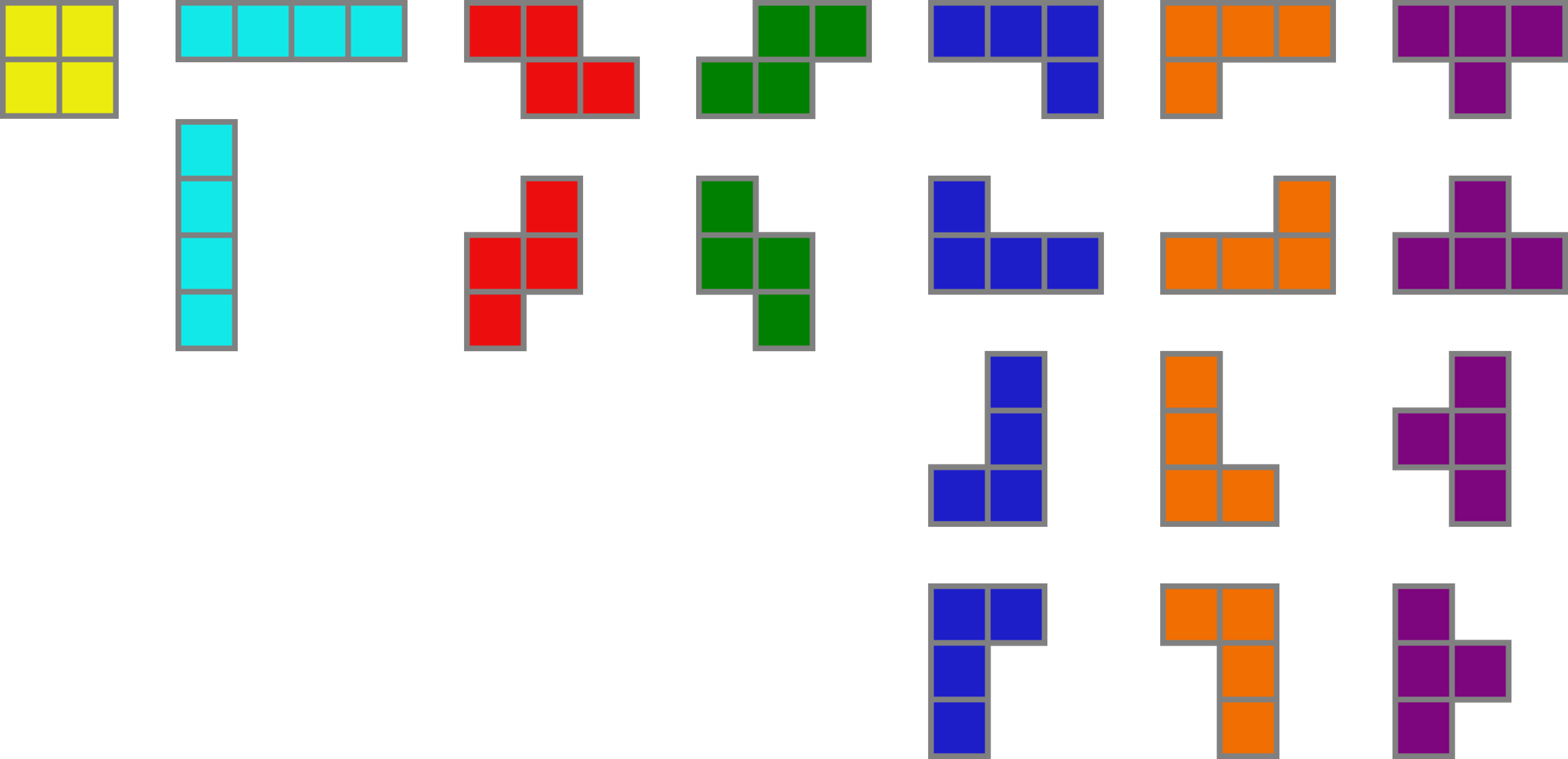] ] -- .full-width[.center[
]] --- count: false ## An incredibly ~~intuitive easy~~ hard problem .highlight1[Problem]: find .highlight2[all] the arrangements of Tetris pieces on the board that .highlight2[optimise an unknown function]. .left-column-33[ .center[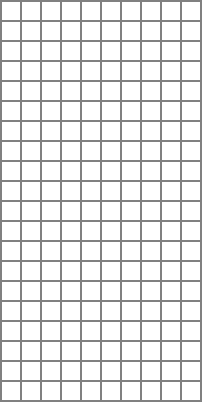] ] .right-column-66[ .center[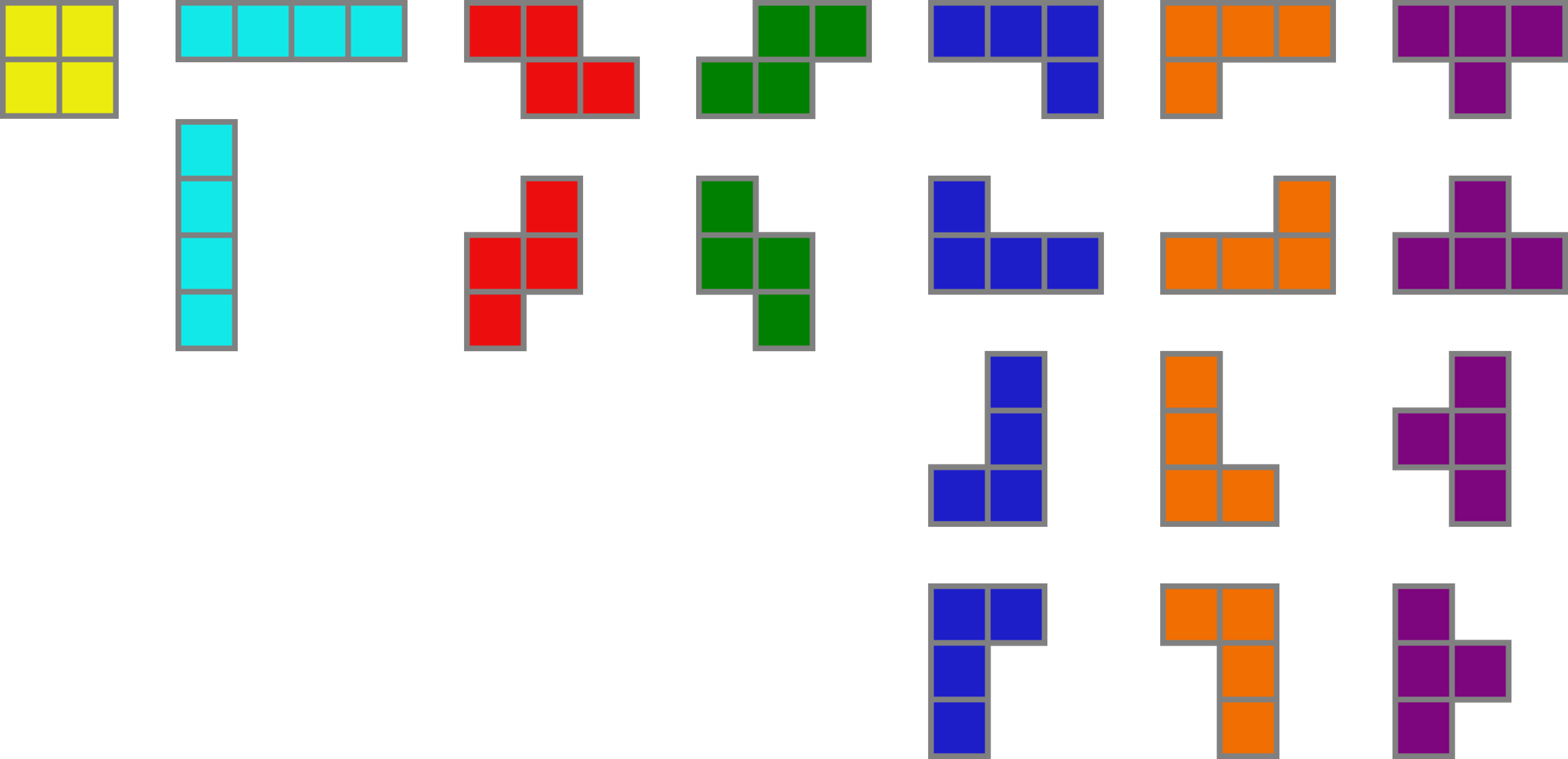] ] -- .full-width[.center[
]] --- count: false ## An incredibly ~~intuitive easy~~ hard problem .highlight1[Problem]: find .highlight2[all] the arrangements of Tetris pieces on the board that .highlight2[optimise an unknown function]. .left-column-33[ .center[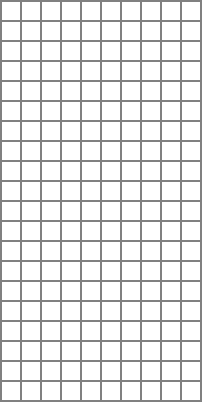] ] .right-column-66[ .center[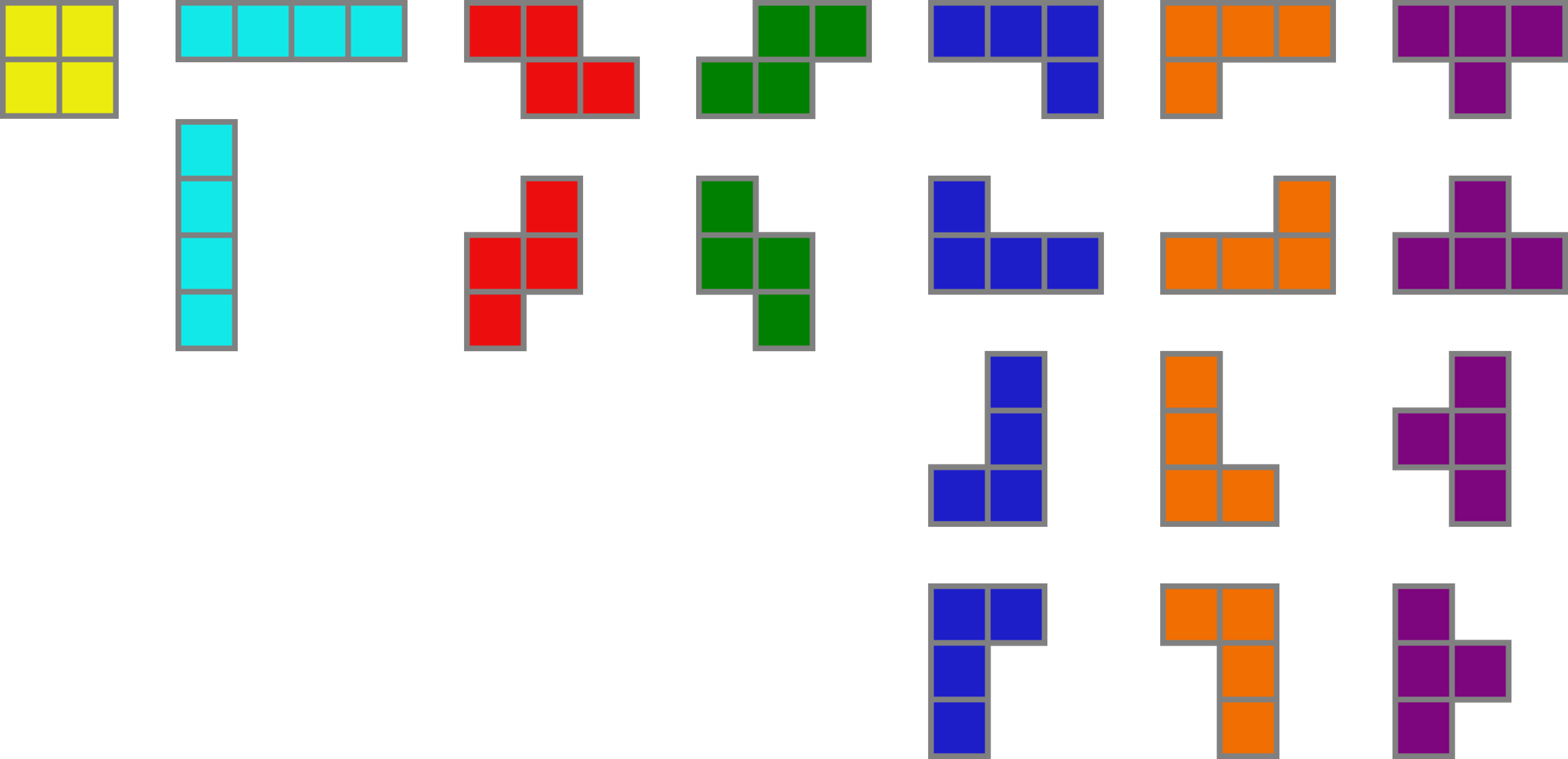] ] .full-width[.conclusion[Materials and drug discovery involve finding **diverse** candidates in **combinatorially or infinitely large search spaces**, with **rare properties** that are **expensive to evaluate**.]] --- count: false ## Why Tetris for scientific discovery? .context35[The "Tetris problem" involves .highlight1[sampling from an unknown distribution] in a .highlight1[discrete, high-dimensional, combinatorially large space].] --- count: false ## Why Tetris for scientific discovery? ### Biological sequence design
Proteins, antimicrobial peptides (AMP) and DNA can be represented as sequences of amino acids or nucleobases. There are $22^{100} \approx 10^{134}$ protein sequences with 100 amino acids. .context35[The "Tetris problem" involves sampling from an unknown distribution in a discrete, high-dimensional, combinatorially large space] .center[] .left-column-66[ .dnag[`G`].dnaa[`A`].dnag[`G`].dnag[`G`].dnag[`G`].dnac[`C`].dnag[`G`].dnaa[`A`].dnac[`C`].dnag[`G`].dnag[`G`].dnat[`T`].dnaa[`A`].dnac[`C`].dnag[`G`].dnag[`G`].dnaa[`A`].dnag[`G`].dnac[`C`].dnat[`T`].dnac[`C`].dnat[`T`].dnag[`G`].dnac[`C`].dnat[`T`].dnac[`C`].dnac[`C`].dnag[`G`].dnat[`T`].dnat[`T`].dnaa[`A`]
.dnat[`T`].dnac[`C`].dnaa[`A`].dnac[`C`].dnac[`C`].dnat[`T`].dnac[`C`].dnac[`C`].dnac[`C`].dnag[`G`].dnaa[`A`].dnag[`G`].dnac[`C`].dnaa[`A`].dnaa[`A`].dnat[`T`].dnaa[`A`].dnag[`G`].dnat[`T`].dnat[`T`].dnag[`G`].dnat[`T`].dnaa[`A`].dnag[`G`].dnag[`G`].dnac[`C`].dnaa[`A`].dnag[`G`].dnac[`C`].dnag[`G`].dnat[`T`].dnac[`C`].dnac[`C`].dnat[`T`].dnaa[`A`].dnac[`C`].dnac[`C`].dnag[`G`].dnat[`T`].dnat[`T`].dnac[`C`].dnag[`G`]
.dnac[`C`].dnat[`T`].dnaa[`A`].dnac[`C`].dnag[`G`].dnac[`C`].dnag[`G`].dnat[`T`].dnac[`C`].dnat[`T`].dnac[`C`].dnat[`T`].dnat[`T`].dnat[`T`].dnac[`C`].dnag[`G`].dnag[`G`].dnag[`G`].dnag[`G`].dnag[`G`].dnat[`T`].dnat[`T`].dnaa[`A`]
.dnat[`T`].dnat[`T`].dnag[`G`].dnac[`C`].dnaa[`A`].dnag[`G`].dnaa[`A`].dnag[`G`].dnag[`G`].dnat[`T`].dnat[`T`].dnaa[`A`].dnaa[`A`].dnac[`C`].dnag[`G`].dnac[`C`].dnag[`G`].dnac[`C`].dnaa[`A`].dnat[`T`].dnag[`G`].dnac[`C`].dnag[`G`].dnaa[`A`].dnac[`C`].dnat[`T`].dnag[`G`].dnag[`G`].dnag[`G`].dnag[`G`].dnat[`T`].dnat[`T`].dnaa[`A`].dnag[`G`].dnat[`T`].dnaa[`A`].dnag[`G`].dnat[`T`].dnac[`C`].dnag[`G`].dnaa[`A`].dnaa[`A`].dnac[`C`].dnaa[`A`].dnat[`T`].dnaa[`A`].dnat[`T`].dnaa[`A`].dnat[`T`].dnat[`T`].dnag[`G`].dnaa[`A`].dnat[`T`].dnaa[`A`].dnaa[`A`].dnaa[`A`].dnac[`C`].dnaa[`A`]
.dnag[`G`].dnac[`C`].dnat[`T`].dnac[`C`].dnag[`G`].dnac[`C`].dnat[`T`].dnat[`T`].dnaa[`A`].dnag[`G`].dnag[`G`].dnag[`G`].dnac[`C`].dnac[`C`].dnat[`T`].dnac[`C`].dnag[`G`].dnaa[`A`].dnac[`C`].dnat[`T`].dnac[`C`].dnac[`C`].dnat[`T`].dnac[`C`].dnat[`T`].dnag[`G`].dnaa[`A`].dnaa[`A`].dnat[`T`].dnag[`G`].dnag[`G`].dnaa[`A`].dnag[`G`].dnat[`T`].dnag[`G`].dnat[`T`].dnat[`T`].dnac[`C`].dnaa[`A`].dnat[`T`].dnac[`C`].dnag[`G`].dnaa[`A`].dnaa[`A`].dnat[`T`].dnag[`G`].dnag[`G`].dnaa[`A`].dnag[`G`].dnat[`T`].dnag[`G`]
] --- count: false ## Why Tetris for scientific discovery? ### Molecular generation .context35[The "Tetris problem" involves sampling from an unknown distribution in a discrete, high-dimensional, combinatorially large space]
Small molecules can also be represented as sequences or by a combination of of higher-level fragments. There may be about $10^{60}$ drug-like molecules. .columns-3-left[ .center[ 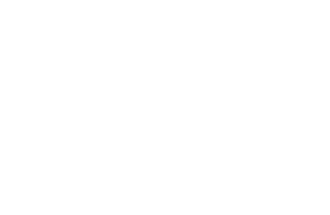 `CC(=O)NCCC1=CNc2c1cc(OC)cc2 CC(=O)NCCc1c[nH]c2ccc(OC)cc12` ]] .columns-3-center[ .center[ 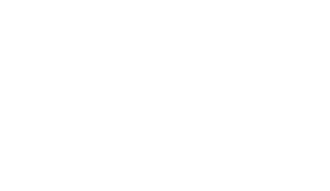 `OCCc1c(C)[n+](cs1)Cc2cnc(C)nc2N` ]] .columns-3-right[ .center[ 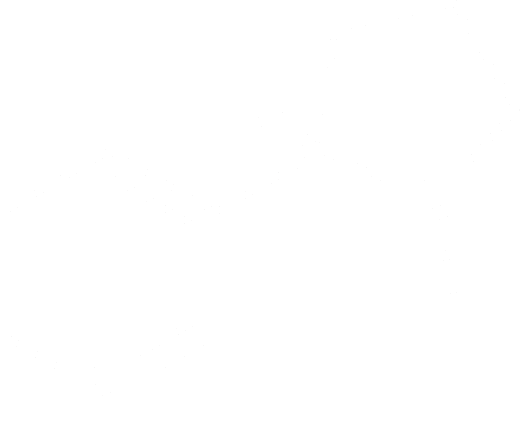 `CN1CCC[C@H]1c2cccnc2` ]] --- count: false ## Why Tetris for scientific discovery? ### Crystal structure generation .context35[The "Tetris problem" involves sampling from an unknown distribution in a discrete, high-dimensional, combinatorially large space]
Crystal structures can be described by their chemical composition, the symmetry group and the lattice parameters (and more generally by atomic positions). .left-column[ .center[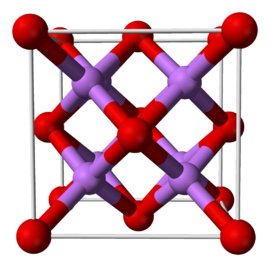] ] .right-column[ .center[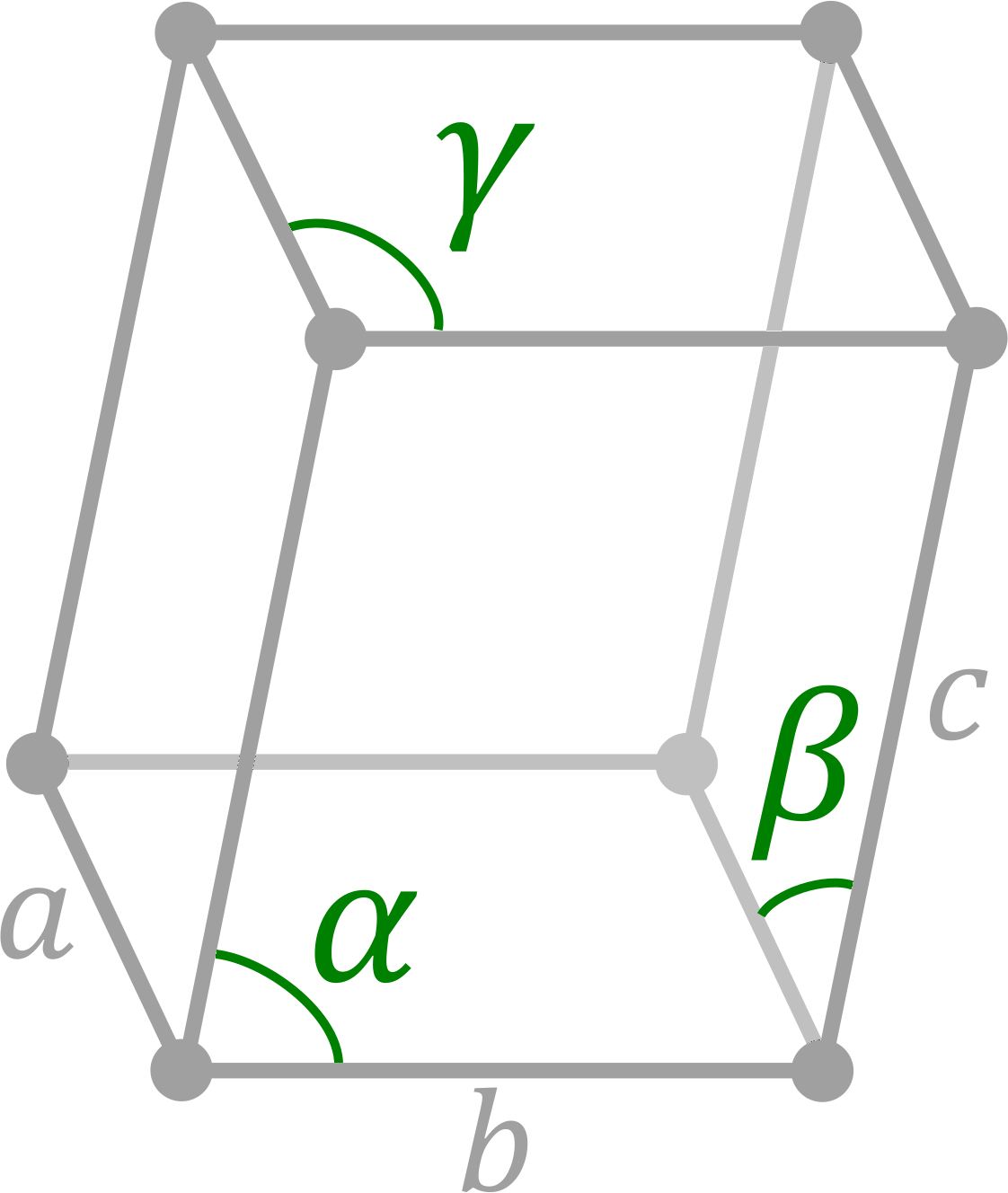] ] .references[ * Mila AI4Science et al. [Crystal-GFN: sampling crystals with desirable properties and constraints](https://arxiv.org/abs/2310.04925). AI4Mat, NeurIPS 2023 (spotlight). ] --- count: false name: gflownets class: title, middle ### A gentle introduction to Generative Flow Networks (GFlowNets) .center[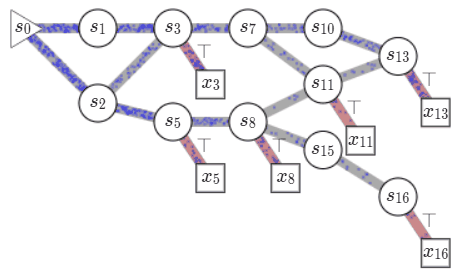] --- count: false name: gflownets class: title, middle ### A brief ~~gentle~~ introduction to Generative Flow Networks (GFlowNets) .center[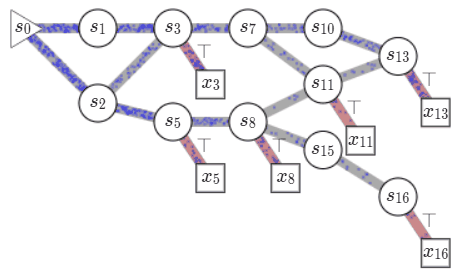] --- count: false name: gflownets class: title, middle ### A lightning ~~brief gentle~~ introduction to Generative Flow Networks (GFlowNets) .center[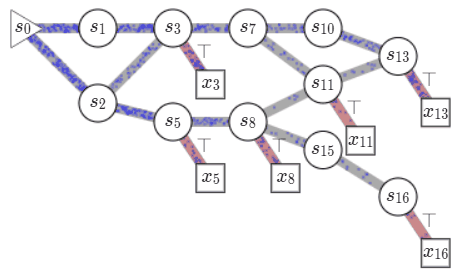] --- count: false ## GFlowNets for science ### 3 key ingredients .context[Materials and drug discovery involve .highlight1[sampling from unknown distributions] in .highlight1[discrete or mixed, high-dimensional, combinatorially large spaces.]] --
1. .highlight1[Diversity] as an objective. -- - Given a score or reward function $R(x)$, learn to _sample proportionally to the reward_. -- 2. .highlight1[Compositionality] in the sample generation. -- - A meaningful decomposition of samples $x$ into multiple sub-states $s_0\rightarrow s_1 \rightarrow \dots \rightarrow x$ can yield generalisable patterns. -- 3. .highlight1[Deep learning] to learn from the generated samples. -- - A machine learning model can learn the transition function $F(s\rightarrow s')$ and generalise the patterns. -- .references[ - [Skip to multi-fidelity active learning](#mfal) ] --- count: false ## 1. Diversity as an objective .context[Many existing approaches treat scientific discovery as an _optimisation_ problem.]
Given a reward or objective function $R(x)$, GFlowNet can be seen a generative model trained to sample objects $x \in \cal X$ according to .highlight1[a sampling policy $\pi(x)$ proportional to the reward $R(x)$]: -- .left-column[ $$\pi(x) = \frac{R(x)}{Z} \propto R(x)$$ ] -- .right-column[ $$Z = \sum_{x' \in \cal X} R(x')$$ ] -- .full-width[ .center[ 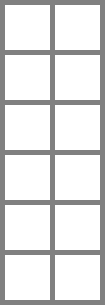 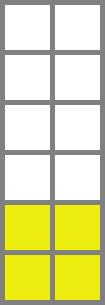 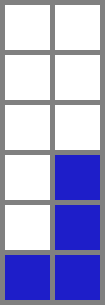 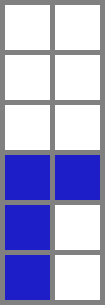 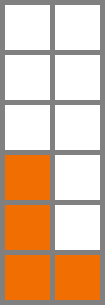 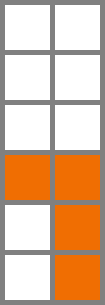 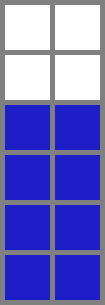 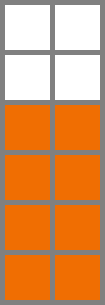 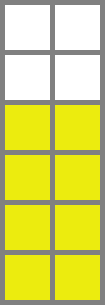 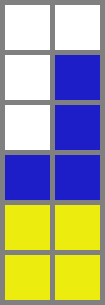 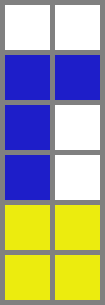 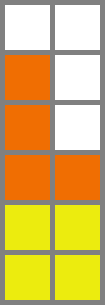 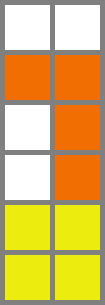 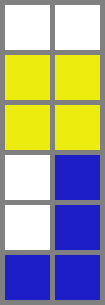 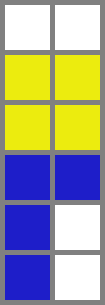 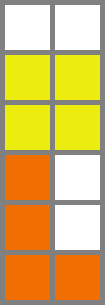 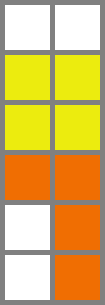 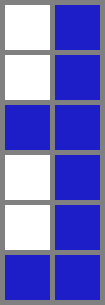 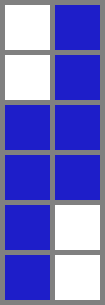 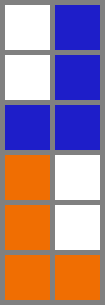 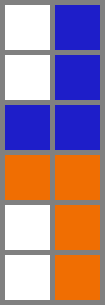 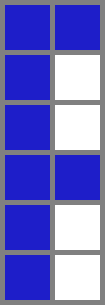 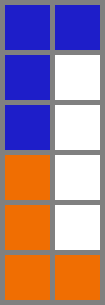 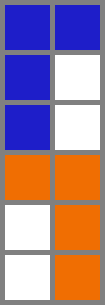 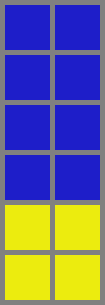 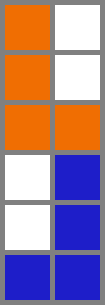 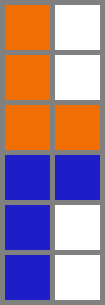 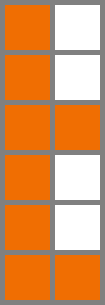 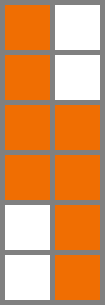 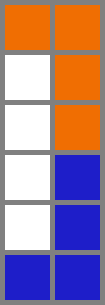 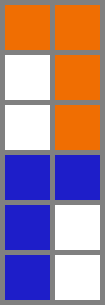 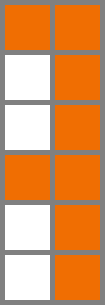 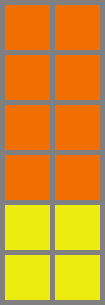 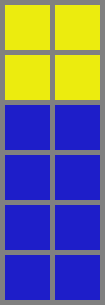 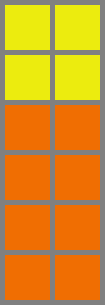 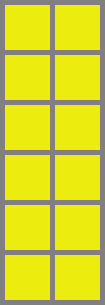 ]] --- count: false ## 1. Diversity as an objective .context[Many existing approaches treat scientific discovery as an _optimisation_ problem.]
Given a reward or objective function $R(x)$, GFlowNet can be seen a generative model trained to sample objects $x \in \cal X$ according to .highlight1[a sampling policy $\pi(x)$ proportional to the reward $R(x)$]: .left-column[ $$\pi(x) = \frac{R(x)}{Z} \propto R(x)$$ ] .right-column[ $$Z = \sum_{x' \in \cal X} R(x')$$ ] .full-width[ → Sampling proportionally to the reward function enables finding .highlight1[multiple modes], hence .highlight1[diversity]. .center[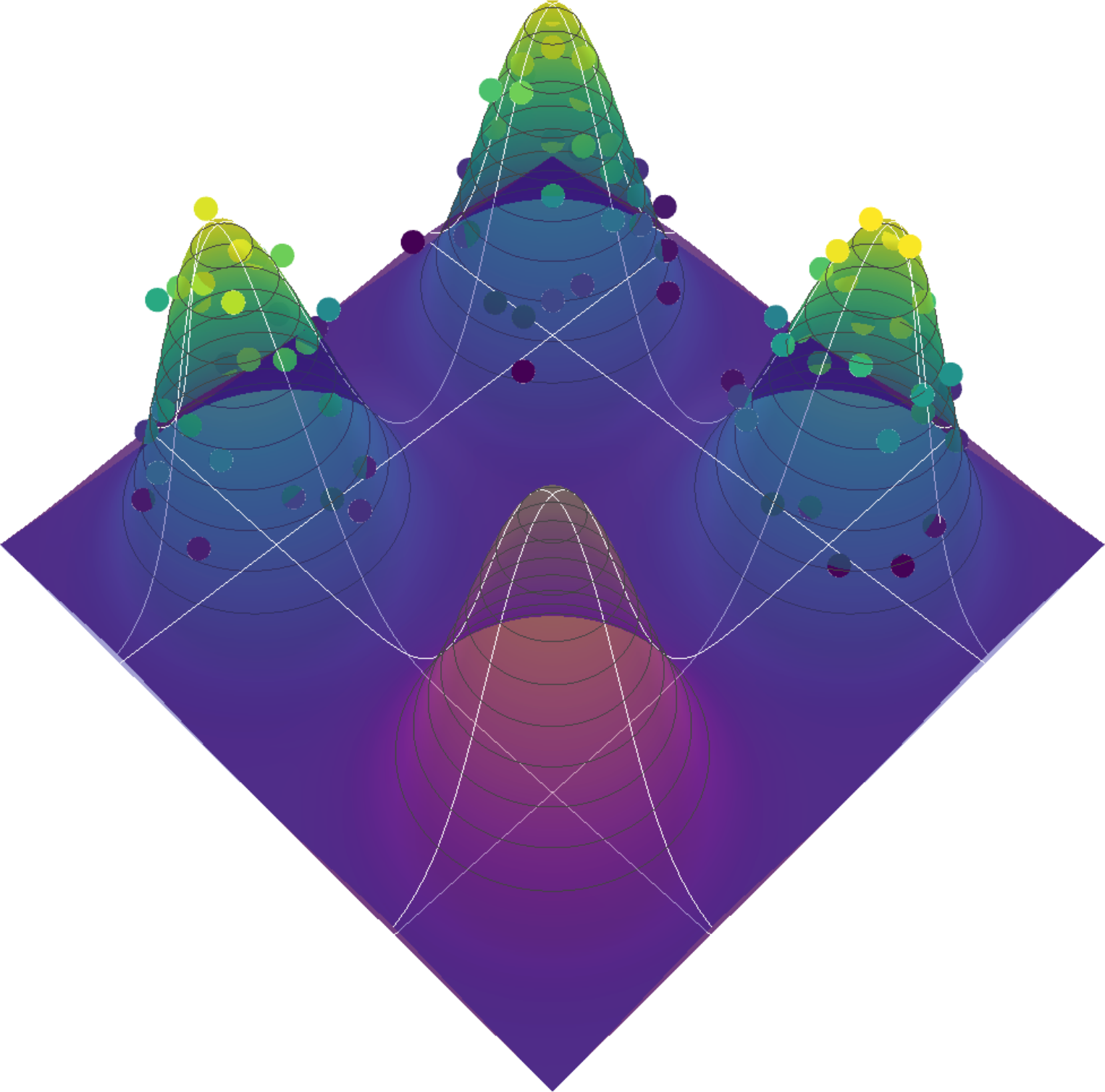] ] --- count: false ## 2. Compositionality ### Sample generation process .context35[Sampling _directly_ from a complex, high-dimensional distribution is difficult.] The principle of compositionality is fundamental in semantics, linguistics, mathematical logic and is thought to be a cornerstone of human reasoning. --- count: false ## 2. Compositionality ### Sample generation process .context35[Sampling _directly_ from a complex, high-dimensional distribution is difficult.] For the Tetris problem, a meaningful decomposition of the samples is .highlight1[adding one piece to the board at a time]. -- .left-column[.center[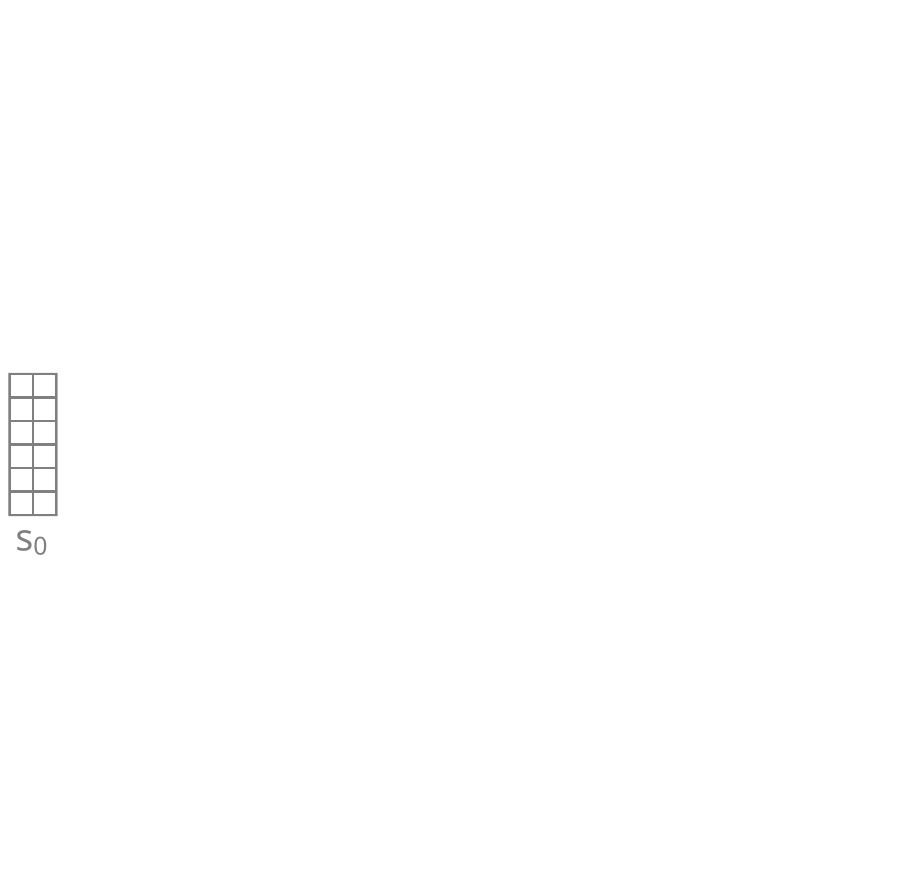]] --- count: false ## 2. Compositionality ### Sample generation process .context35[Sampling _directly_ from a complex, high-dimensional distribution is difficult.] For the Tetris problem, a meaningful decomposition of the samples is .highlight1[adding one piece to the board at a time]. .left-column[.center[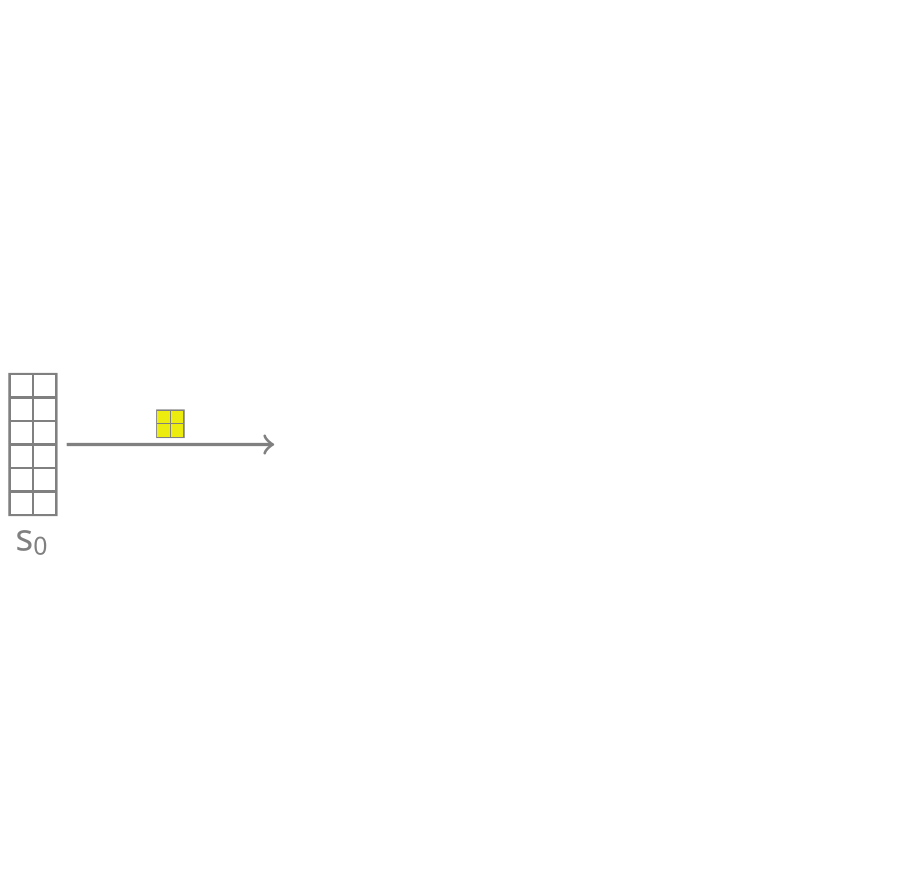]] --- count: false ## 2. Compositionality ### Sample generation process .context35[Sampling _directly_ from a complex, high-dimensional distribution is difficult.] For the Tetris problem, a meaningful decomposition of the samples is .highlight1[adding one piece to the board at a time]. .left-column[.center[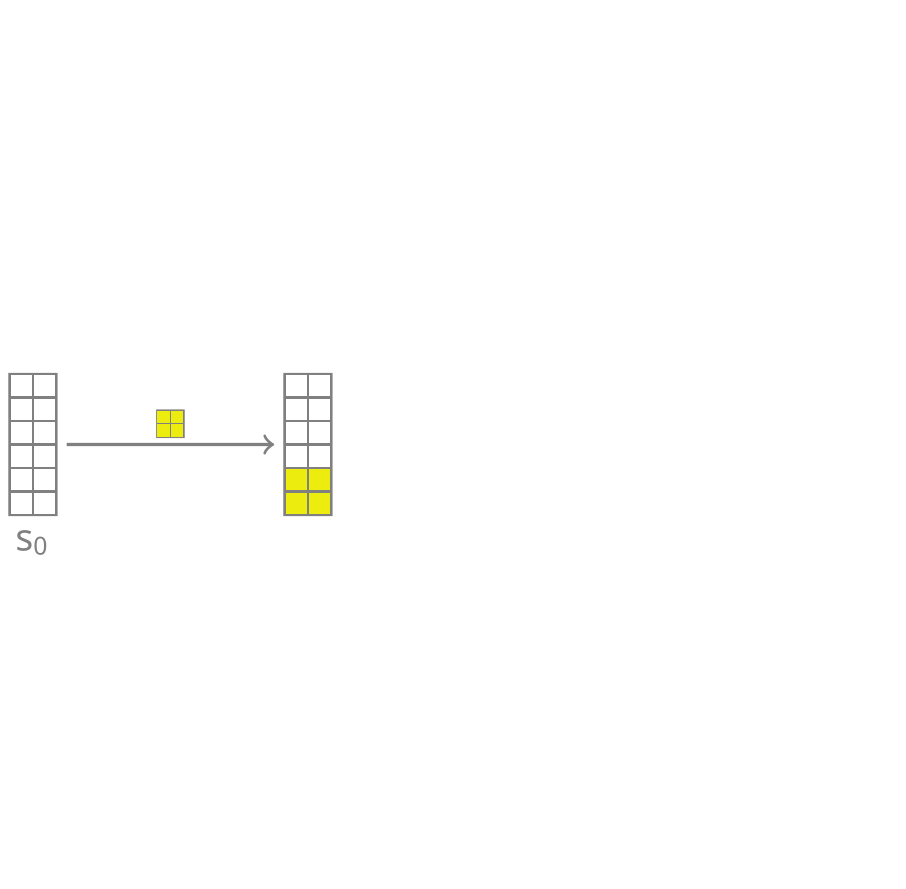]] --- count: false ## 2. Compositionality ### Sample generation process .context35[Sampling _directly_ from a complex, high-dimensional distribution is difficult.] For the Tetris problem, a meaningful decomposition of the samples is .highlight1[adding one piece to the board at a time]. .left-column[.center[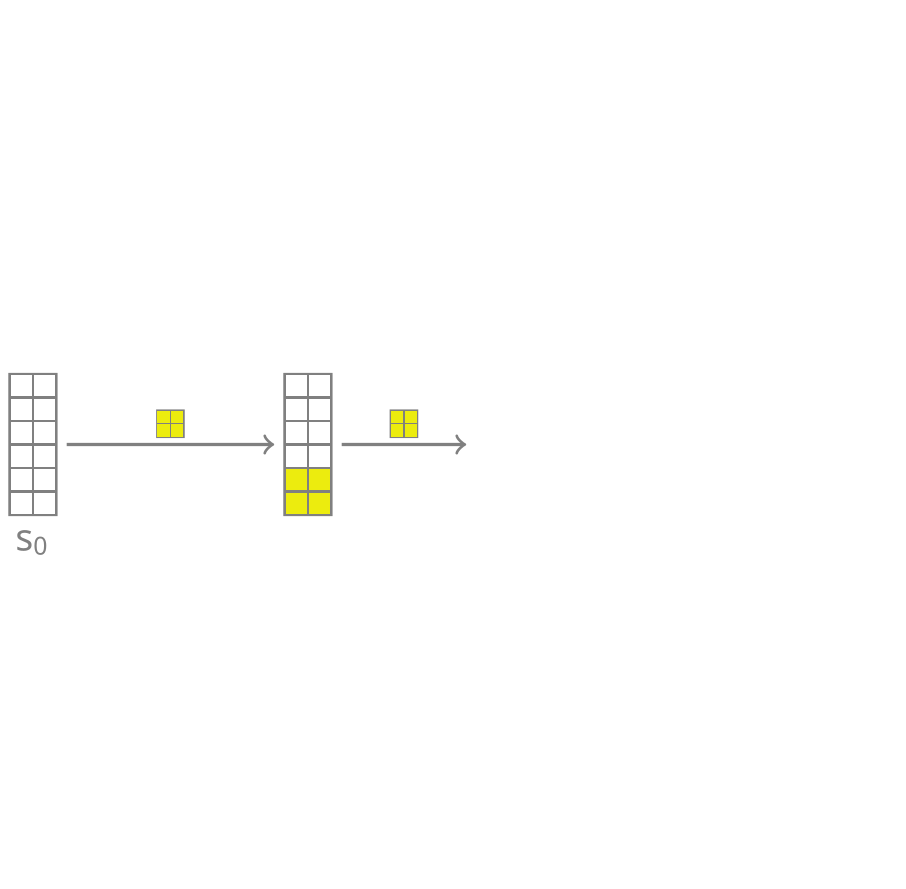]] --- count: false ## 2. Compositionality ### Sample generation process .context35[Sampling _directly_ from a complex, high-dimensional distribution is difficult.] For the Tetris problem, a meaningful decomposition of the samples is .highlight1[adding one piece to the board at a time]. .left-column[.center[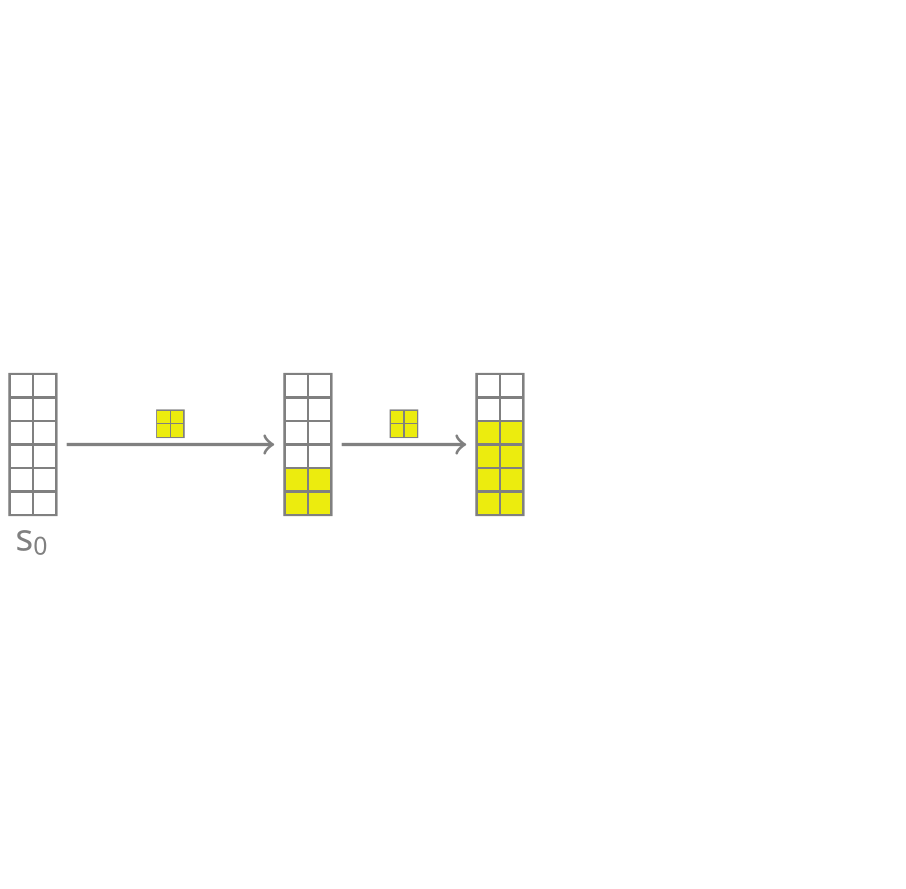]] --- count: false ## 2. Compositionality ### Sample generation process .context35[Sampling _directly_ from a complex, high-dimensional distribution is difficult.] For the Tetris problem, a meaningful decomposition of the samples is .highlight1[adding one piece to the board at a time]. .left-column[.center[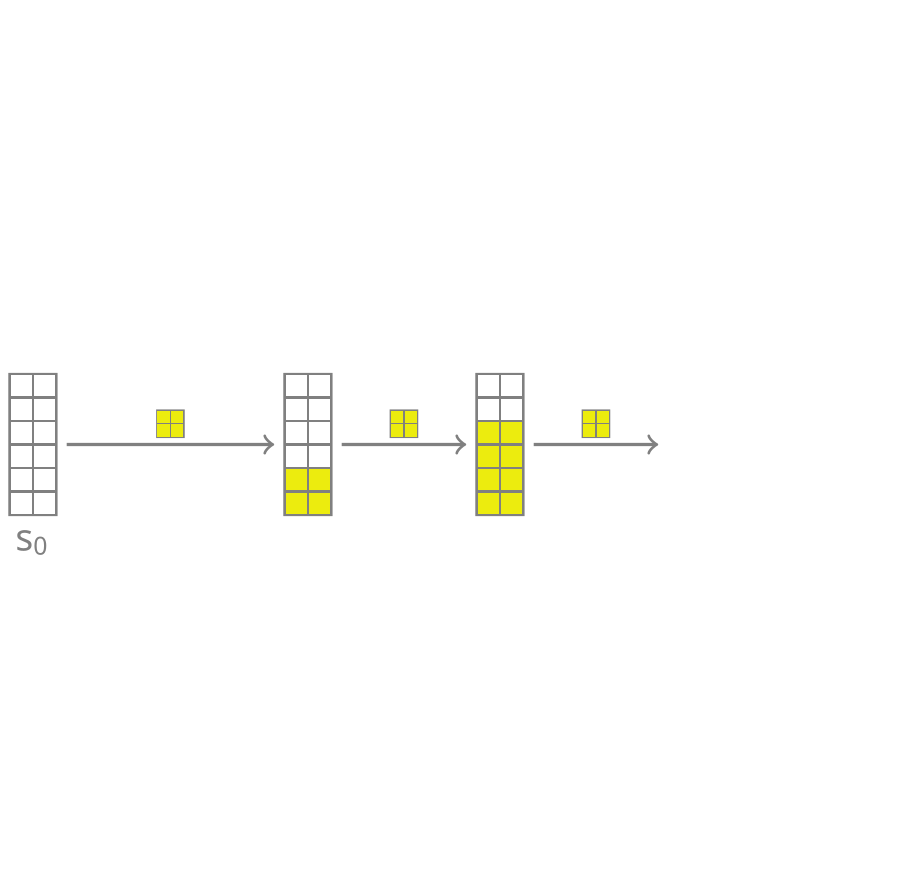]] --- count: false ## 2. Compositionality ### Sample generation process .context35[Sampling _directly_ from a complex, high-dimensional distribution is difficult.] For the Tetris problem, a meaningful decomposition of the samples is .highlight1[adding one piece to the board at a time]. .left-column[.center[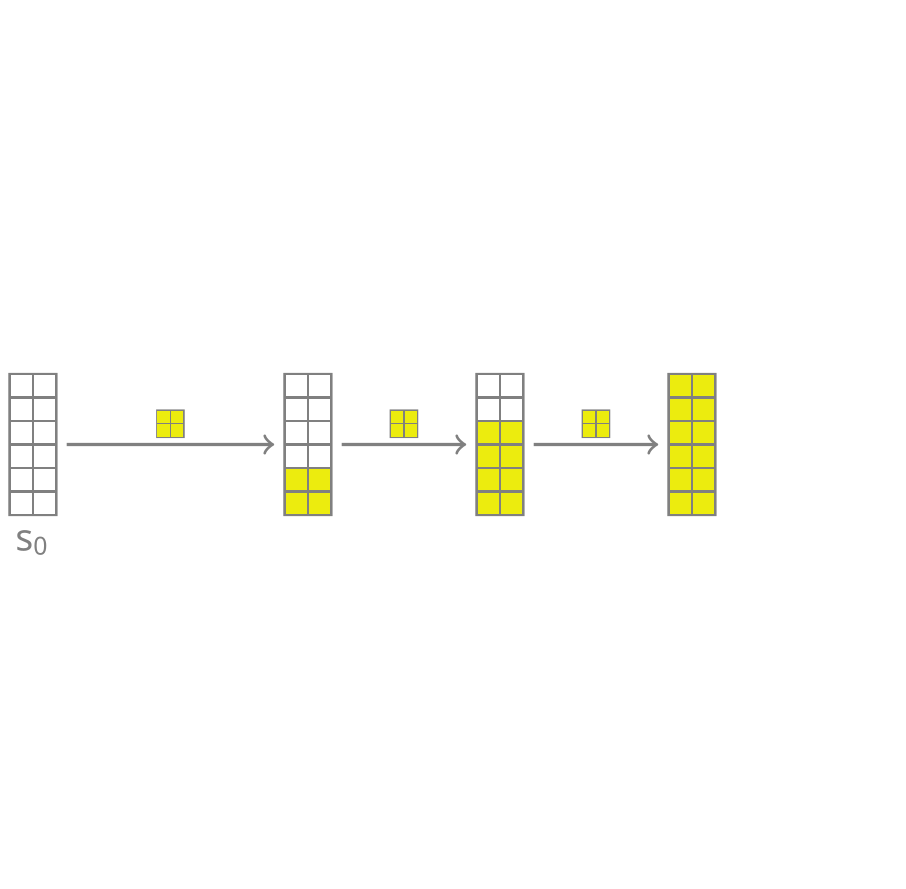]] --- count: false ## 2. Compositionality ### Sample generation process .context35[Sampling _directly_ from a complex, high-dimensional distribution is difficult.] For the Tetris problem, a meaningful decomposition of the samples is .highlight1[adding one piece to the board at a time]. .left-column[.center[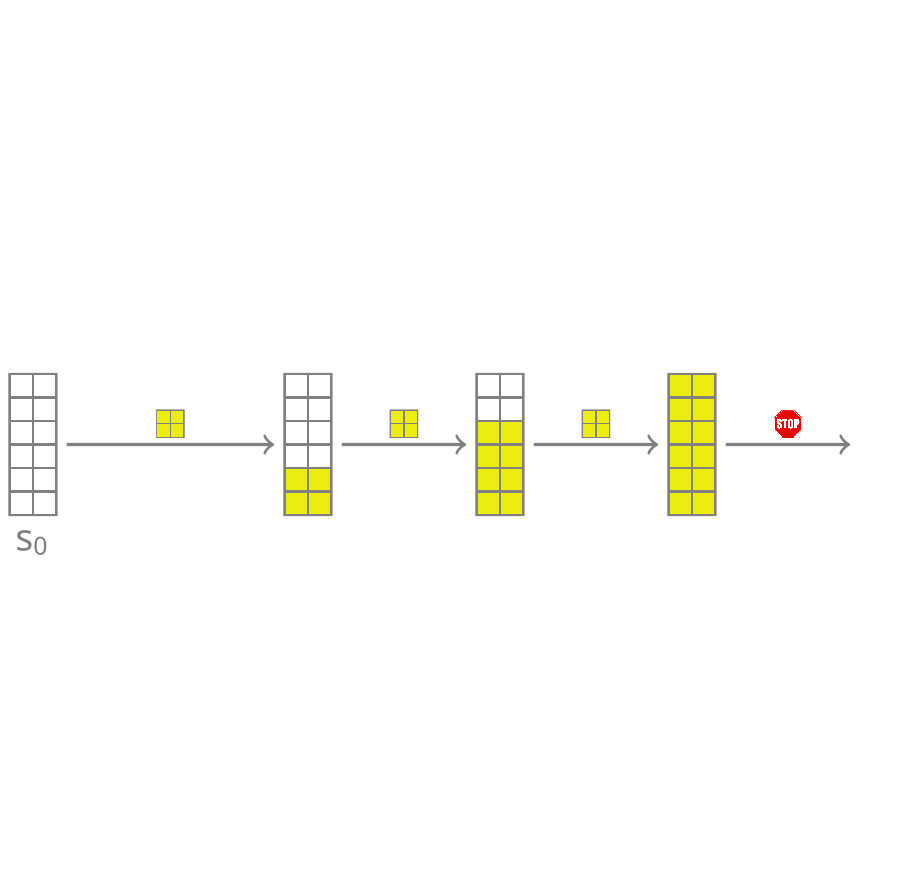]] --- count: false ## 2. Compositionality ### Sample generation process .context35[Sampling _directly_ from a complex, high-dimensional distribution is difficult.] For the Tetris problem, a meaningful decomposition of the samples is .highlight1[adding one piece to the board at a time]. .left-column[.center[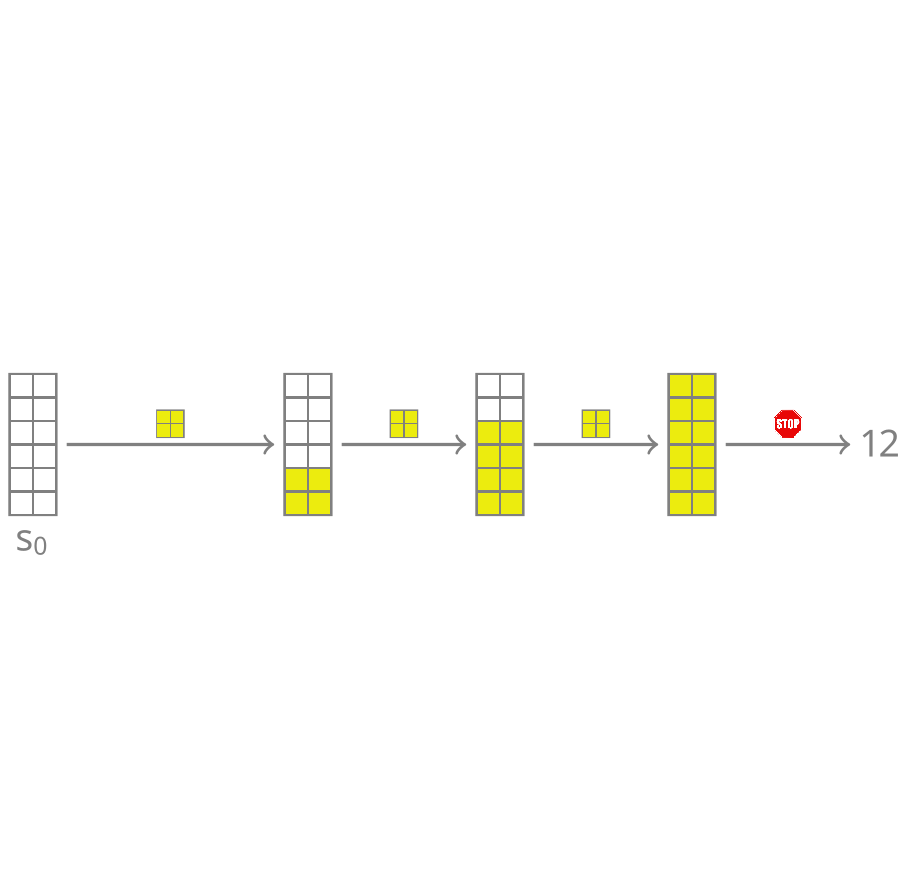]] --- count: false ## 2. Compositionality ### Sample generation process .context35[Sampling _directly_ from a complex, high-dimensional distribution is difficult.] For the Tetris problem, a meaningful decomposition of the samples is .highlight1[adding one piece to the board at a time]. .left-column[.center[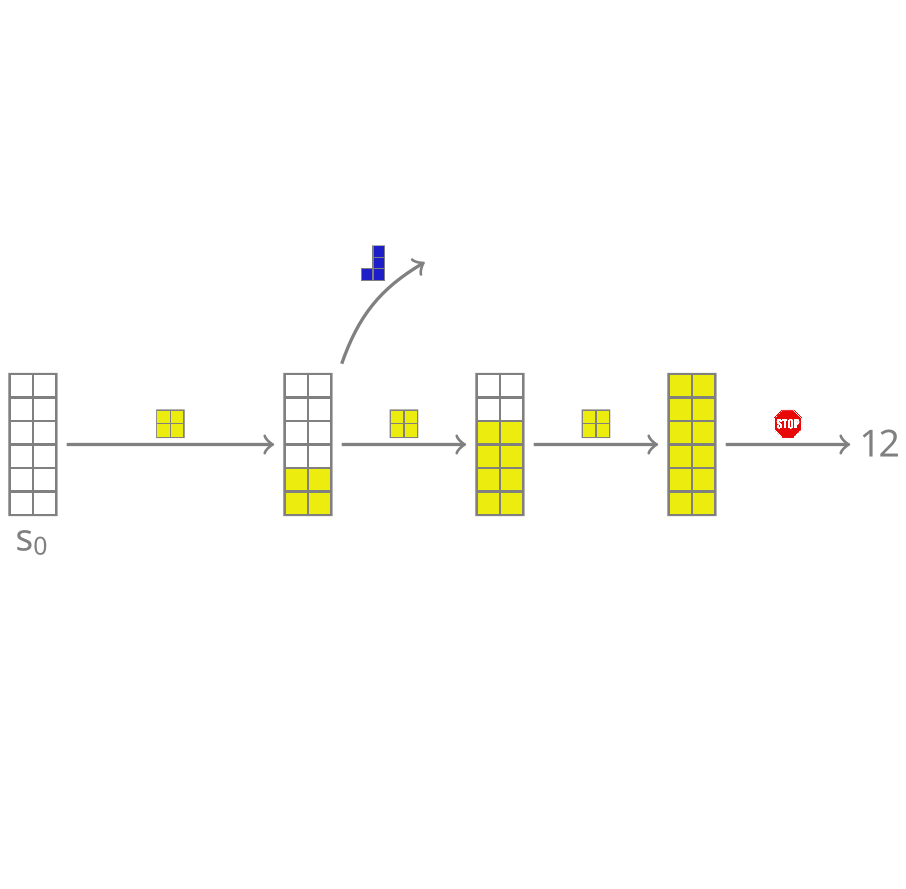]] --- count: false ## 2. Compositionality ### Sample generation process .context35[Sampling _directly_ from a complex, high-dimensional distribution is difficult.] For the Tetris problem, a meaningful decomposition of the samples is .highlight1[adding one piece to the board at a time]. .left-column[.center[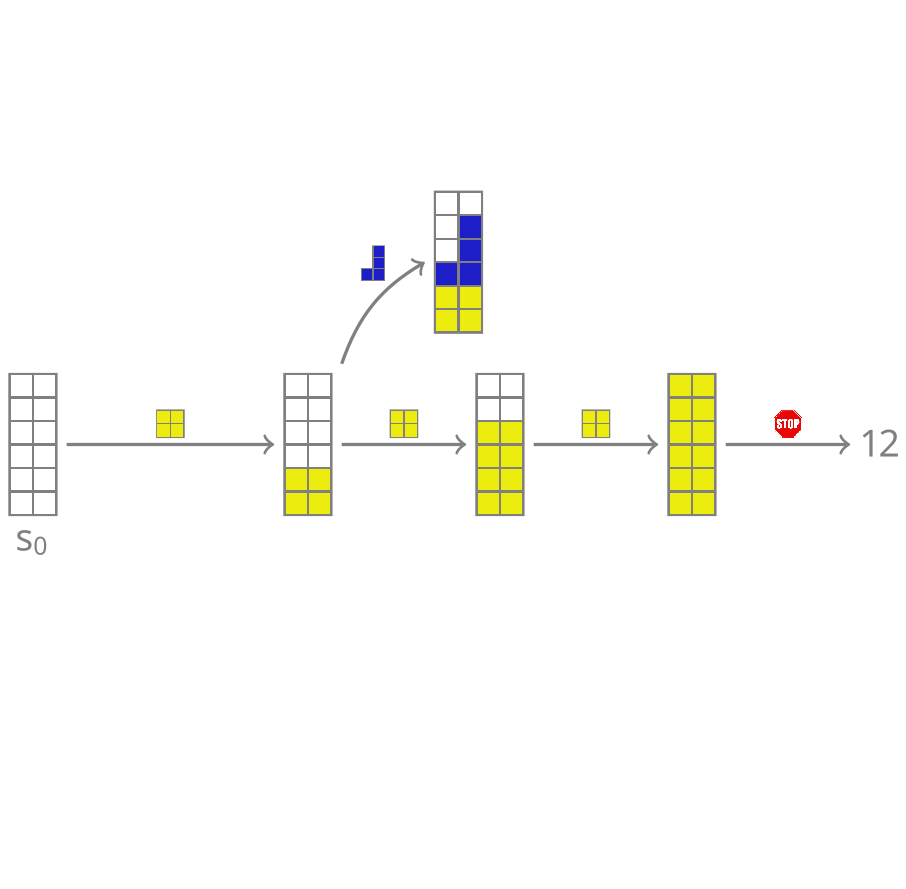]] --- count: false ## 2. Compositionality ### Sample generation process .context35[Sampling _directly_ from a complex, high-dimensional distribution is difficult.] For the Tetris problem, a meaningful decomposition of the samples is .highlight1[adding one piece to the board at a time]. .left-column[.center[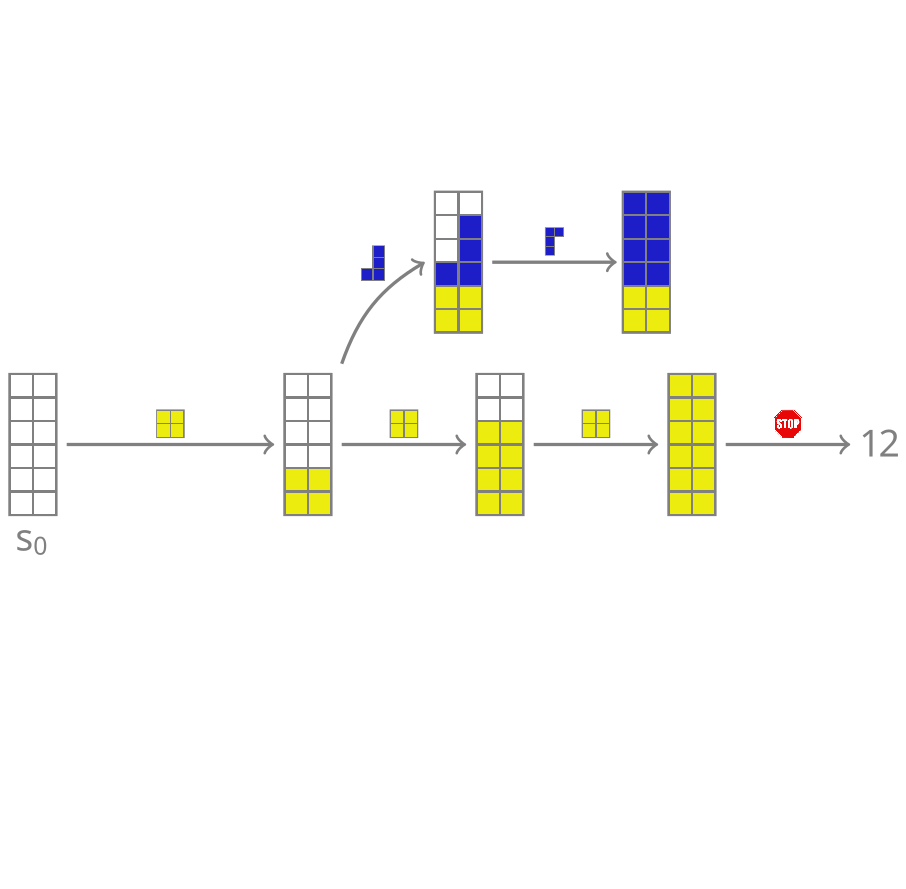]] --- count: false ## 2. Compositionality ### Sample generation process .context35[Sampling _directly_ from a complex, high-dimensional distribution is difficult.] For the Tetris problem, a meaningful decomposition of the samples is .highlight1[adding one piece to the board at a time]. .left-column[.center[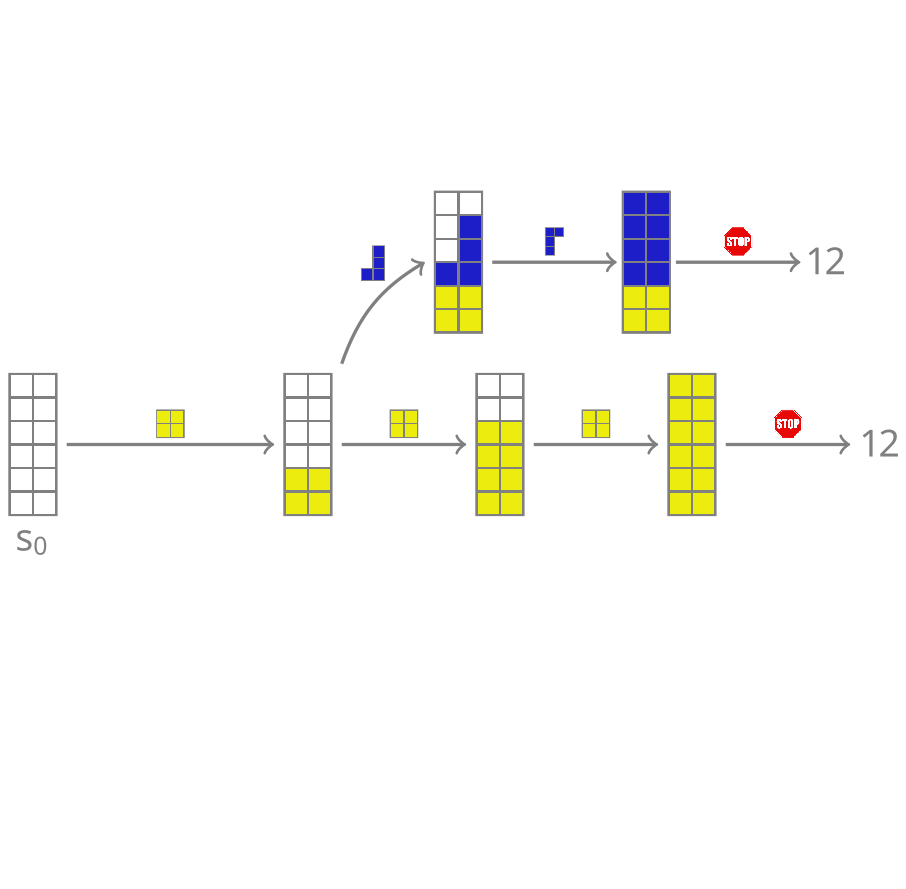]] --- count: false ## 2. Compositionality ### Sample generation process .context35[Sampling _directly_ from a complex, high-dimensional distribution is difficult.] For the Tetris problem, a meaningful decomposition of the samples is .highlight1[adding one piece to the board at a time]. .left-column[.center[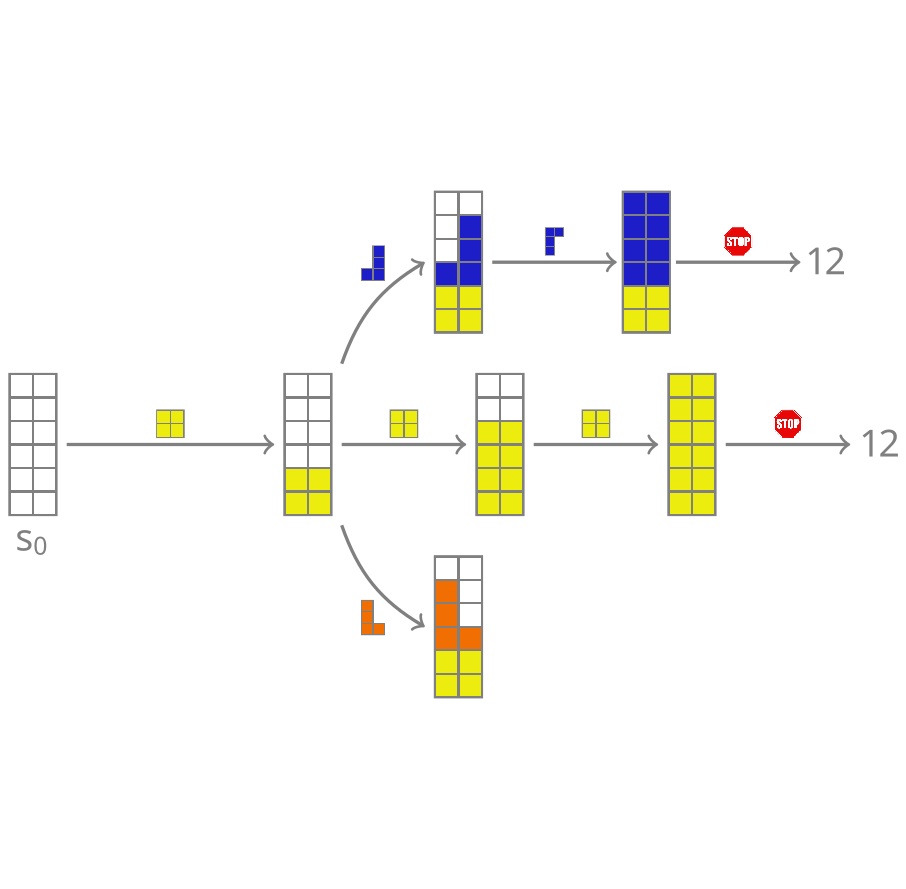]] --- count: false ## 2. Compositionality ### Sample generation process .context35[Sampling _directly_ from a complex, high-dimensional distribution is difficult.] For the Tetris problem, a meaningful decomposition of the samples is .highlight1[adding one piece to the board at a time]. .left-column[.center[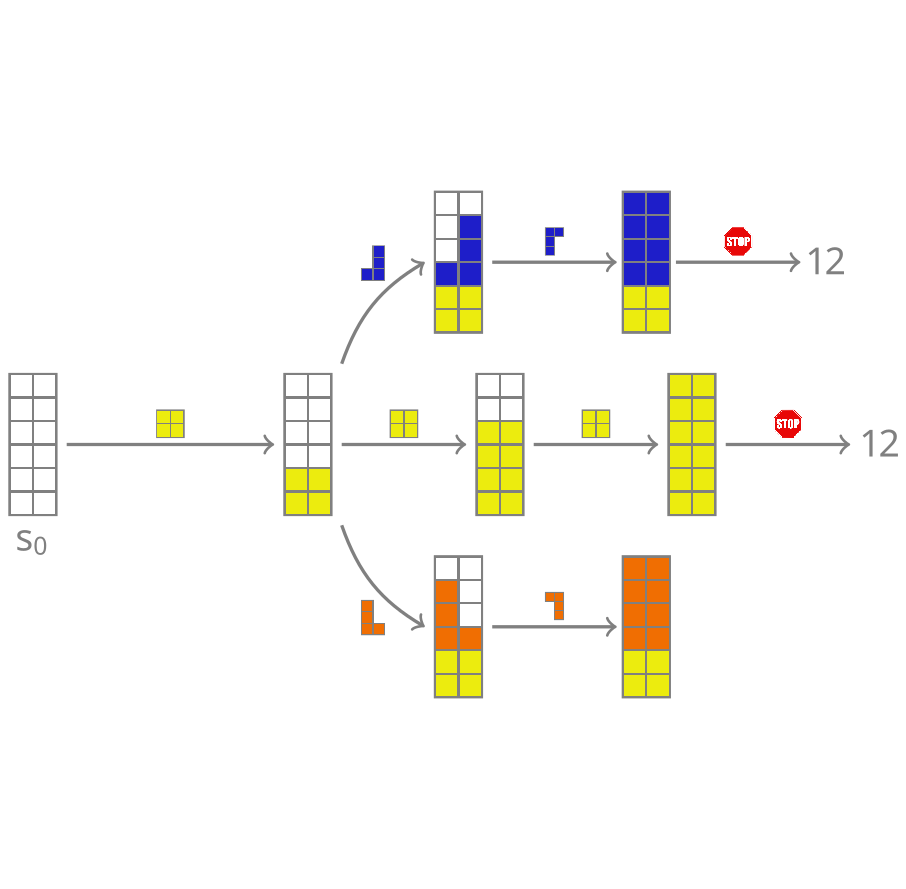]] --- count: false ## 2. Compositionality ### Sample generation process .context35[Sampling _directly_ from a complex, high-dimensional distribution is difficult.] For the Tetris problem, a meaningful decomposition of the samples is .highlight1[adding one piece to the board at a time]. .left-column[.center[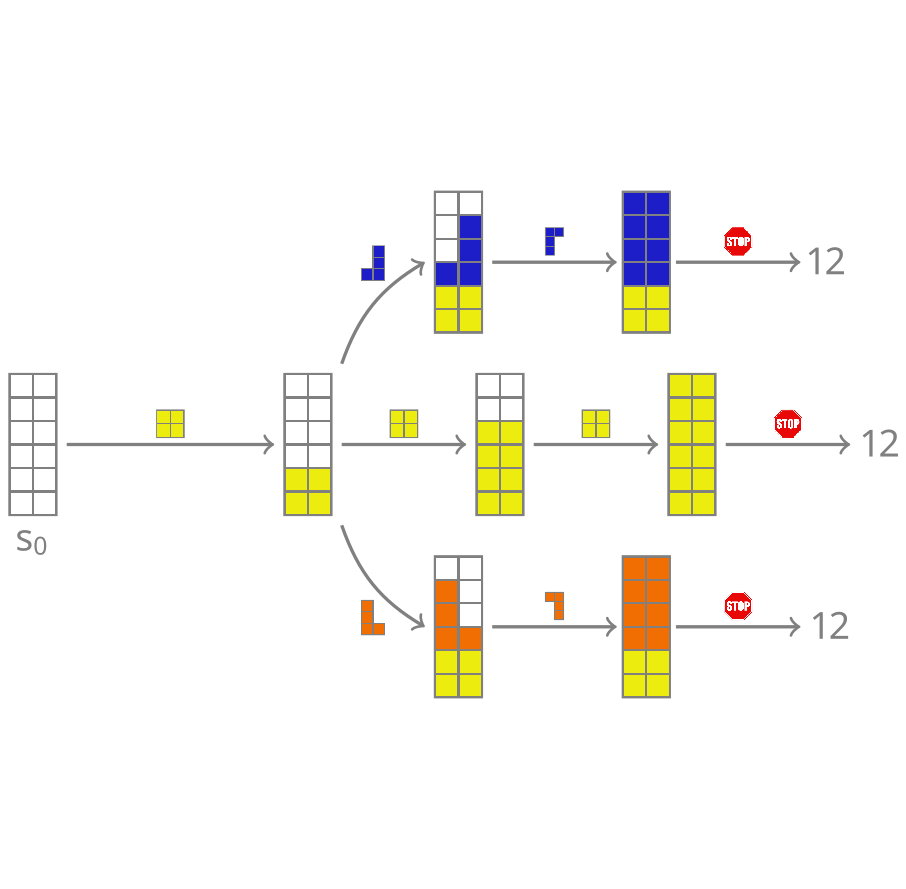]] --- count: false ## 2. Compositionality ### Sample generation process .context35[Sampling _directly_ from a complex, high-dimensional distribution is difficult.] For the Tetris problem, a meaningful decomposition of the samples is .highlight1[adding one piece to the board at a time]. .left-column[.center[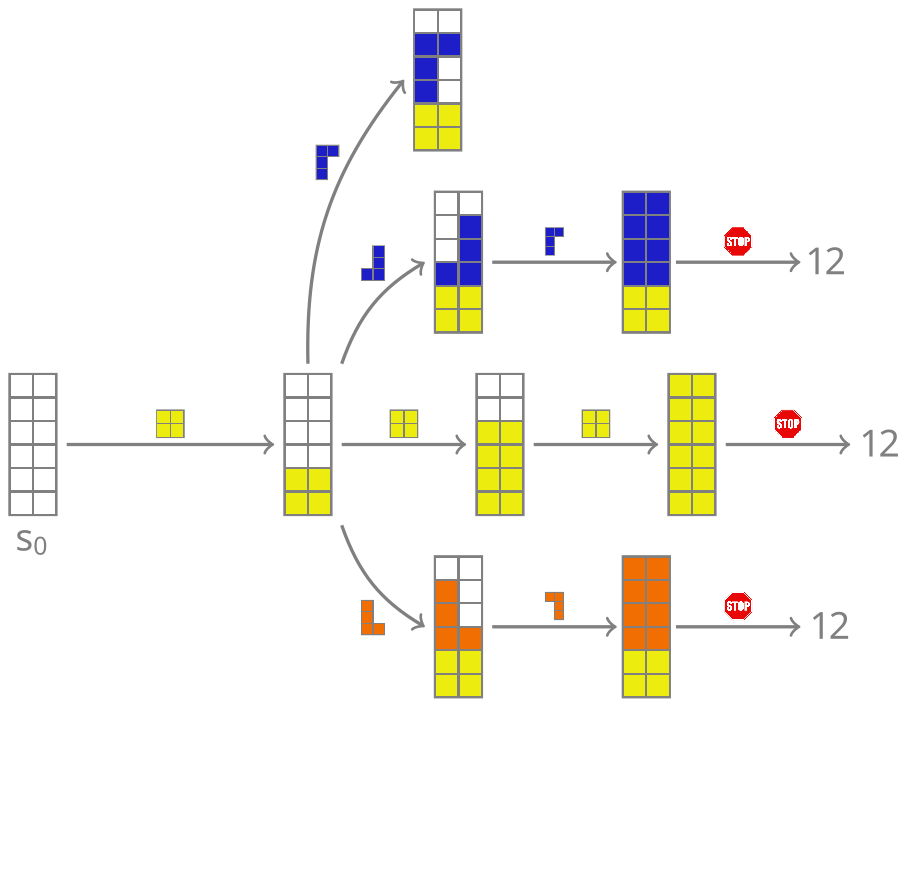]] --- count: false ## 2. Compositionality ### Sample generation process .context35[Sampling _directly_ from a complex, high-dimensional distribution is difficult.] For the Tetris problem, a meaningful decomposition of the samples is .highlight1[adding one piece to the board at a time]. .left-column[.center[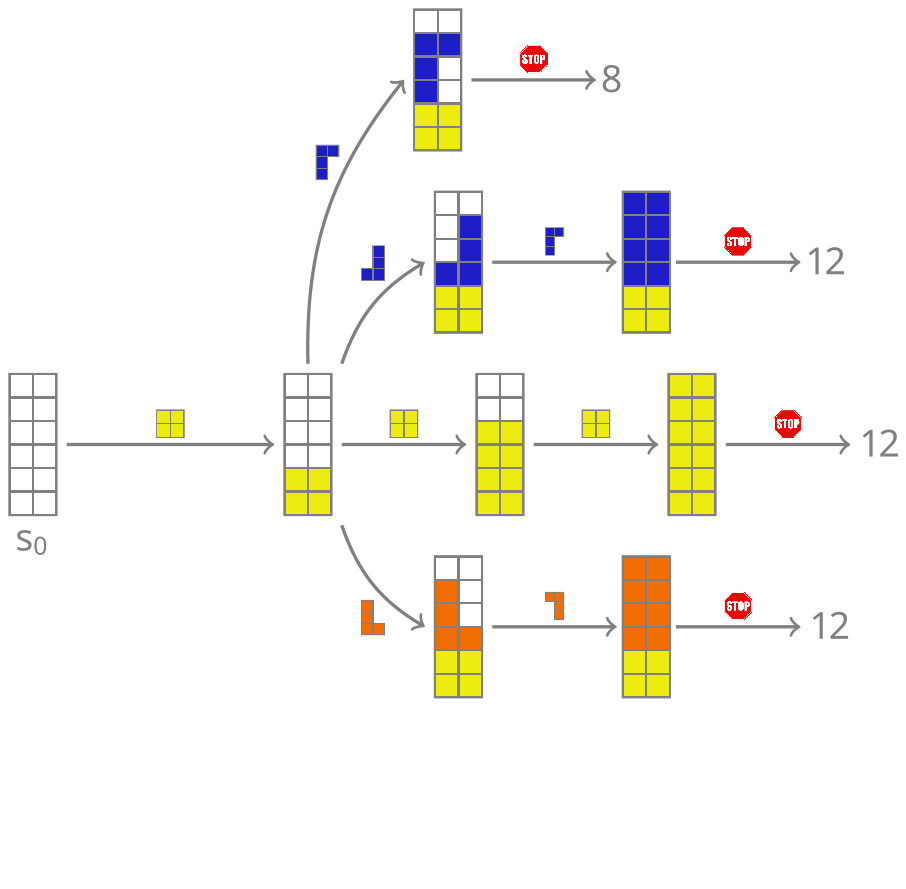]] --- count: false ## 2. Compositionality ### Sample generation process .context35[Sampling _directly_ from a complex, high-dimensional distribution is difficult.] For the Tetris problem, a meaningful decomposition of the samples is .highlight1[adding one piece to the board at a time]. .left-column[.center[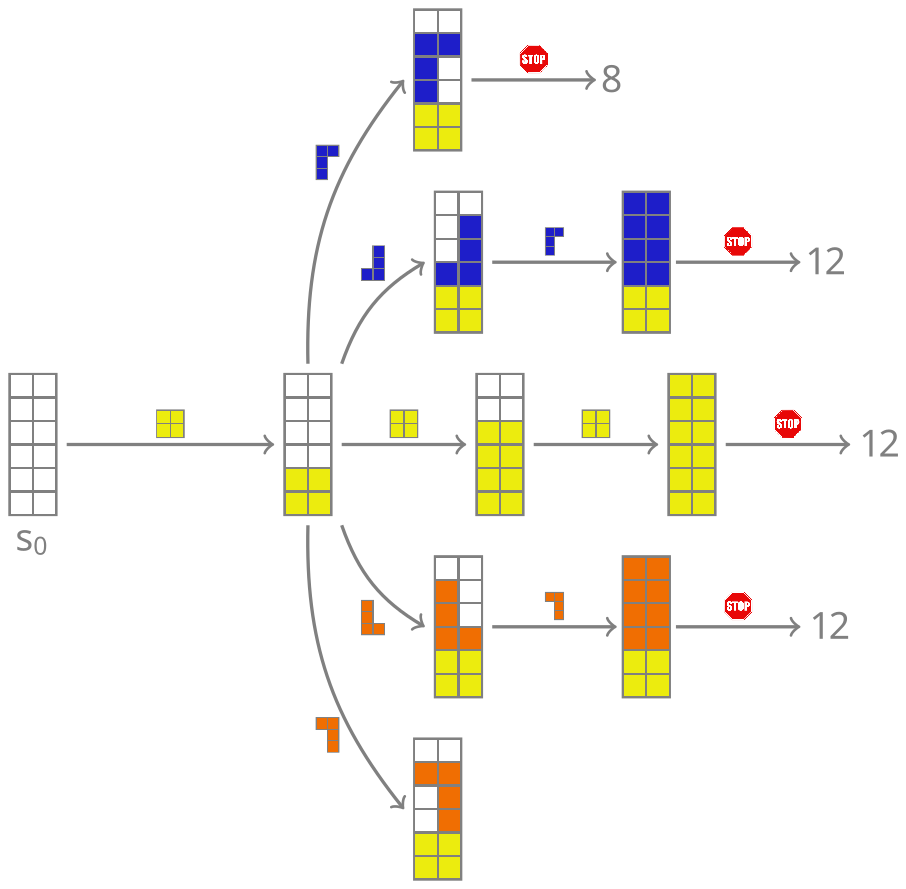]] --- count: false ## 2. Compositionality ### Sample generation process .context35[Sampling _directly_ from a complex, high-dimensional distribution is difficult.] For the Tetris problem, a meaningful decomposition of the samples is .highlight1[adding one piece to the board at a time]. .left-column[.center[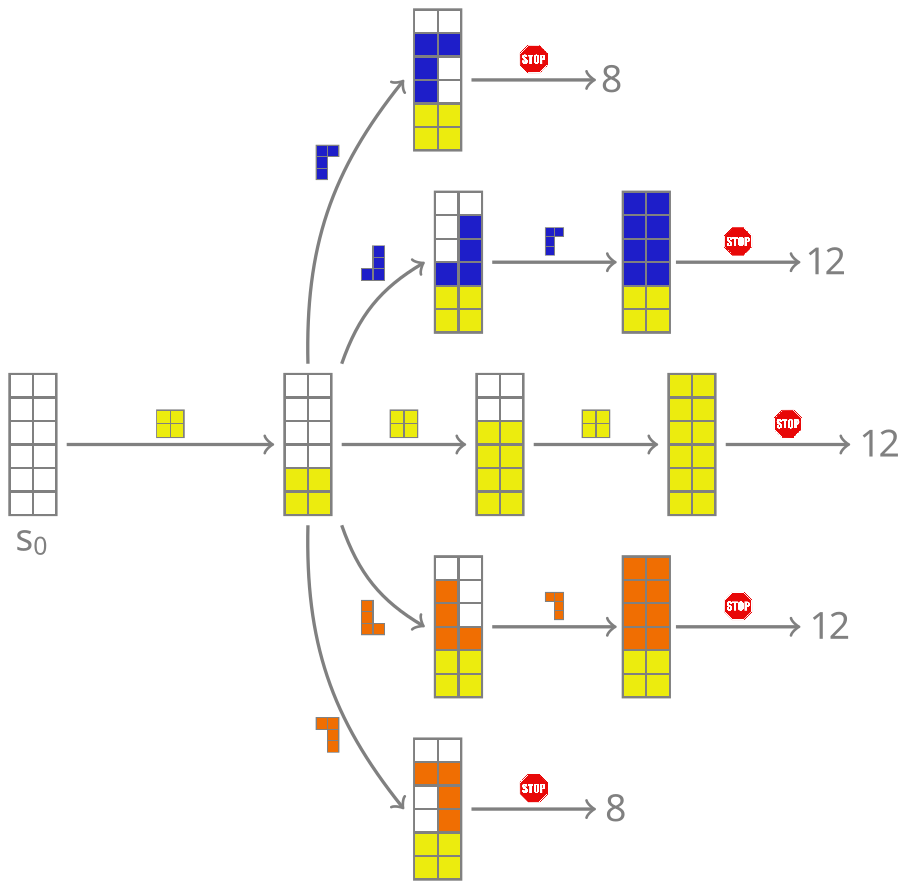]] --- count: false ## 2. Compositionality ### Sample generation process .context35[Sampling _directly_ from a complex, high-dimensional distribution is difficult.] For the Tetris problem, a meaningful decomposition of the samples is .highlight1[adding one piece to the board at a time]. .left-column[.center[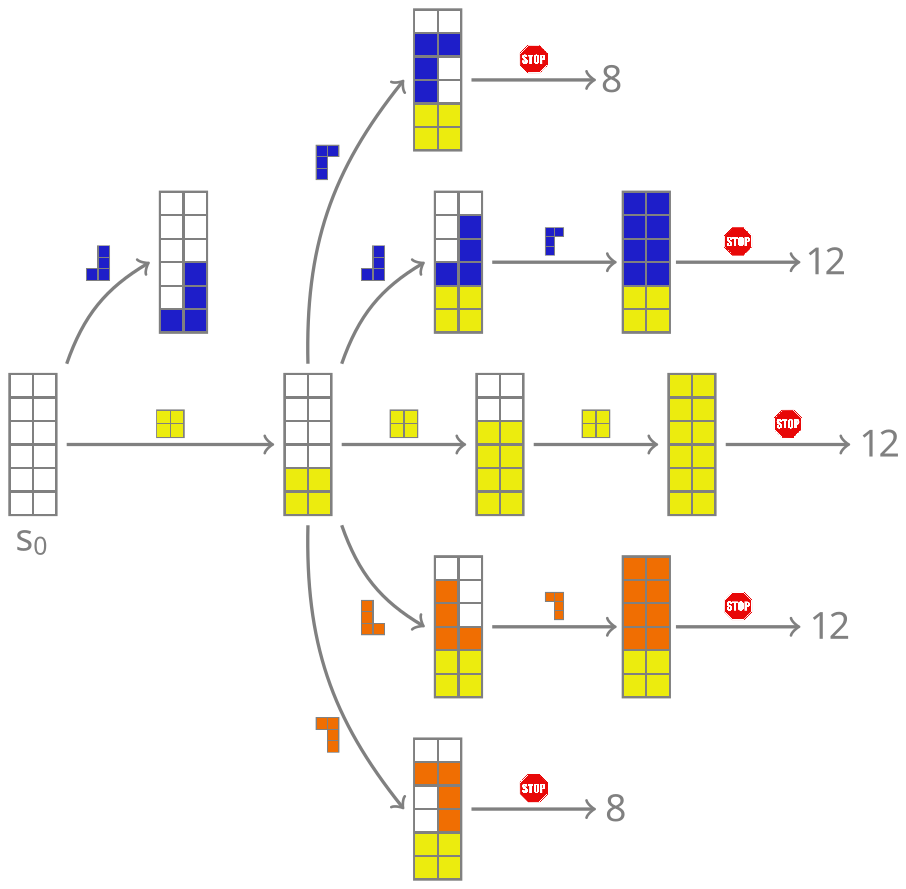]] --- count: false ## 2. Compositionality ### Sample generation process .context35[Sampling _directly_ from a complex, high-dimensional distribution is difficult.] For the Tetris problem, a meaningful decomposition of the samples is .highlight1[adding one piece to the board at a time]. .left-column[.center[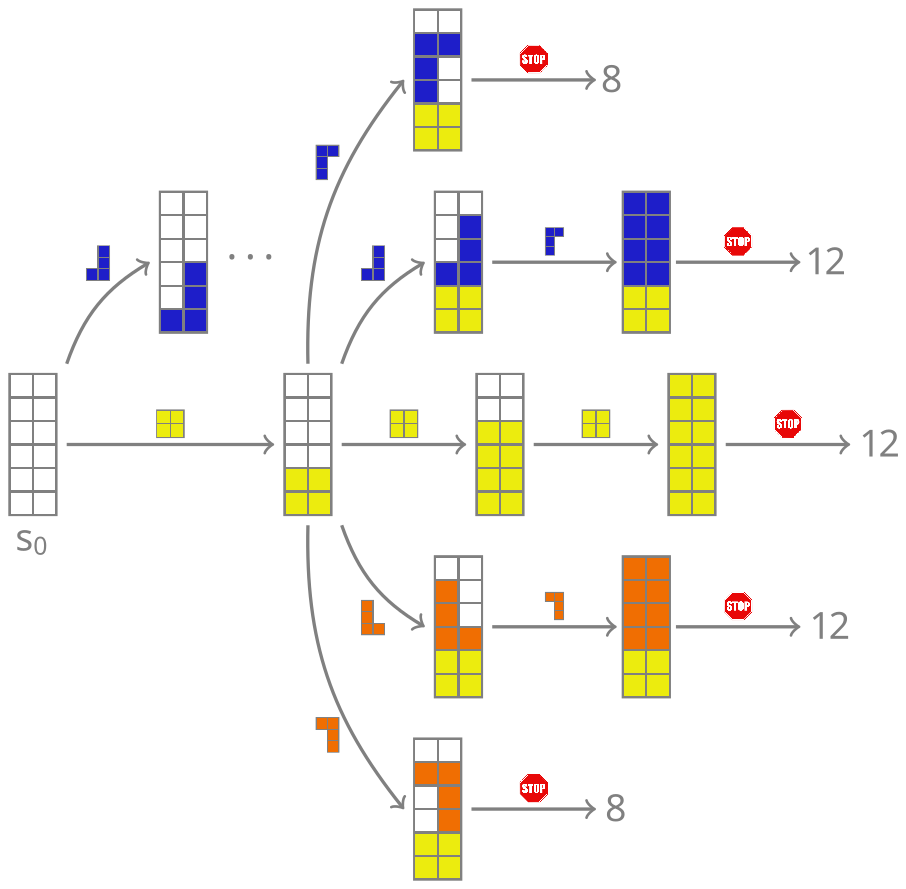]] --- count: false ## 2. Compositionality ### Sample generation process .context35[Sampling _directly_ from a complex, high-dimensional distribution is difficult.] For the Tetris problem, a meaningful decomposition of the samples is .highlight1[adding one piece to the board at a time]. .left-column[.center[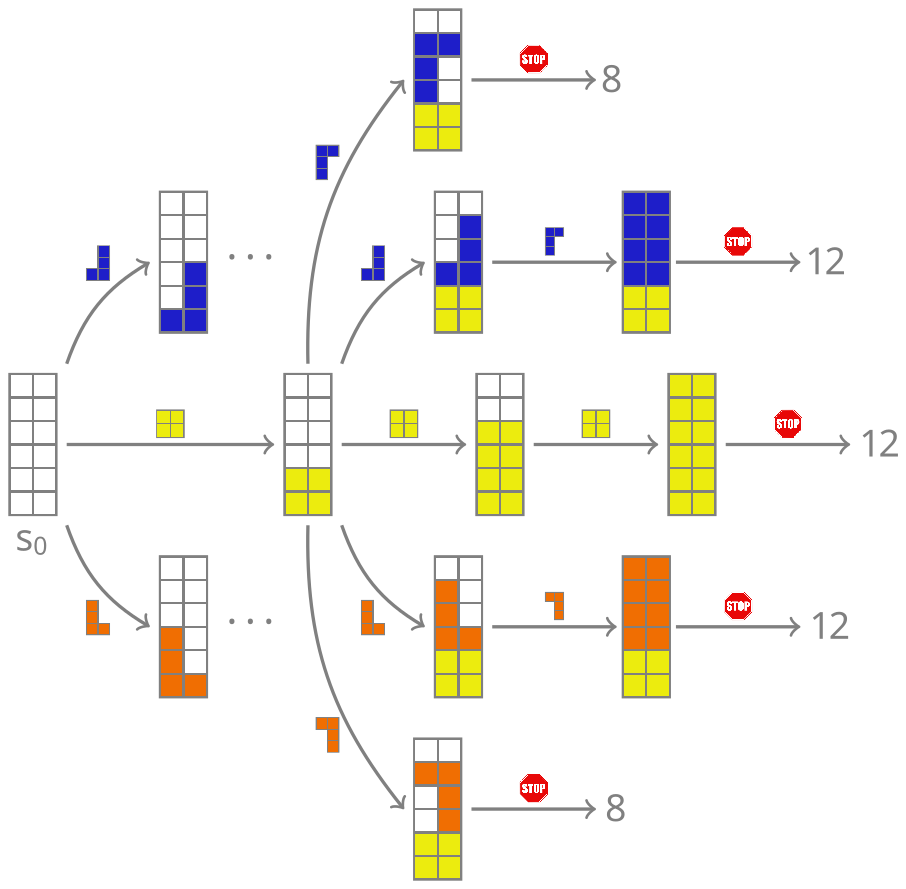]] --- count: false ## 2. Compositionality ### Sample generation process .context35[Sampling _directly_ from a complex, high-dimensional distribution is difficult.] For the Tetris problem, a meaningful decomposition of the samples is .highlight1[adding one piece to the board at a time]. .left-column[.center[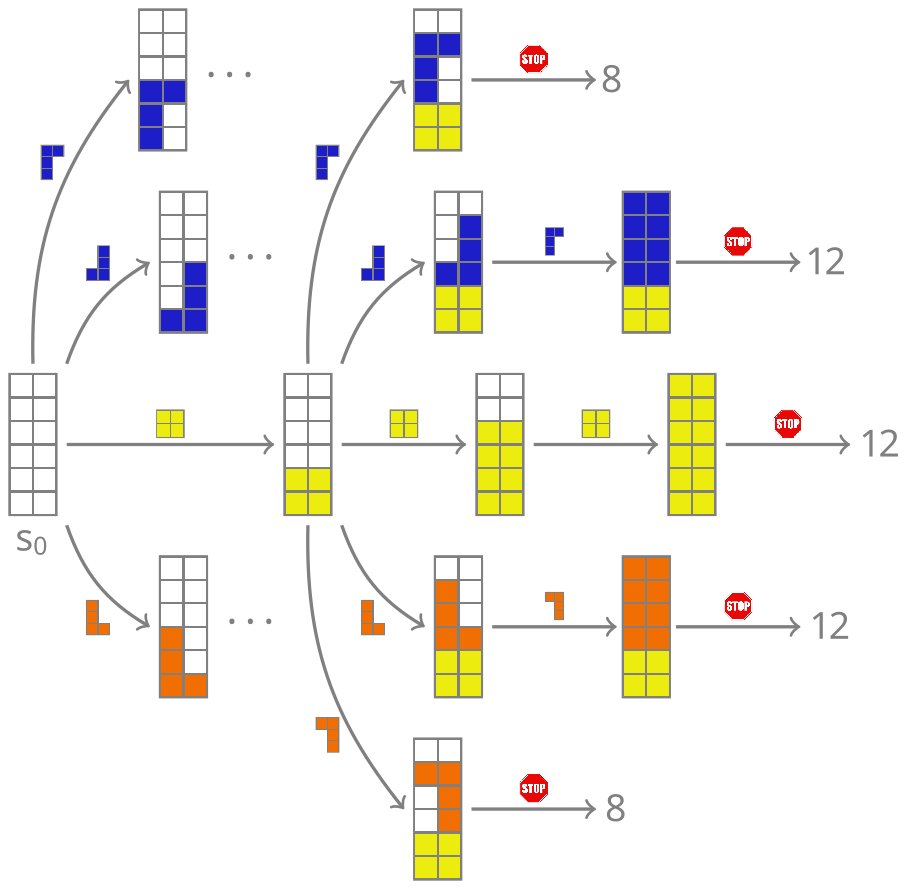]] --- count: false ## 2. Compositionality ### Sample generation process .context35[Sampling _directly_ from a complex, high-dimensional distribution is difficult.] For the Tetris problem, a meaningful decomposition of the samples is .highlight1[adding one piece to the board at a time]. .left-column[.center[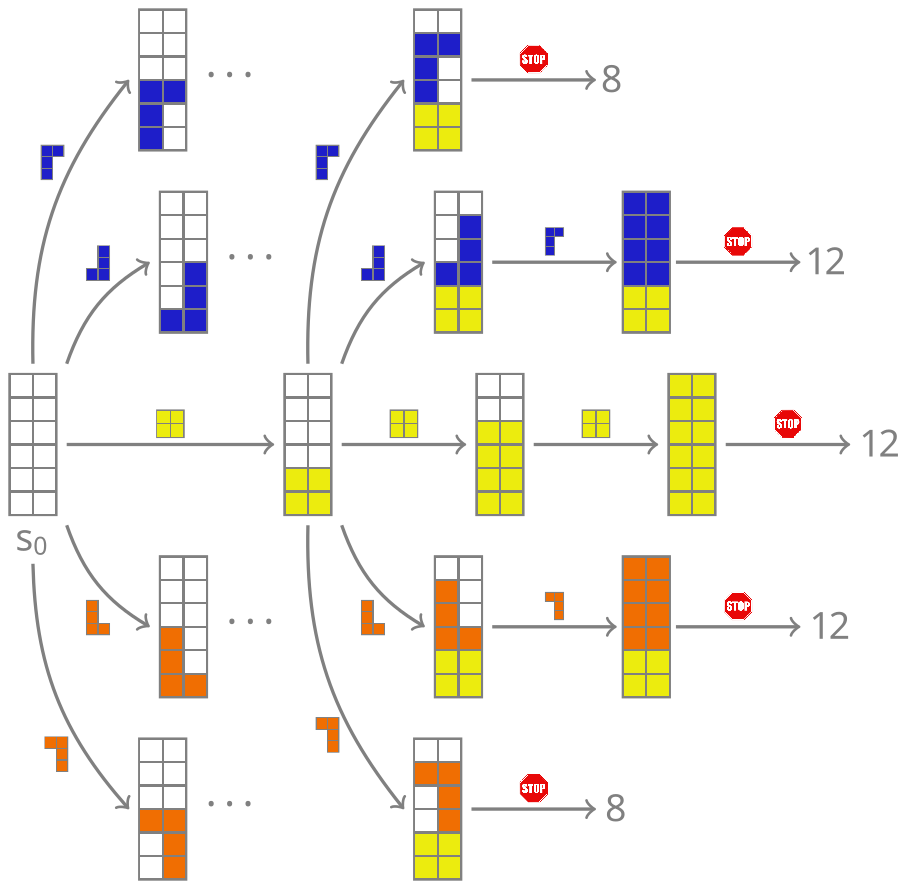]] --- count: false ## 2. Compositionality ### Sample generation process .context35[Sampling _directly_ from a complex, high-dimensional distribution is difficult.] For the Tetris problem, a meaningful decomposition of the samples is .highlight1[adding one piece to the board at a time]. .left-column[.center[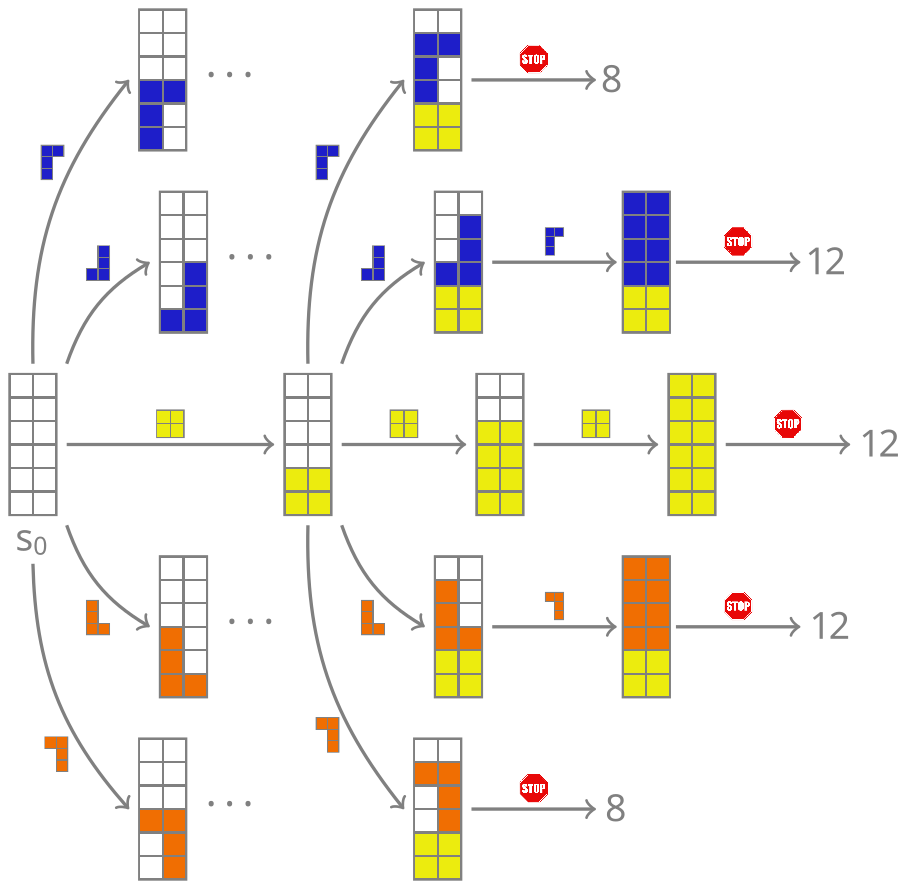]] .right-column[
.conclusion[The decomposition of the sampling process into meaningful steps yields patterns that may be correlated with the reward function and facilitates learning complex distributions.] ] --- count: false ## 3. Deep learning policy .context35[GFlowNets learn a sampling policy $\pi\_{\theta}(x)$ proportional to the reward $R(x)$.] -- .left-column[ .center[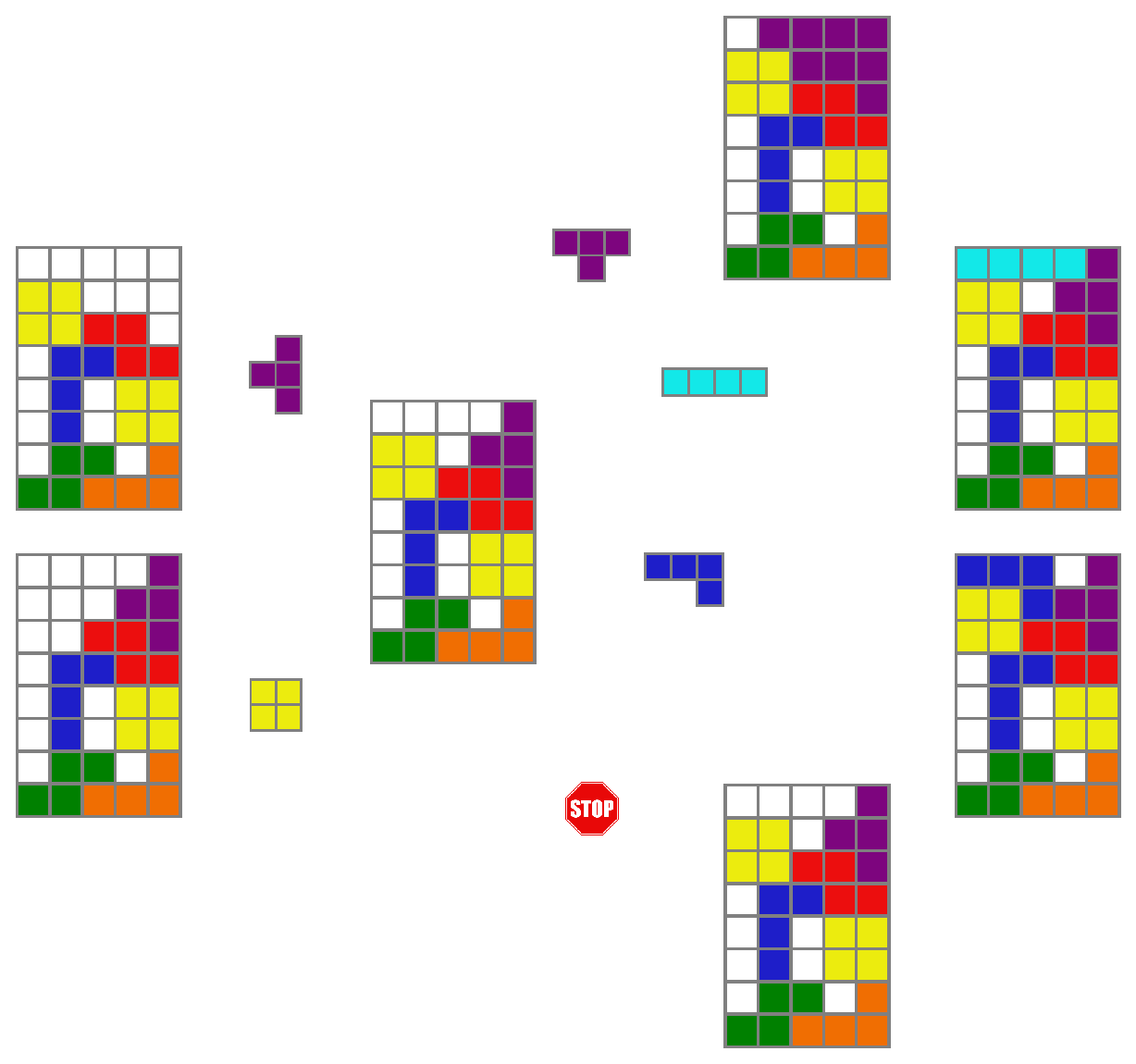] ] --- count: false ## 3. Deep learning policy .context35[GFlowNets learn a sampling policy $\pi\_{\theta}(x)$ proportional to the reward $R(x)$.] .left-column[ .center[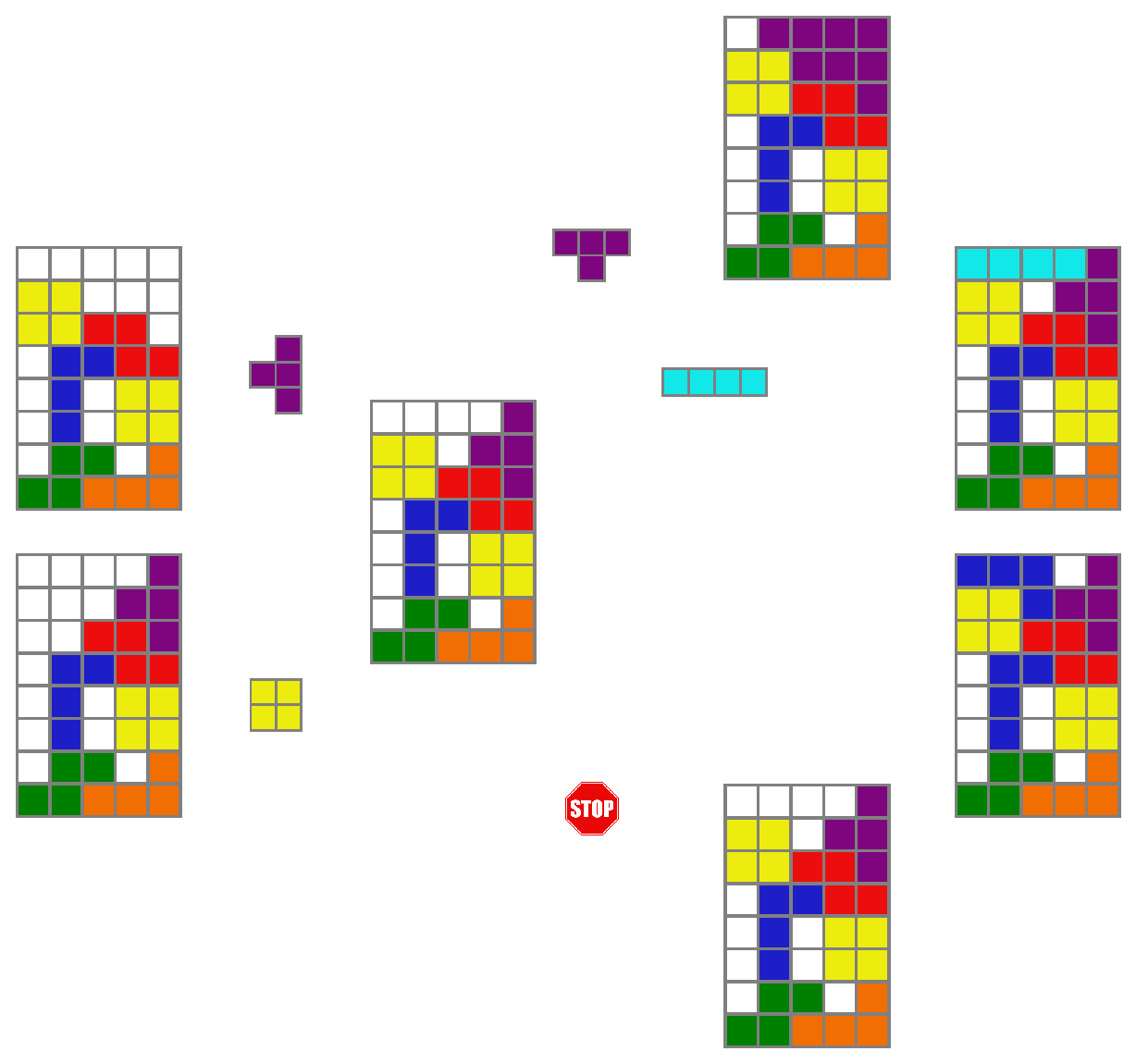] ] .right-column[
Deep neural networks are trained to learn the transitions (flows) policy: $F\_{\theta}(s\_t\rightarrow s\_{t+1})$. ] -- .right-column[ Consistent flow theorem (informal): if the sum of the flows into state $s$ is equal to the sum of the flows out, then $\pi(x) \propto R(x)$. ] .references[ Bengio et al. [Flow network based generative models for non-iterative diverse candidate generation](https://arxiv.org/abs/2106.04399), NeurIPS, 2021. (_not_ co-authored) ] -- .right-column[ .conclusion[GFlowNets can be trained with deep learning methods to learn a sampling policy $\pi\_{\theta}$ proportional to a reward $R(x)$.] ] --- count: false ## GFlowNets review paper A review of the potential of GFlowNets for AI-driven scientific discoveries. .center[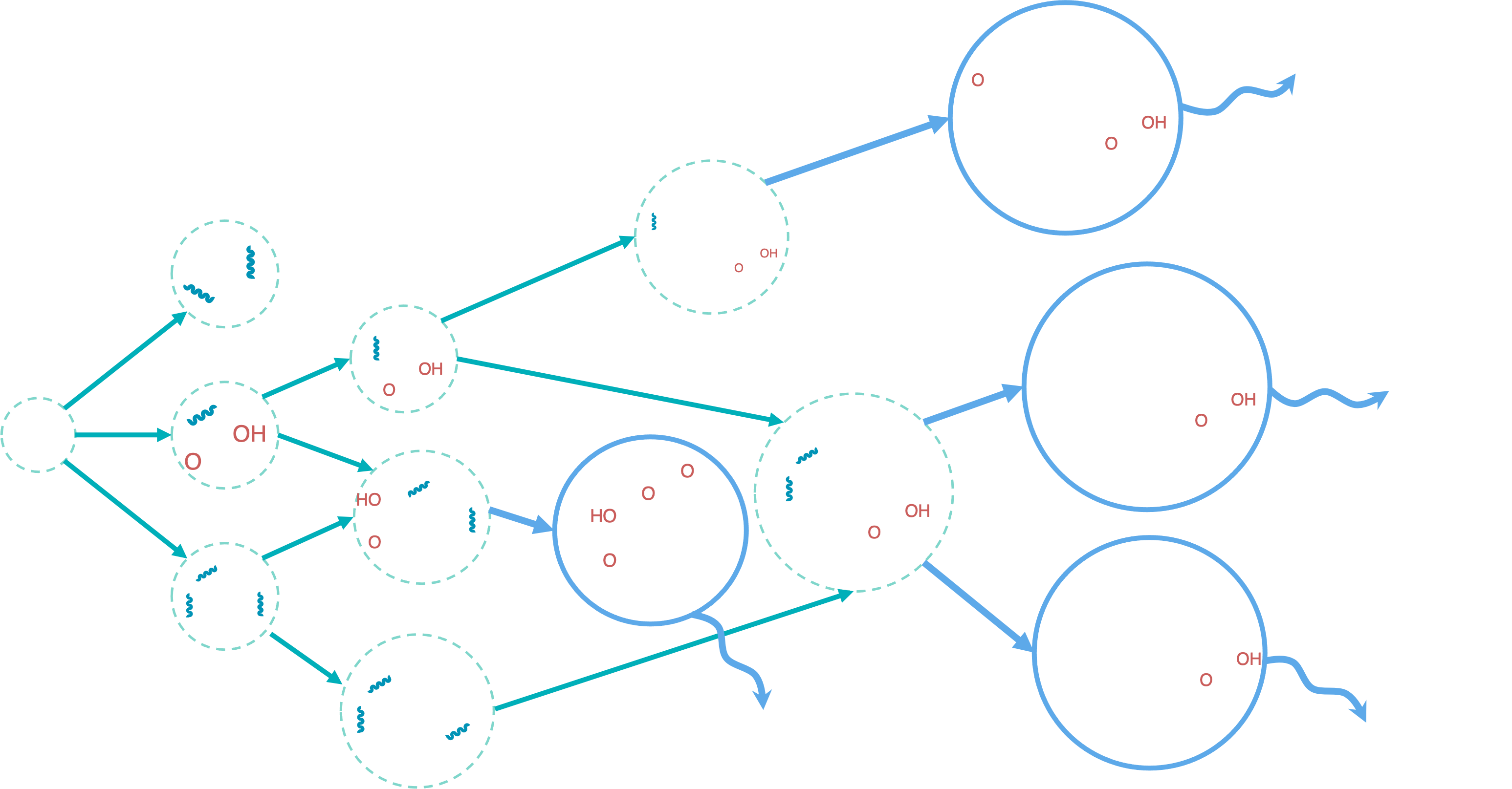] .references[ Jain et al. [GFlowNets for AI-Driven Scientific Discovery](https://pubs.rsc.org/en/content/articlelanding/2023/dd/d3dd00002h). Digital Discovery, Royal Society of Chemistry, 2023. ] --- count: false ## GFlowNet Python package Open sourced GFlowNet package, together with Mila collaborators: Nikita Saxena, Alexandra Volokhova, Michał Koziarski, Divya Sharma, Pierre Luc Carrier, Victor Schmidt, Joseph Viviano. .highlight2[Open source GFlowNet implementation]: [github.com/alexhernandezgarcia/gflownet](https://github.com/alexhernandezgarcia/gflownet) --- count: false name: mfal class: title, middle ## Multi-fidelity active learning Nikita Saxena, Moksh Jain, Cheng-Hao Liu, Yoshua Bengio .smaller[[Multi-fidelity active learning with GFlowNets](https://arxiv.org/abs/2306.11715). Transactions on Machine Learning Research (TMLR). 2024.] .center[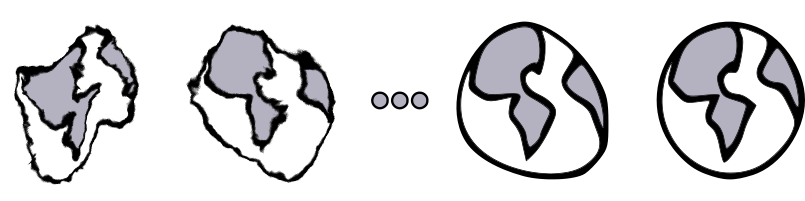] --- count: false ## Why multi-fidelity? .context35[We had described the scientific discovery loop as a cycle with one single oracle.]
.right-column[ .center[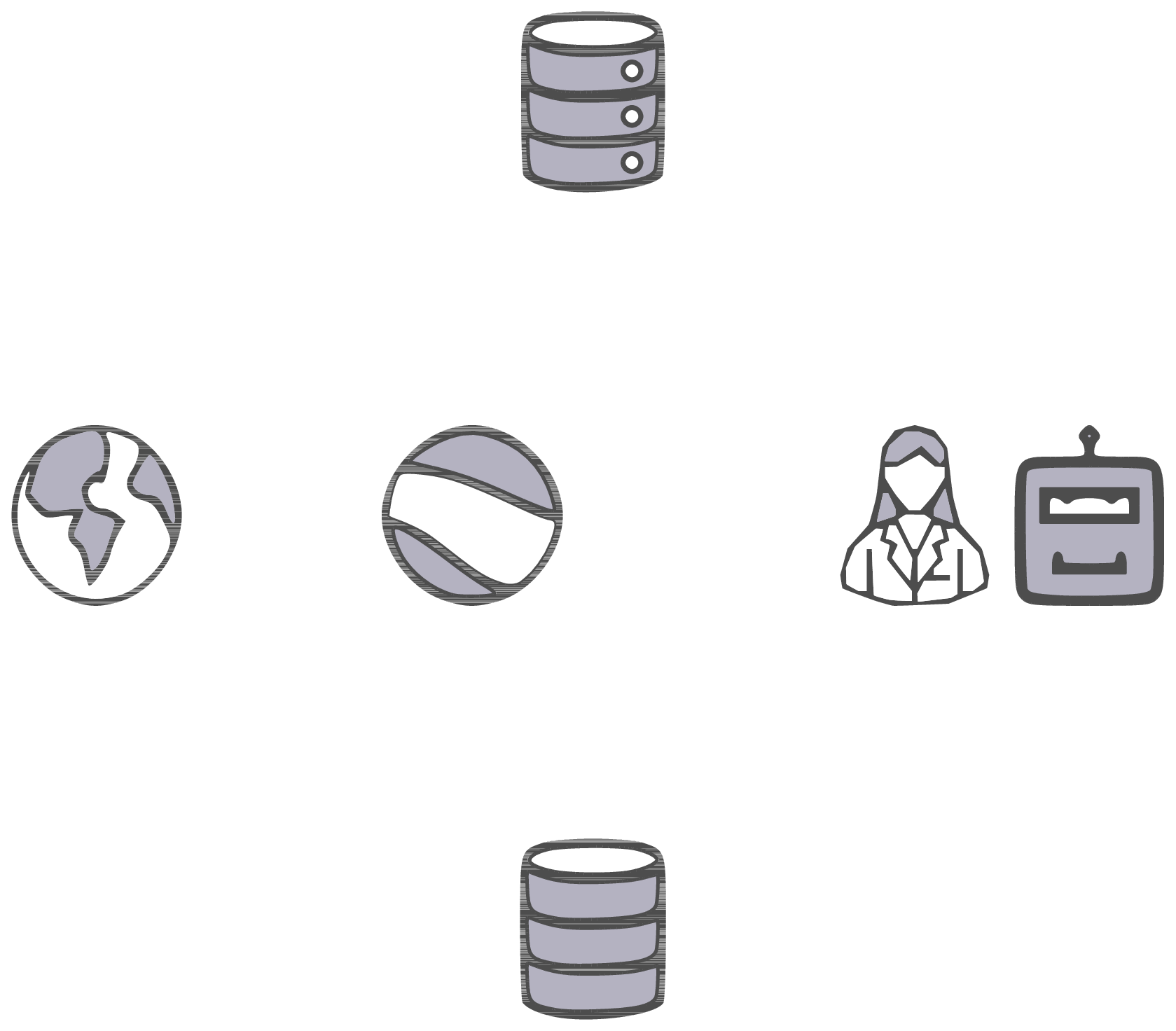] ] -- .left-column[ Example: "incredibly hard" Tetris problem: find arrangements of Tetris pieces that optimise an .highlight2[unknown function $f$]. - $f$: Oracle, cost per evaluation 1000 CAD. .center[
] ] --- count: false ## Why multi-fidelity? .context35[However, in practice, multiple oracles (models) of different fidelity and cost are available in scientific applications.]
.right-column[ .center[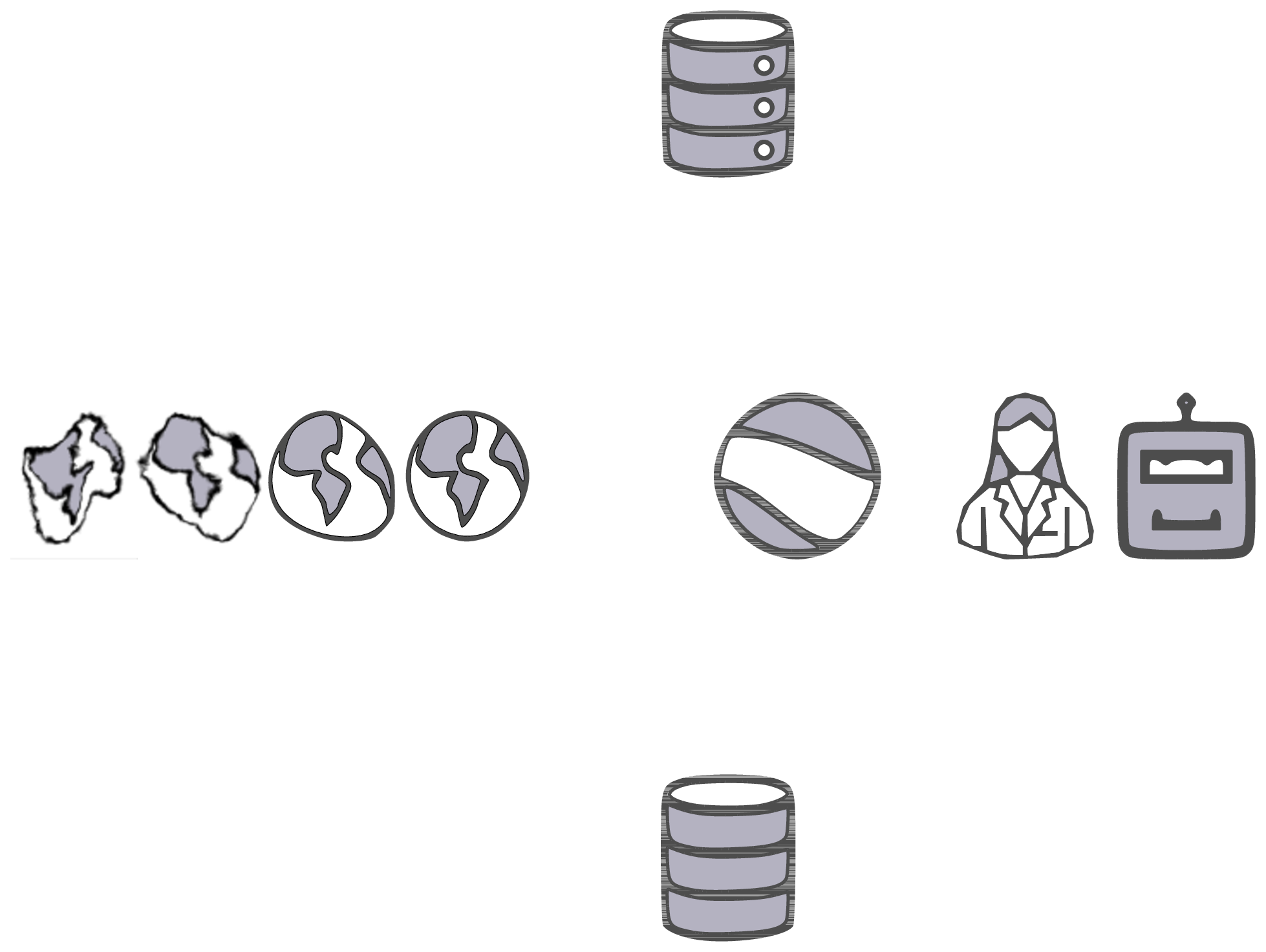] ] .left-column[ Example: "incredibly hard" Tetris problem: find arrangements of Tetris pieces that optimise an .highlight2[unknown function $f$]. - $f$: Oracle, cost per evaluation 1000 CAD. .center[
] ] --- count: false ## Why multi-fidelity? .context35[However, in practice, multiple oracles (models) of different fidelity and cost are available in scientific applications.]
.right-column[ .center[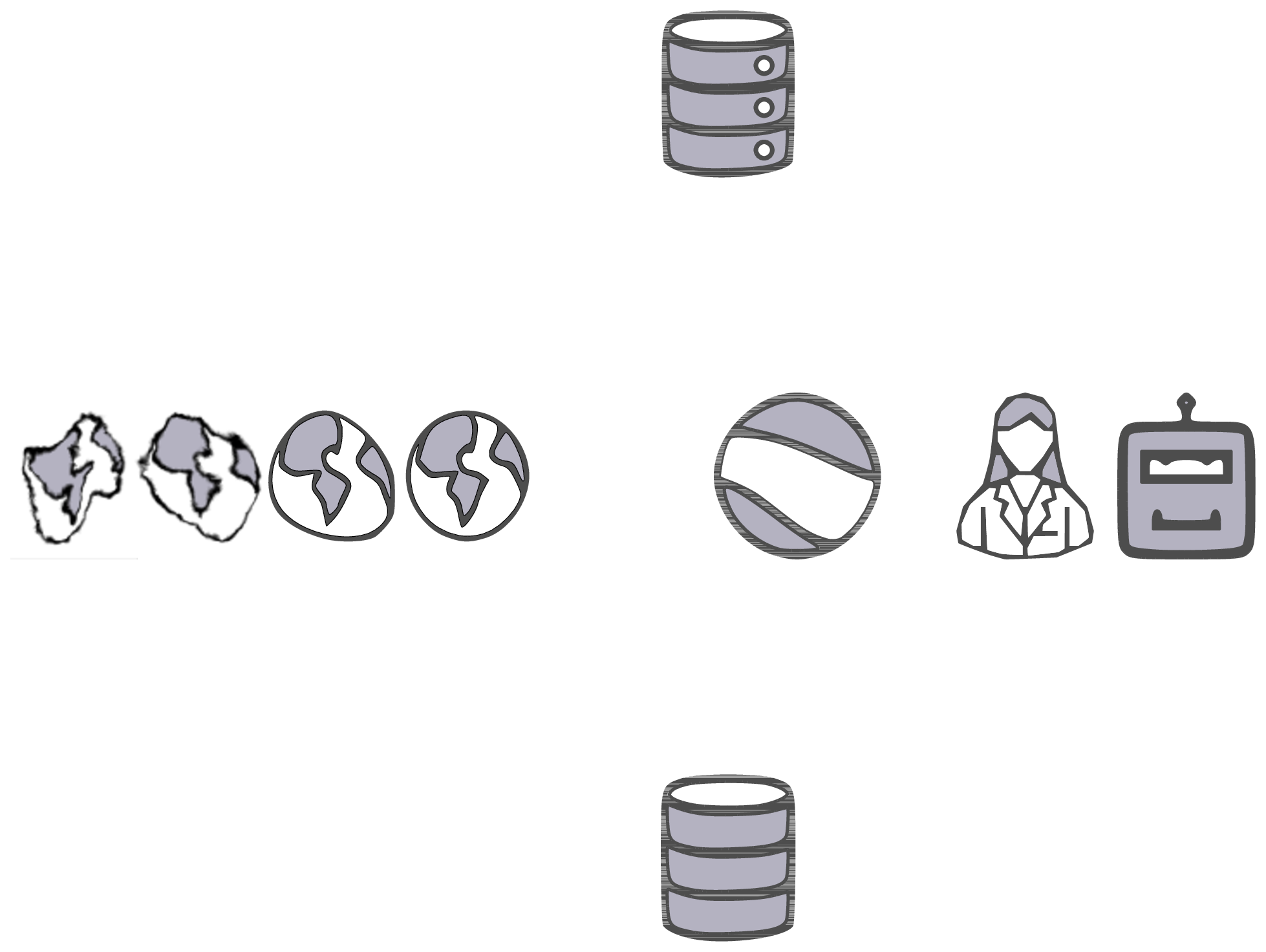] ] .left-column[ Example: "incredibly hard" Tetris problem: find arrangements of Tetris pieces that optimise an .highlight2[unknown function $f$]. - $f$: Oracle, cost per evaluation 1000 CAD. - $f\_1$: Slightly inaccurate oracle, cost 100 CAD. - $f\_2$: Noisy but informative oracle, cost 1 CAD. .center[
] ] --- count: false ## Why multi-fidelity? .context[In many scientific applications we have access to multiple approximations of the objective function.] .left-column[ For example, in .highlight1[material discovery]: * .highlight1[Synthesis] of a material and characterisation of a property in the lab * Molecular dynamic .highlight1[simulations] to estimate the property * .highlight1[Machine learning] models trained to predict the property ] .right-column[ .center[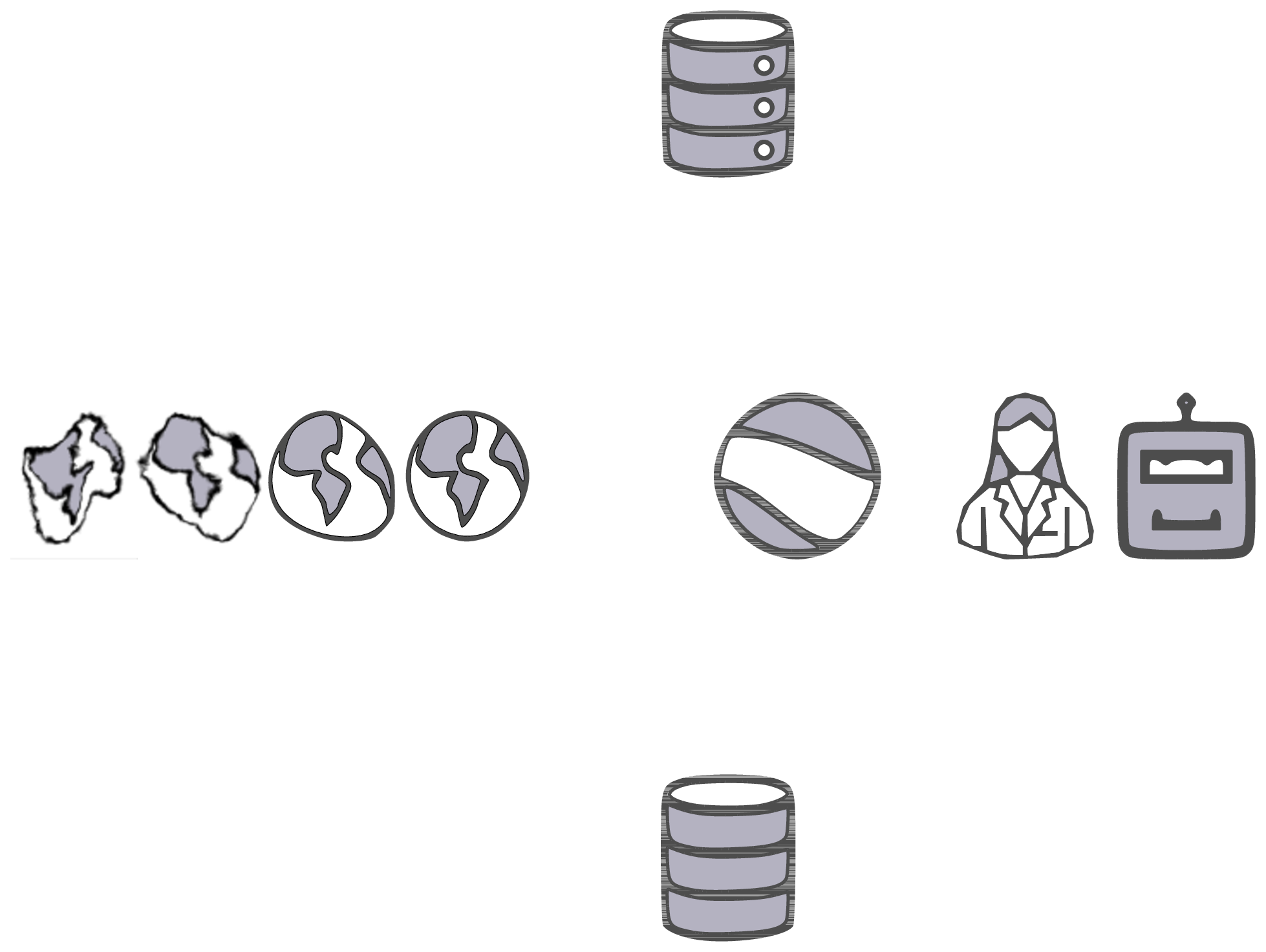] ] -- .conclusion[However, current machine learning methods cannot efficiently leverage the availability of multiple oracles and multi-fidelity data. Especially with .highlight1[structured, large, high-dimensional search spaces].] --- count: false ## Contribution - An .highlight1[active learning] algorithm to leverage the availability of .highlight1[multiple oracles at different fidelities and costs]. -- - The goal is two-fold: 1. Find high-scoring candidates 2. Candidates must be diverse -- - Experimental evaluation with .highlight1[biological sequences and molecules]: - DNA - Antimicrobial peptides - Small molecules - Classical multi-fidelity toy functions (Branin and Hartmann) -- .conclusion[Likely the first multi-fidelity active learning method for biological sequences and molecules.] --- count: false ## Our multi-fidelity active learning algorithm .center[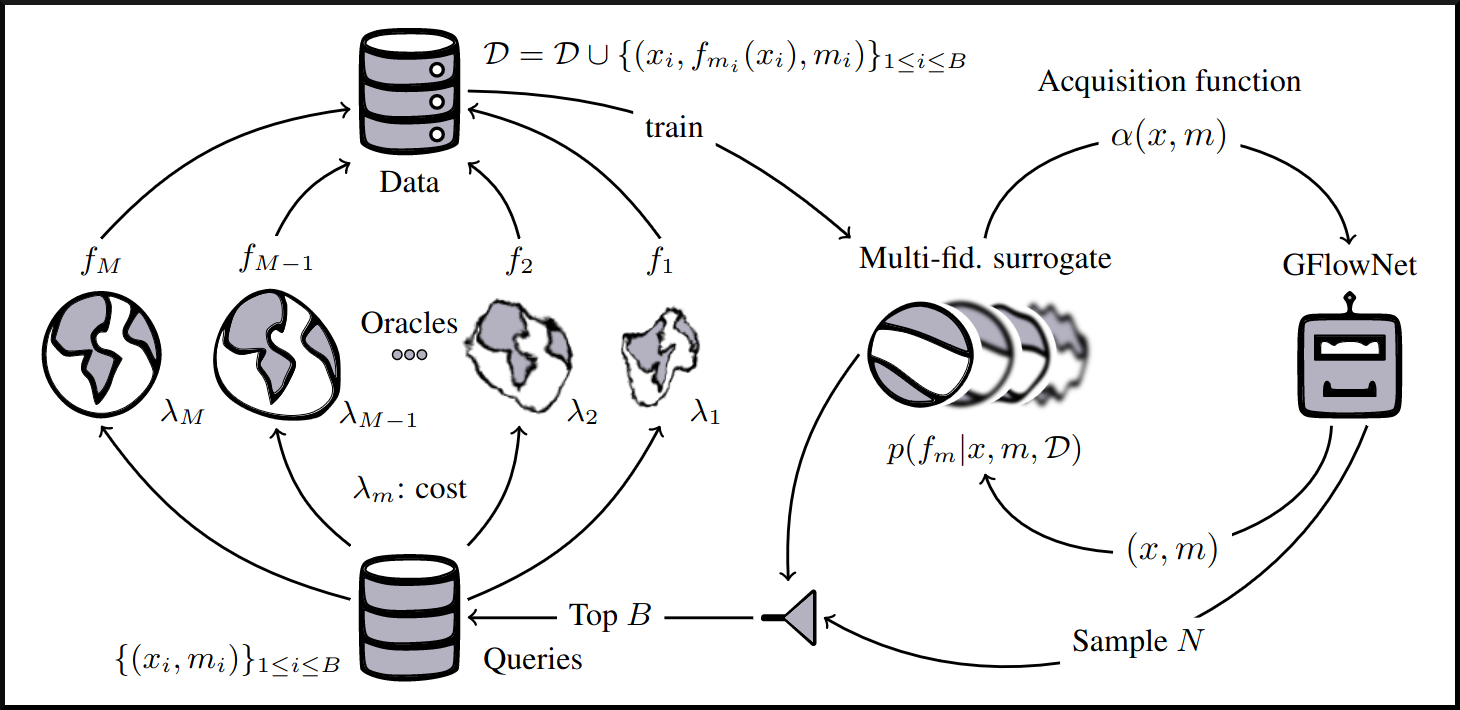] --- count: false ## Applications ### Ongoing, planned and potential * Discovering materials with high ionic conductivity for solid-state electrolyte batteries. * Discovering novel antibiotics through a lab-in-the-loop approach. * Designing electrocatalysts for sustainability purposes. * Designing DNA aptamers and proteins that can bind to specific targets. * `
`